Graph-CNNpred: Advanced Deep Learning for Superior Accuracy in Stock Market Trend Prediction
Yuhui Jin's Graph-CNNpred model integrates convolutional and graph neural networks to predict stock market indices, significantly outperforming traditional models and demonstrating superior trading performance. This innovative approach effectively captures both temporal and relational data, enhancing prediction accuracy and practical trading strategies.
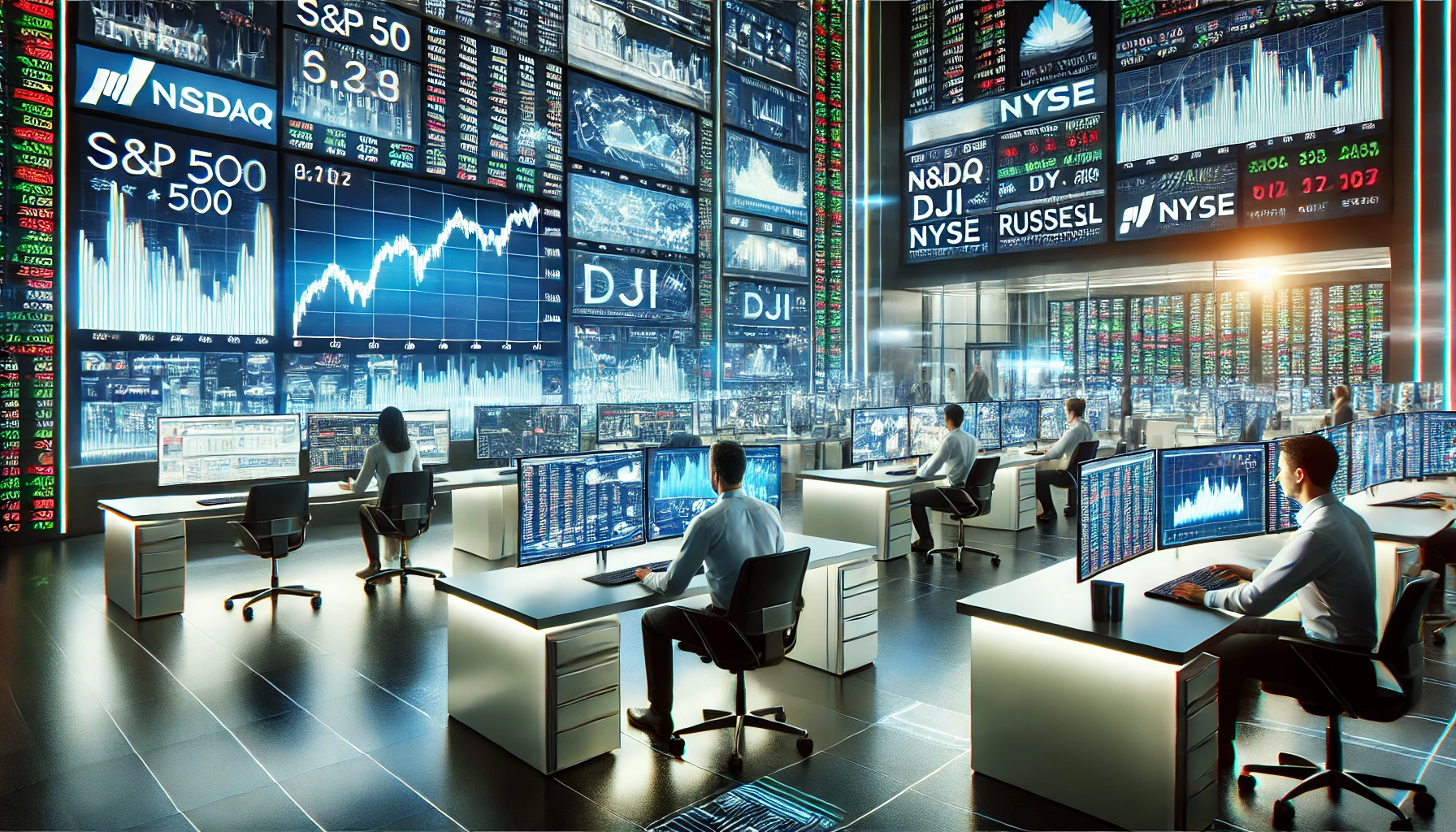
Yuhui Jin from the California Institute of Technology has introduced an advanced deep learning model named Graph-CNNpred, designed to predict trends in stock market indices. This model uniquely integrates convolutional neural networks (CNN) and graph neural networks (GNN) to utilize diverse data sources effectively, aiming to forecast indices such as the S&P 500, NASDAQ, DJI, NYSE, and RUSSELL with high accuracy. The complexity of financial markets, influenced by a myriad of variables including global economic conditions, company financial reports, and news events, poses a significant challenge for predictive modeling. Traditional models often fail to capture the intricate interplay of these factors. However, with the rise of deep learning in the past decade, new methods have emerged to tackle these forecasting problems. Graph-CNNpred distinguishes itself by combining CNNs and GNNs to capture both temporal and relational data patterns.
