New model sheds light on protecting privacy amid rapid advances in AI
Enhanced models that incorporate additional parameters, such as temporal changes in datasets or adversarial behavior, can improve predictions. Combining insights from privacy, AI ethics, and behavioral sciences can address broader societal concerns.
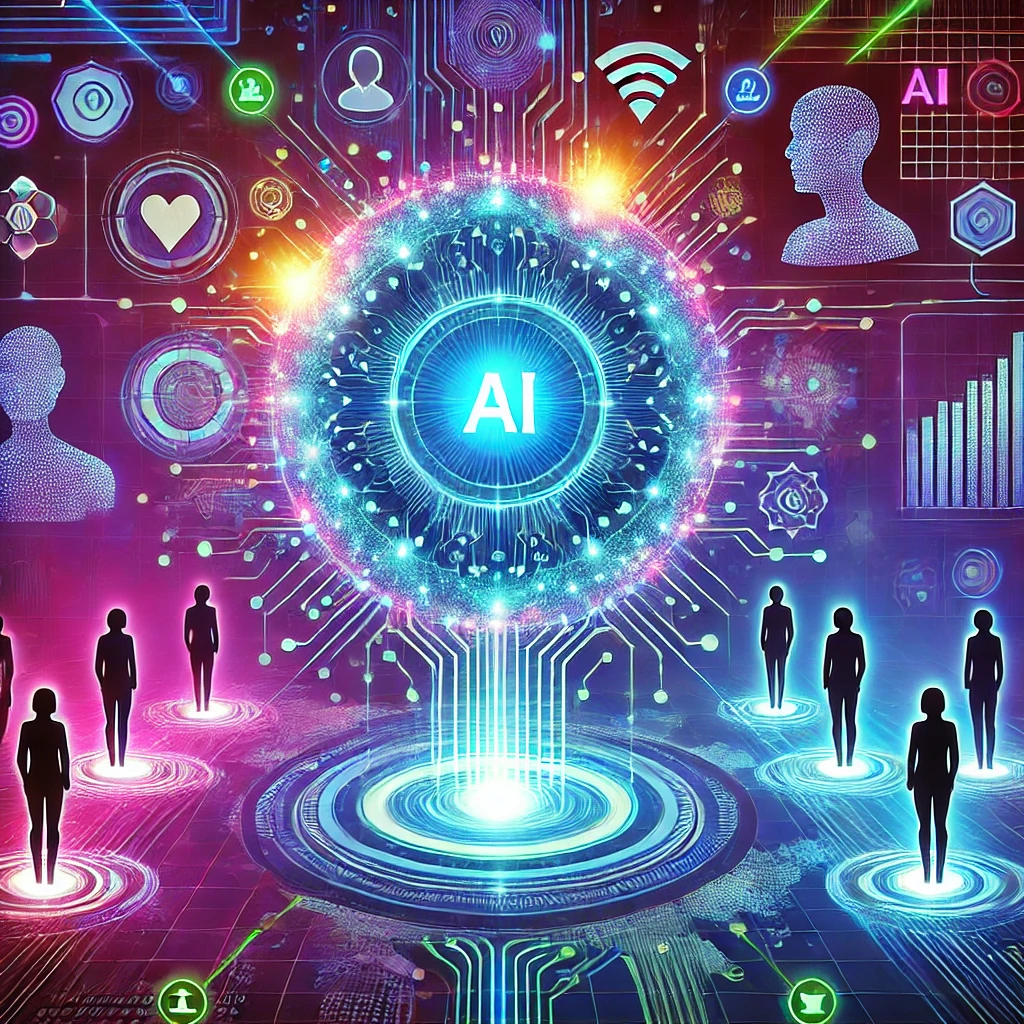
In an increasingly interconnected digital world, the ability to identify individuals from their data has grown exponentially. AI-driven identification techniques have transformed domains such as biometrics, behavioral analysis, and cybersecurity. However, this power brings a dual-edged sword: while identification advances can enhance security and personalization, they also pose significant threats to privacy.
Addressing this, Luc Rocher, Julien M. Hendrickx, and Yves-Alexandre de Montjoye present a seminal study, "A Scaling Law to Model the Effectiveness of Identification Techniques", published in Nat Commun 16, 347 (2025). Their work introduces a Bayesian framework to forecast how the effectiveness of identification techniques scales with population size. This model not only provides theoretical insights but also serves as a practical tool for policymakers, researchers, and technologists to assess privacy risks at scale.
This article delves into the study’s methodology, findings, and implications, offering an in-depth exploration of how this innovative model can shape the future of identification and privacy.
