Real-time gesture recognition improves smart home accessibility for elderly and disabled users
Gesture recognition stands out as a revolutionary tool in making technology more inclusive. Unlike traditional interfaces like keyboards, touchscreens, or voice commands, gesture-based systems rely on natural movements, such as waving a hand or pointing, to control devices. This eliminates barriers posed by physical exertion or complex instructions for individuals with disabilities or mobility challenges.
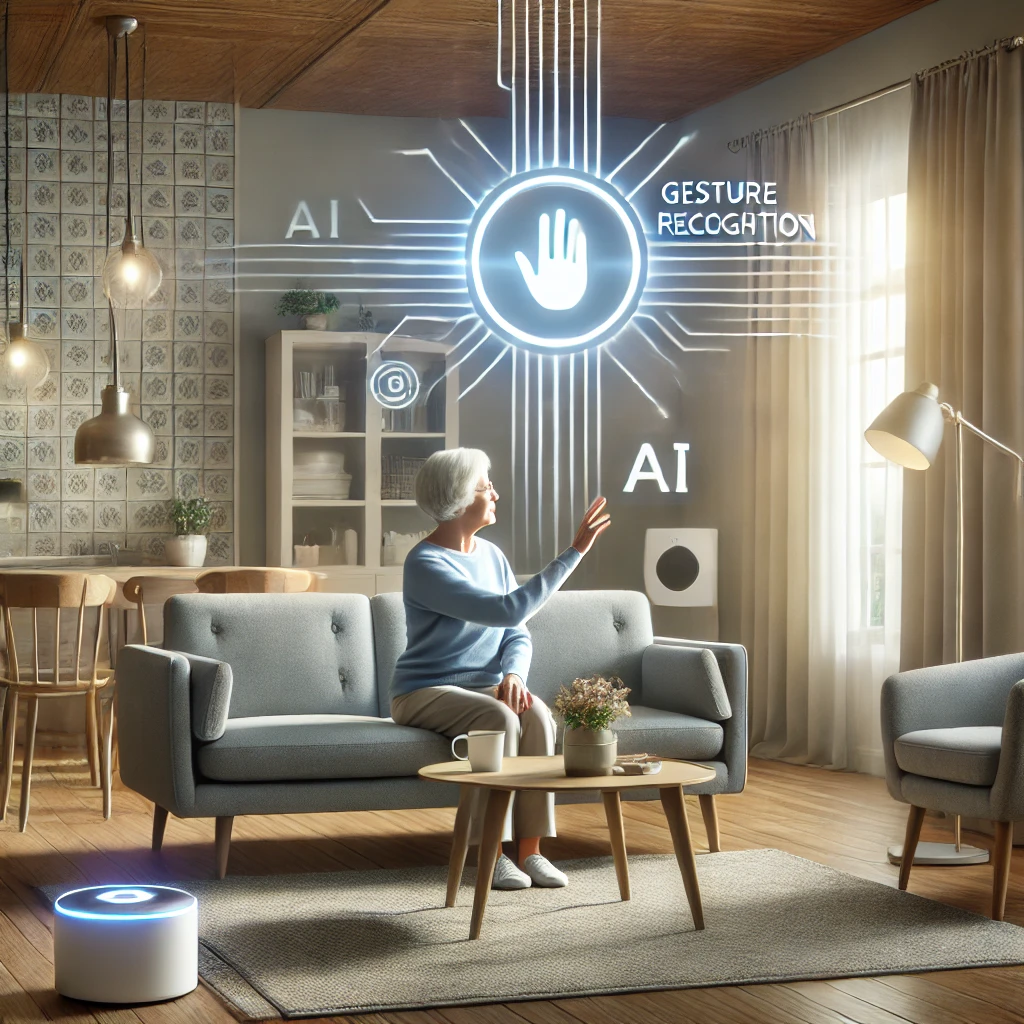
For people with disabilities, technology offers not just convenience but a gateway to independence and empowerment. Among the most promising advancements is gesture recognition - a technology that allows users to interact with devices seamlessly, without physical strain or complicated controls. In smart home environments, this innovation has the power to redefine accessibility, creating a future where technology serves everyone, regardless of physical or cognitive limitations.