Leveraging CNNs for Time Series Data
The CNN component, which was originally developed for image processing tasks, has been adapted to handle time series data for stock market predictions. It processes historical data to extract features representing daily market behaviors and trends. This is achieved through a series of convolutional layers that transform raw data into higher-level features, essential for understanding market movements. Concurrently, the GNN part of Graph-CNNpred leverages the interconnected nature of financial data. GNNs are particularly effective in applications requiring the understanding of relationships and dependencies within a dataset, making them ideal for stock market analysis. In this model, GNNs employ message-passing mechanisms to update node features based on information from their neighbors, effectively capturing the complex dependencies among different market indicators.
Optimizing Feature Extraction
Graph-CNNpred is designed to optimize both daily and durational feature extraction. The daily feature extraction phase utilizes CNN layers to consolidate daily market variables into significant higher-level features. Following this, the durational feature extraction phase analyzes these features over time to identify patterns and trends. The final stage involves a fully connected layer that synthesizes these extracted features to predict future market trends. The dataset used for this study spans from January 2010 to November 2017 and includes 82 features categorized into primitive features, technical indicators, economic data, global stock indices, exchange rates, commodities, major US company data, and future contracts. The model undergoes a rigorous training-validation-testing split to ensure robust evaluation and validation.
Superior Performance and Trading Success
Comparative analysis shows that Graph-CNNpred significantly outperforms baseline models, including PCA-ANN, technical indicators, and CNN-cor, across multiple indices. For example, the GAT-CNNpred variant achieves notable improvements in F-measure, underscoring its superior predictive capabilities. Furthermore, the GAT-CNNpred model demonstrates impressive trading performance, validated through simulations that consider Sharpe ratios and certainty-equivalent returns. The success of Graph-CNNpred is attributed to its dual capability to respect the time series nature of financial data while efficiently processing complex relational features. This dual approach not only enhances prediction accuracy but also ensures robust and smooth learning processes, making it a valuable tool for financial market analysis.
Innovative Data Representation Techniques
In practice, the CNN part of Graph-CNNpred processes input data from different markets to predict future market fluctuations. The goal is to develop a general model that can predict the future of a market based on its historical data, applicable to various markets. This involves designing a model that learns from samples across different markets to extract the desired mapping function from historical data to future trends. Each day in the historical data is represented by variables like opening and closing prices, which are combined in the first CNN layer to create higher-level features representing daily market activities. Over time, these features are analyzed to identify trends and patterns, which are then used for final predictions through a fully connected layer.
Breaking New Ground in Financial Forecasting
The GNN component, on the other hand, constructs a graph where each feature is a node, and edges are formed based on feature correlations. This structure allows the model to gather information from correlated features, enhancing the prediction process. By integrating GNNs with CNN layers, Graph-CNNpred effectively captures both temporal and relational aspects of the data, leading to more accurate predictions. The performance of Graph-CNNpred is further validated through trading simulations, where it demonstrates superior results in terms of Sharpe ratios and certainty-equivalent returns compared to other models. This indicates that Graph-CNNpred not only provides accurate predictions but also translates these predictions into effective trading strategies. Overall, Graph-CNNpred represents a significant advancement in stock market prediction models, combining the strengths of CNN and GNN techniques to offer a powerful framework for financial market analysis and trading strategy formulation. This study opens new avenues for integrating deep learning approaches in financial market analysis, with potential applications extending to real-world trading systems.
- FIRST PUBLISHED IN:
- Devdiscourse
ALSO READ
Markets Steady After Economic Data Surprises: S&P 500 and Nasdaq on the Rise
FSB Pursues CNN Journalist for Alleged Illegal Border Crossing
FSB Targets CNN Journalist for Illegal Border Crossing
FSB Opens Criminal Case Against CNN Journalist Nick Paton Walsh
CNN Journalist Faces Criminal Charges for Alleged Russian Border Crossing