Importance of anonymity
Anonymity is a foundational element of personal freedom, enabling open expression, protection against surveillance, and the preservation of digital rights. Traditionally, anonymity was considered inherent in large populations, where individual identification required significant effort and resources. However, technological advancements have drastically eroded this assumption. Identification techniques now utilize sparse and robust datasets, enabling precise matches even with limited information. This trend is exemplified by the infamous re-identification of the Governor of Massachusetts’s medical records using only a ZIP code, gender, and date of birth—an event that highlighted the fragility of anonymization.
As identification techniques evolve, their implications extend far beyond academic curiosity. Governments, corporations, and malicious actors can exploit these techniques for purposes ranging from targeted advertising to political surveillance. Understanding the scalability and effectiveness of such methods is essential for balancing innovation with ethical considerations.
A Bayesian model for identification
The cornerstone of the study is a two-parameter Bayesian model designed to predict the effectiveness of identification techniques, termed "correctness" (κ). Correctness represents the fraction of individuals accurately identified within a population. The model’s simplicity lies in its reliance on two parameters: entropy (“h”), which measures the average uncertainty or information content in a dataset, and tail complexity (“γ”), which represents the distribution of auxiliary information in the dataset. Higher entropy suggests more variability and complexity in the data, making identification more challenging, while high γ values indicate a heavy-tailed distribution, where a few records contain disproportionately significant identifying information.
The model’s analytical expression for correctness incorporates these parameters to predict identification accuracy across varying population sizes. By validating the model on 476 correctness curves from both real-world and synthetic datasets, the researchers demonstrated its robustness and versatility. It outperformed traditional curve-fitting methods and entropy-based heuristics, achieving a low root mean square error (RMSE) of 1.7 percentage points.
The study examined three categories of identification techniques, namely exact, sparse, and robust matching. Exact matching refers to traditional methods using specific demographic identifiers, where the model accurately forecasted performance across population sizes. Sparse matching, on the other hand, leverages set-valued data, such as shopping histories or geolocation points, demonstrating the model’s adaptability to such complex data. Finally, robust matching employs advanced methods powered by machine learning to handle noisy or approximate data. For example, facial recognition systems maintained high correctness even with large datasets, demonstrating their scalability.
The scaling law provides an unprecedented ability to extrapolate results from small-scale experiments to large populations. For instance, facial recognition techniques could achieve 62% correctness for a global population of 7.53 billion individuals using a single photograph. Similarly, browser fingerprinting - analyzing technical configurations - could correctly identify 75% of 4 billion internet devices. The study also revealed that techniques exhibit varied scaling behaviors depending on their reliance on entropy and tail complexity. Behavioral techniques, like authorship attribution, suffer rapid degradation in correctness with larger populations due to low tail complexity, whereas techniques with higher entropy and tail complexity, such as facial recognition, degrade more gradually.
Practical implications
The study’s findings are far-reaching, offering tools and insights that can influence several domains. In the realm of privacy and data protection, regulators and policymakers can use the scaling law to quantify the risks associated with data releases. By simulating how identification accuracy changes with population size, organizations can ensure compliance with privacy laws such as the GDPR and mitigate re-identification risks.
High-stakes applications, such as facial recognition at borders or hospitals, require robust risk assessments, and this model enables stakeholders to evaluate whether identification systems are suitable for specific contexts and populations. Developers can incorporate the model to forecast unintended consequences of their systems, preventing the deployment of overly invasive technologies in sensitive settings. Furthermore, by predicting correctness, the model aids in designing more effective anonymization strategies, ensuring that datasets retain utility without compromising privacy.
Challenges, opportunities and the road ahead
While the model offers profound capabilities, challenges remain. Real-world data is often noisy, incomplete, or dynamic, complicating predictions. Incorporating temporal and spatial variability remains a challenge. High correctness rates may inadvertently reinforce biases, disproportionately impacting certain demographics. Additionally, advanced adversaries may exploit auxiliary information beyond what the model assumes, necessitating continuous refinement.
The study opens exciting avenues for future research and applications. Enhanced models that incorporate additional parameters, such as temporal changes in datasets or adversarial behavior, can improve predictions. Combining insights from privacy, AI ethics, and behavioral sciences can address broader societal concerns. Moreover, the model can guide the development of synthetic data generators and differential privacy algorithms to balance utility and security. The scaling law can also be extended to novel identification scenarios, ensuring its relevance in an evolving technological landscape.
As we embrace the transformative potential of AI, balancing innovation with ethical considerations will be paramount. This scaling law is not just a tool for analysis but a step toward ensuring that privacy and individuality remain safeguarded in the digital age.
- FIRST PUBLISHED IN:
- Devdiscourse