Healthcare reimagined: AI systems that integrate seamlessly, protecting what matters
The study outlines innovative approaches to model validation that go beyond internal testing. By leveraging external validation - testing models on entirely separate datasets - researchers demonstrate how robust CDSS can generalize across different patient populations and settings. This focus on generalizability addresses one of the most critical hurdles in AI healthcare applications.
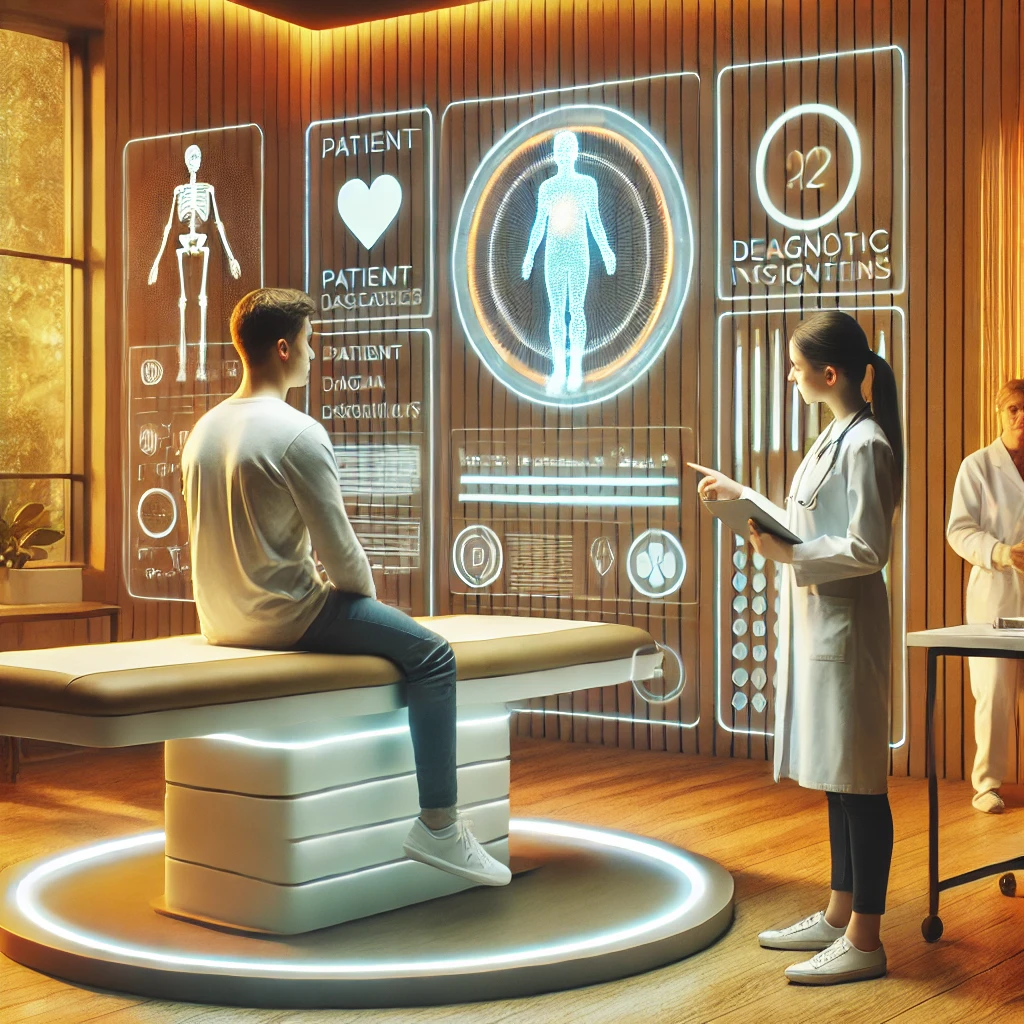
The healthcare sector is undergoing a revolutionary transformation, driven by advancements in artificial intelligence (AI). From diagnosing diseases to personalizing treatments, AI has the potential to enhance patient outcomes, optimize clinical workflows, and empower healthcare professionals with precise, data-driven insights. However, the integration of AI into healthcare systems, particularly Clinical Decision Support Systems (CDSS), presents unique challenges. Ensuring fairness, privacy, and explainability remains critical to building systems that clinicians can trust and patients can rely on.