In their groundbreaking study titled “Multidisciplinary ML Techniques on Gesture Recognition for People with Disabilities in a Smart Home Environment,” authors Christos Panagiotou, Evanthia Faliagka, Christos P. Antonopoulos, and Nikolaos Voros present a transformative vision of how AI-powered systems can enhance human-computer interaction (HCI) for individuals with disabilities. Published in AI 2025, 6, 17, the research combines advanced machine learning with practical application, delivering solutions that empower users while addressing real-world challenges of usability, adaptability, and scalability.
Role of gesture recognition in accessibility
Gesture recognition stands out as a revolutionary tool in making technology more inclusive. Unlike traditional interfaces like keyboards, touchscreens, or voice commands, gesture-based systems rely on natural movements, such as waving a hand or pointing, to control devices. This eliminates barriers posed by physical exertion or complex instructions for individuals with disabilities or mobility challenges.
In a smart home environment, gesture recognition enables critical functionalities like controlling lights, adjusting locks, locating objects, and activating alarms. These capabilities not only make everyday tasks manageable but also enhance safety, independence, and overall quality of life for the elderly and disabled. Importantly, this technology bridges the gap between innovation and accessibility, ensuring that advancements in AI serve all members of society equitably.
A user-centric approach to research
The study begins with a needs analysis involving 30 participants aged 60 and above, shedding light on the specific challenges faced by elderly individuals in interacting with smart home systems. Tasks such as turning on lights, locking doors, or locating objects emerged as critical pain points that gesture recognition could address.
By grounding the research in real-world needs, the authors ensured that their solutions were both practical and impactful. To explore these challenges, the researchers evaluated three distinct approaches to gesture recognition: wearable inertial measurement unit (IMU) systems, lightweight machine learning models running on edge devices, and vision-based solutions combining MoveNet and convolutional neural networks (CNNs). Each approach was rigorously tested under realistic conditions to ensure usability, reliability, and adaptability in daily life scenarios.
Innovations in gesture recognition technology
The integration of MoveNet and convolutional neural networks forms the cornerstone of this research. MoveNet detects key body points, such as hand positions and joint movements, translating physical actions into digital signals, while CNNs classify these signals into actionable gestures, enabling commands like "turn on the lights" or "lock the door" to be executed seamlessly. By processing only keypoint data instead of raw images, this hybrid approach reduces computational complexity while improving real-time performance. The system also demonstrates remarkable robustness against environmental factors such as lighting variations and occlusions, ensuring reliable performance in diverse settings. This combination of efficiency, accuracy, and adaptability positions the MoveNet-CNN framework as a leading solution for gesture recognition in smart homes.
The study’s results highlight the effectiveness of gesture recognition in enhancing accessibility. For the "Lights On" gesture, the system achieved a 96.5% accuracy rate, demonstrating reliability even in challenging conditions. For the "Activate Locks" gesture, the framework delivered its highest performance, with 97.0% accuracy and an F1 score of 96.9%, reflecting both precision and consistency.
Comparative analysis revealed important trade-offs among the methods tested. Wearable IMU systems offered high precision but were less practical due to user discomfort and dependence on external devices. Vision-based methods, on the other hand, proved more adaptable and convenient for everyday use, making them ideal for integration into smart homes.
Challenges and opportunities
Despite its success, the study acknowledges the inherent challenges of developing gesture recognition systems for diverse users. Variability in physical capabilities, cultural differences in gestures, and environmental inconsistencies can hinder performance. For example, gestures that work well in a well-lit room may fail under dim lighting or obstructions caused by furniture.
The researchers propose several strategies to address these challenges, including the development of adaptive algorithms that learn and adjust to individual user preferences and abilities, the integration of multimodal systems combining visual data with additional inputs like audio cues or wearable sensors, and the expansion of training datasets to include diverse demographics and gesture variations. These advancements will ensure that gesture recognition systems remain inclusive and effective across diverse populations and environments.
Future implications for smart home accessibility
The study’s findings hold transformative implications for the design of inclusive smart home technologies. By enabling natural, intuitive interactions, gesture recognition systems eliminate barriers to accessibility, empowering elderly and disabled individuals to live independently and safely. The integration of gesture recognition with other AI-driven technologies such as voice assistants, home automation, and IoT devices has the potential to create a truly interconnected and intelligent living environment. This vision aligns with broader societal goals of equity and inclusivity, ensuring that technological advancements benefit all segments of the population.
- FIRST PUBLISHED IN:
- Devdiscourse