AI that emulates human-like expansion and reflection in writing
Traditionally, machine writing frameworks rely heavily on retrieval-augmented generation (RAG) to produce content by sourcing information from vast databases. While effective in providing information, these systems often fail to deliver content that is both deeply insightful and contextually relevant. The results are frequently fragmented, redundant, or lack the coherence expected in high-quality writing.
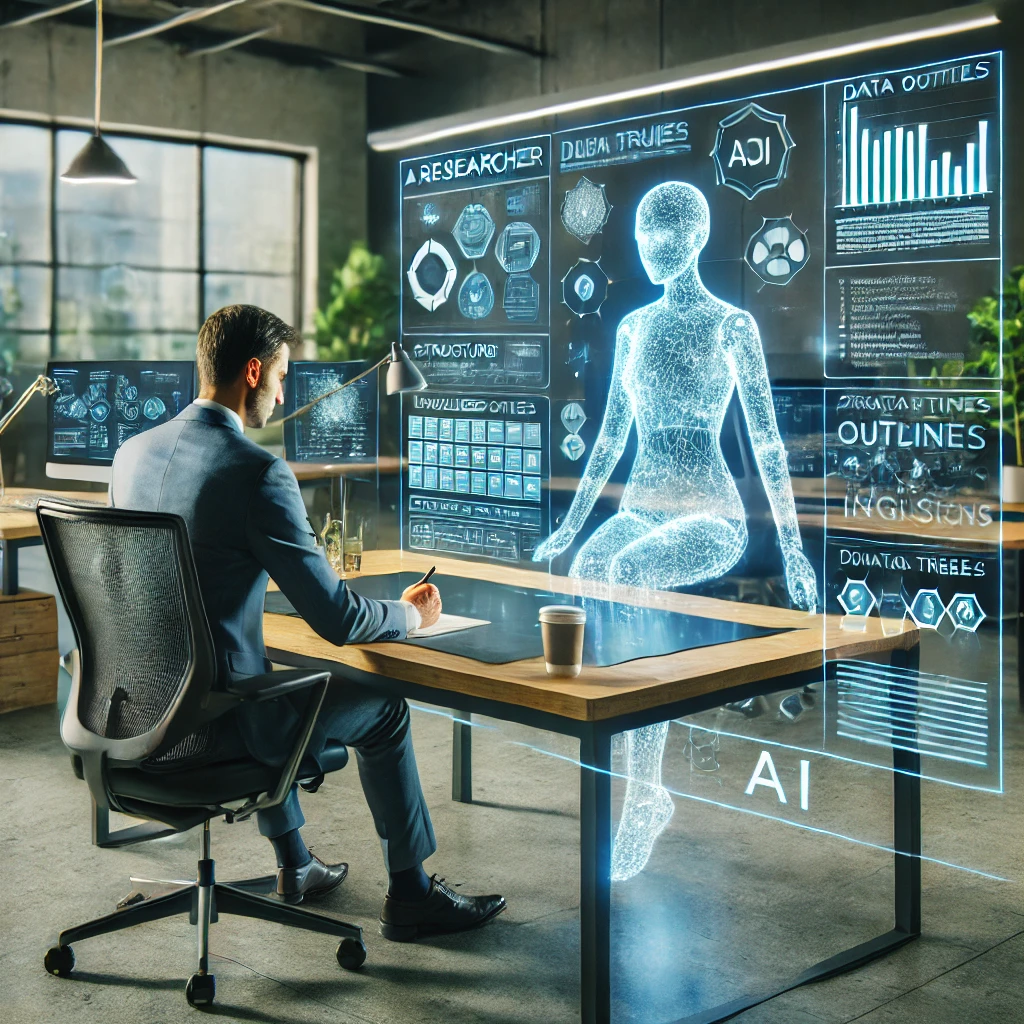
Artificial intelligence (AI) continues to push the boundaries of human ingenuity, with machine writing emerging as one of its most transformative capabilities. However, despite significant advancements in large language models (LLMs), the ability to emulate nuanced human cognition remains a formidable challenge.
Addressing this gap, a pioneering study titled “OmniThink: Expanding Knowledge Boundaries in Machine Writing through Thinking” introduces a novel framework that combines expansion and reflection to revolutionize AI-driven content creation. Authored by Zekun Xi, Wenbiao Yin, Jizhan Fang, Jialong Wu, Runnan Fang, Ningyu Zhang, Jiang Yong, Pengjun Xie, Fei Huang, and Huajun Chen, this research, a collaboration between Zhejiang University and Tongyi Lab (Alibaba Group), is submitted on arXiv. OmniThink represents a new era in machine writing, mimicking human cognitive processes to generate content that is both insightful and highly structured.