In this context, the study titled “Artificial Intelligence-Driven Clinical Decision Support Systems,” authored by Muhammet Alkan, Idris Zakariyya, Samuel Leighton, Kaushik Bhargav Sivangi, Christos Anagnostopoulos, and Fani Deligianni from the University of Glasgow, offers a groundbreaking exploration of how AI can reshape clinical decision-making. Submitted on the arXiv preprint repository, the research delves into the intricacies of creating robust, ethical, and reliable CDSS, charting a path forward for AI in healthcare.
Key insights
Bridging gaps in model development and validation
The study outlines innovative approaches to model validation that go beyond internal testing. By leveraging external validation - testing models on entirely separate datasets - researchers demonstrate how robust CDSS can generalize across different patient populations and settings. This focus on generalizability addresses one of the most critical hurdles in AI healthcare applications.
Calibration as a cornerstone
Effective CDSS must align predicted risks with observed outcomes. Calibration curves, which plot predicted probabilities against actual outcomes, serve as vital tools in assessing and refining models. The research emphasizes that well-calibrated models are essential for preventing over- or underestimation of risks, a critical factor in clinical decision-making.
Explainability: The human connection
Explainability is a central theme of the study, addressing the need for clinicians to understand AI-driven recommendations. The authors argue that trust in AI systems hinges on their transparency. By integrating explainability methods - such as Shapley Additive Explanations (SHAP) and class activation maps - the study provides a roadmap for building AI systems that offer interpretable and actionable insights.
Addressing fairness and bias
A standout aspect of this research is its emphasis on addressing fairness and bias, a critical concern in the development of AI models for Clinical Decision Support Systems (CDSS). Clinical data, which forms the backbone of AI training, often carries historical biases rooted in socio-economic, racial, and gender disparities. If left unchecked, these biases can lead to discriminatory outcomes, exacerbating existing inequalities in healthcare delivery. The authors underscore the need to recognize and mitigate biases at every stage of the model development process, from the initial selection of datasets to the final design and deployment of algorithms.
One of the key strategies discussed is the use of stratified k-fold cross-validation, which ensures that datasets are divided into balanced subsets representing diverse patient groups. This technique minimizes the risk of overfitting to dominant groups in the data and improves the model’s generalizability across varied populations. Additionally, the study highlights the importance of subgroup calibration, where models are fine-tuned to perform equitably across different demographic subgroups, ensuring that predictions are not skewed in favor of or against any particular group.
The research also advocates for proactive identification of implicit biases through exploratory data analysis and fairness audits, enabling developers to detect patterns of discrimination early in the development cycle. By incorporating these methodologies, the study paves the way for creating AI systems that align with the principles of equity and inclusivity, ensuring that advancements in healthcare technology benefit all individuals, regardless of their background.
Privacy in AI-driven healthcare
The integration of AI into healthcare systems raises pressing privacy concerns. Deep learning models, while powerful, are susceptible to data leakage and adversarial attacks. The research explores privacy-preserving techniques such as differential privacy and federated learning, which allow models to train on decentralized data without compromising patient confidentiality.
Differential privacy
This technique involves adding noise to data or model parameters, ensuring that individual patient information remains secure while enabling accurate predictions. By implementing differential privacy, healthcare systems can balance the trade-off between data utility and protection.
Federated learning
Federated learning offers a decentralized approach to model training, where data remains on local devices, and only aggregated insights are shared. This method addresses privacy concerns while enabling collaborative advancements across institutions.
Future directions and implications
The researchers highlight that the future of Clinical Decision Support Systems (CDSS) depends on their ability to integrate seamlessly into clinical workflows, ensuring their utility in real-world healthcare settings. Achieving this vision requires more than just technical advancements; it demands active collaboration among AI developers, clinicians, and policymakers to align technological capabilities with practical needs and ethical standards.
A key priority is the design of ethical AI frameworks, which must prioritize patient well-being, ensure transparency, and comply with stringent regulatory standards to foster trust and accountability. Equally important is the focus on scalable deployment, ensuring that CDSS can function effectively in diverse healthcare environments, ranging from advanced urban hospitals to resource-constrained rural clinics, thereby expanding the reach of quality care.
Furthermore, the study underscores the need for interdisciplinary collaboration, bridging the gap between technical innovation and clinical expertise. By incorporating insights from healthcare practitioners and domain experts, AI systems can be designed to address real-world challenges while being intuitive and user-friendly. Together, these efforts pave the way for AI-driven CDSS to not only enhance decision-making but also transform healthcare delivery on a global scale.
- FIRST PUBLISHED IN:
- Devdiscourse