Socially responsible AI: Building an inclusive future for all
AI has the potential to transform society for the better, solving complex global challenges and enhancing efficiency in various sectors. However, the authors highlight a critical paradox: while AI can drive positive change, its reliance on historical data often amplifies existing inequalities. For example, biased hiring algorithms, discriminatory loan approval systems, and gendered language models demonstrate how uncritical deployment of AI can perpetuate systemic injustice.
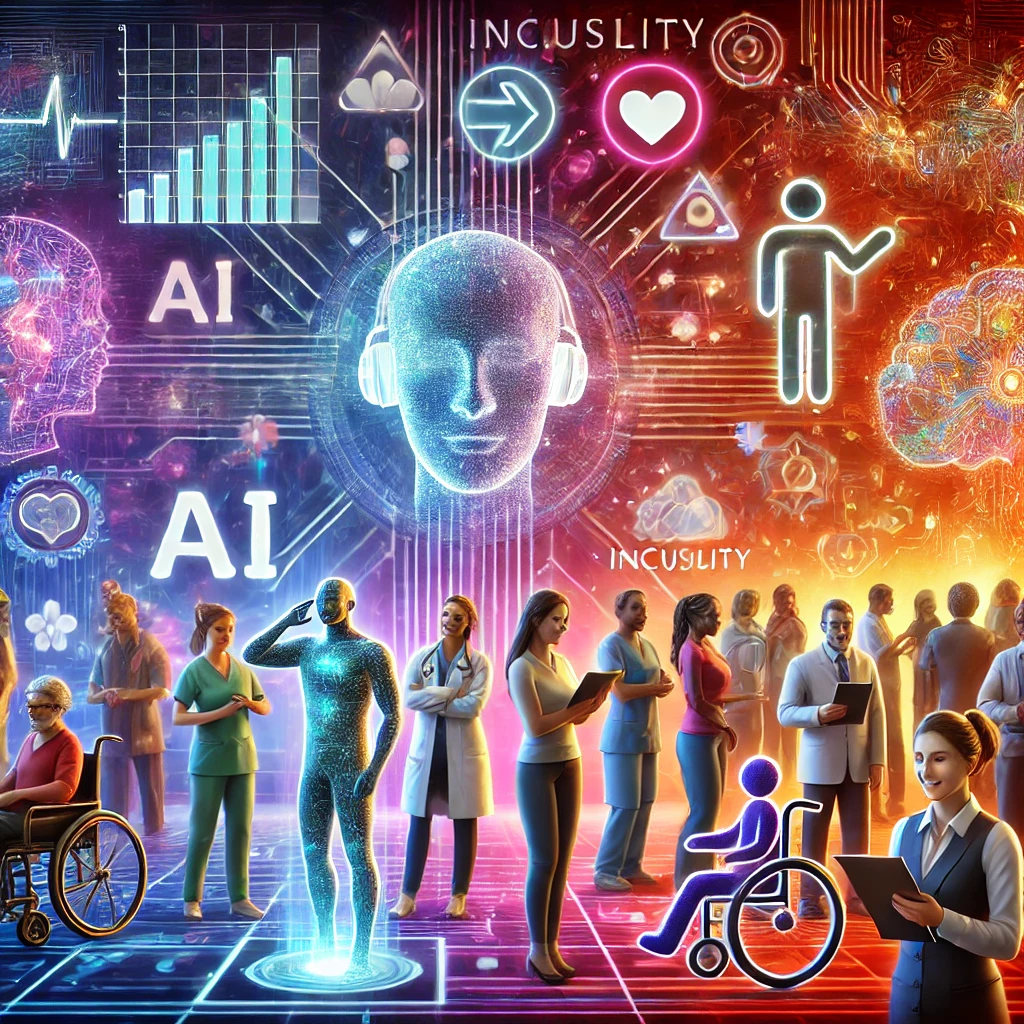
The rapid adoption of artificial intelligence (AI) raises significant concerns about bias, inequality, and accessibility. In their groundbreaking study titled "AI in Support of Diversity and Inclusion," authors Çiçek Güven, Afra Alishahi, Henry Brighton, Gonzalo Nápoles, Juan Sebastian Olier, Eric Postma, Marie Šafář, Dimitar Shterionov, Mirella De Sisto, and Eva Vanmassenhove explore how AI can be harnessed to foster diversity and inclusion, while addressing systemic biases inherent in machine learning systems. Submitted on arXiv by researchers at Tilburg University’s Department of Cognitive Science and Artificial Intelligence (CSAI), this multidisciplinary work presents a comprehensive approach to building equitable AI systems.
The paper delves into real-world applications, discusses inherent challenges in AI development, and offers actionable solutions to create technologies that empower marginalized communities. By embedding fairness and inclusivity into the core of AI systems, the authors argue, we can address societal inequalities and promote a more just future.