Cognitive challenge in machine writing
Traditionally, machine writing frameworks rely heavily on retrieval-augmented generation (RAG) to produce content by sourcing information from vast databases. While effective in providing information, these systems often fail to deliver content that is both deeply insightful and contextually relevant. The results are frequently fragmented, redundant, or lack the coherence expected in high-quality writing.
OmniThink redefines this paradigm by simulating the human process of iterative thinking, where information is not only gathered but critically analyzed, connected, and refined to create comprehensive and meaningful narratives. This approach bridges the gap between machine efficiency and human-level cognitive depth, marking a significant leap forward in AI-driven content creation.
At the heart of OmniThink lies a robust three-phase framework designed to replicate the iterative and reflective nature of human cognition. The first phase, Information Acquisition, constructs a hierarchical “information tree” by dynamically expanding and organizing relevant subtopics. Unlike conventional methods that retrieve data in isolation, OmniThink’s approach ensures a comprehensive exploration of the topic, capturing diverse perspectives and related concepts.
The second phase, Outline Structuring, synthesizes insights derived from the information tree to create a logically coherent framework for the content. This stage mirrors how humans organize thoughts into a structured narrative before beginning the writing process. Finally, the Article Composition phase integrates these elements into high-density, contextually enriched content. By dynamically accessing the conceptual pool generated in earlier phases, OmniThink produces outputs that are not only relevant but also imbued with originality and depth.
What sets OmniThink apart is its ability to combine Expansion and Reflection seamlessly. The expansion module dynamically gathers relevant information, enriching the breadth of content, while the reflection module critically analyzes and refines the gathered data. This iterative process eliminates redundancy, fosters novel insights, and ensures a higher level of coherence, akin to how humans refine their understanding through cycles of learning and introspection.
Raising the bar for machine writing
One of OmniThink’s most significant contributions to the field of AI is the introduction of Knowledge Density (KD) as a metric to evaluate content quality. Knowledge density measures the ratio of meaningful content to overall text volume, addressing a long-standing challenge in machine writing where verbose outputs often mask a lack of depth. In rigorous evaluations, OmniThink achieved a KD score of 0.66, surpassing baseline methods like RAG and STORM. This metric, combined with human evaluations, underscores the system’s ability to generate content that is not only precise but also innovative and comprehensive.
OmniThink also excelled in measures of relevance and novelty. Human evaluators consistently rated its outputs as superior in terms of integrating diverse information into cohesive narratives, highlighting the framework’s ability to bridge complexity with clarity. The framework’s performance on diverse datasets demonstrated its adaptability across varied domains, further solidifying its position as a game-changer in machine writing.
Applications across diverse domains
OmniThink’s ability to mimic human-like thought processes opens doors to transformative applications across industries. In education, the framework can be employed to create high-quality learning materials that are tailored to specific curricula, ensuring depth and contextual relevance. In academic research, OmniThink offers a powerful tool for drafting papers and synthesizing findings, significantly reducing the time and effort required for literature reviews and manuscript preparation. Businesses stand to benefit as well, with OmniThink enhancing marketing and communication by generating persuasive and precise content that aligns with brand objectives.
What truly sets OmniThink apart in these applications is its adaptability. By creating outputs that are both generalizable and domain-specific, the framework caters to diverse user needs while maintaining the consistency and accuracy required in professional environments.
Challenges and future directions
While OmniThink represents a significant advancement, the study acknowledges certain limitations. The framework’s current reliance on text-based inputs restricts its potential to integrate multimodal data such as images, videos, or audio, which are increasingly important in fields like multimedia content creation and scientific research. Additionally, OmniThink’s outputs, while highly structured and insightful, lack the ability to adopt personalized language styles tailored to individual users or audiences.
The researchers propose several future directions to address these limitations. Enhancing OmniThink with multimodal capabilities could revolutionize its application across visual storytelling, video scriptwriting, and scientific visualization. Similarly, incorporating personalized style options would make OmniThink even more versatile, enabling users to tailor outputs to specific contexts or audiences. These advancements would further solidify OmniThink’s role as a comprehensive tool for next-generation content creation.
- FIRST PUBLISHED IN:
- Devdiscourse