Promise and perils of AI in society
AI has the potential to transform society for the better, solving complex global challenges and enhancing efficiency in various sectors. However, the authors highlight a critical paradox: while AI can drive positive change, its reliance on historical data often amplifies existing inequalities. For example, biased hiring algorithms, discriminatory loan approval systems, and gendered language models demonstrate how uncritical deployment of AI can perpetuate systemic injustice.
The study emphasizes the duality of AI as both a tool for empowerment and a potential source of harm. The authors call for a multidisciplinary approach that combines computational science, ethics, sociology, and linguistics to address these risks. This approach requires a fundamental shift in how AI systems are designed, deployed, and monitored.
One of the foundational issues addressed in the study is the lack of transparency in AI systems. Large language models (LLMs) and other deep learning systems are often treated as "black boxes," producing outputs without clear explanations of how decisions are made. This opacity becomes particularly concerning when AI systems are deployed in sensitive areas such as law enforcement, education, and healthcare.
Co-author Afra Alishahi emphasizes that enhancing transparency is essential for building trust in AI systems. To this end, the study suggests developing models that not only provide accurate results but also explain their reasoning processes. This includes techniques to make decision-making pathways visible to users and stakeholders, enabling more informed oversight. Transparent AI systems are better equipped to navigate social dynamics, cultural nuances, and ethical considerations, thereby increasing their reliability and accountability.
Identifying and mitigating bias in AI
The persistence of gender, racial, and cultural biases in AI-driven systems remains a significant challenge. Eva Vanmassenhove, a key contributor to the study, highlights how natural language processing (NLP) tools often reinforce stereotypes. For instance, gender-neutral sentences are frequently translated into languages like English with gendered assumptions, such as defaulting to "he" for professions like doctor or scientist. This bias not only reflects societal inequalities but also risks perpetuating them through the widespread use of AI-driven technologies.
To address these issues, Gonzalo Nápoles focuses on creating advanced algorithms to detect and reduce bias in datasets. Using techniques like fuzzy-rough sets and recurrent neural networks, these algorithms can identify both explicit and implicit biases. Impressively, the study reports that these methods achieve bias mitigation rates of up to 76% while preserving critical data integrity. Such tools are vital for ensuring that AI systems align with the principles of equity and fairness.
Another significant area of concern is bias in visual media. Juan Sebastian Olier’s work in the study demonstrates how AI can uncover patterns of misrepresentation in images and videos, such as the underrepresentation of certain demographics or the reinforcement of stereotypes. These insights can inform more equitable content creation, reshaping how communities are represented in media.
Applications of inclusive AI
The study explores how AI can address global health challenges, particularly in underserved communities. For instance, the Child Growth Monitor project uses AI to detect malnutrition in children by analyzing mobile images. By integrating data from underrepresented groups, this tool ensures more accurate diagnoses and interventions, directly addressing health disparities that disproportionately affect marginalized populations.
Another innovative application is the SignON Project, which leverages AI to bridge communication gaps between hearing and deaf communities. This project employs sign-to-speech translation tools, co-created with input from the deaf community to ensure inclusivity and usability. By addressing a long-standing communication barrier, the SignON Project exemplifies how AI can empower marginalized groups and foster social inclusion.
Diversity in datasets: A critical need
A recurring theme in the study is the importance of diversifying training datasets. The authors emphasize that the effectiveness of AI systems heavily depends on the representativeness of the data they are trained on. When datasets predominantly reflect privileged groups, the resulting AI systems often fail to serve the needs of underrepresented communities.
The researchers propose creating datasets that encompass a wide range of demographics, cultures, and experiences. By doing so, AI systems can provide fairer and more accurate outputs. This approach not only improves the functionality of AI technologies but also ensures that their benefits are accessible to all.
Toward a fairer AI ecosystem
The study proposes actionable strategies to advance diversity and inclusion in AI. Interdisciplinary collaboration emerges as a cornerstone, calling for the integration of computational science with fields such as ethics, sociology, and law to create comprehensive and socially attuned solutions. Equally vital is community involvement, which emphasizes the active participation of marginalized groups in the co-creation of AI technologies, ensuring these systems address real-world needs and foster trust.
The research also underscores the importance of regulatory oversight, advocating for robust policies that promote transparency, accountability, and ethical compliance in AI development. In addition, educational reform is highlighted as a key priority, focusing on equipping future AI professionals with the skills to identify and mitigate biases, fostering a generation of socially responsible developers. These strategies are not merely theoretical, they represent essential steps toward building an AI ecosystem that aligns with global aspirations for equity, inclusivity, and justice.
- FIRST PUBLISHED IN:
- Devdiscourse