Building safer digital spaces: Harnessing AI to detect, prevent, and mitigate cyber abuse
Cyber abuse manifests in various forms, including hate speech, cyberbullying, emotional abuse, trolling, and impersonation. These behaviors can lead to severe psychological distress, such as anxiety, depression, and suicidal thoughts, as documented by numerous studies.
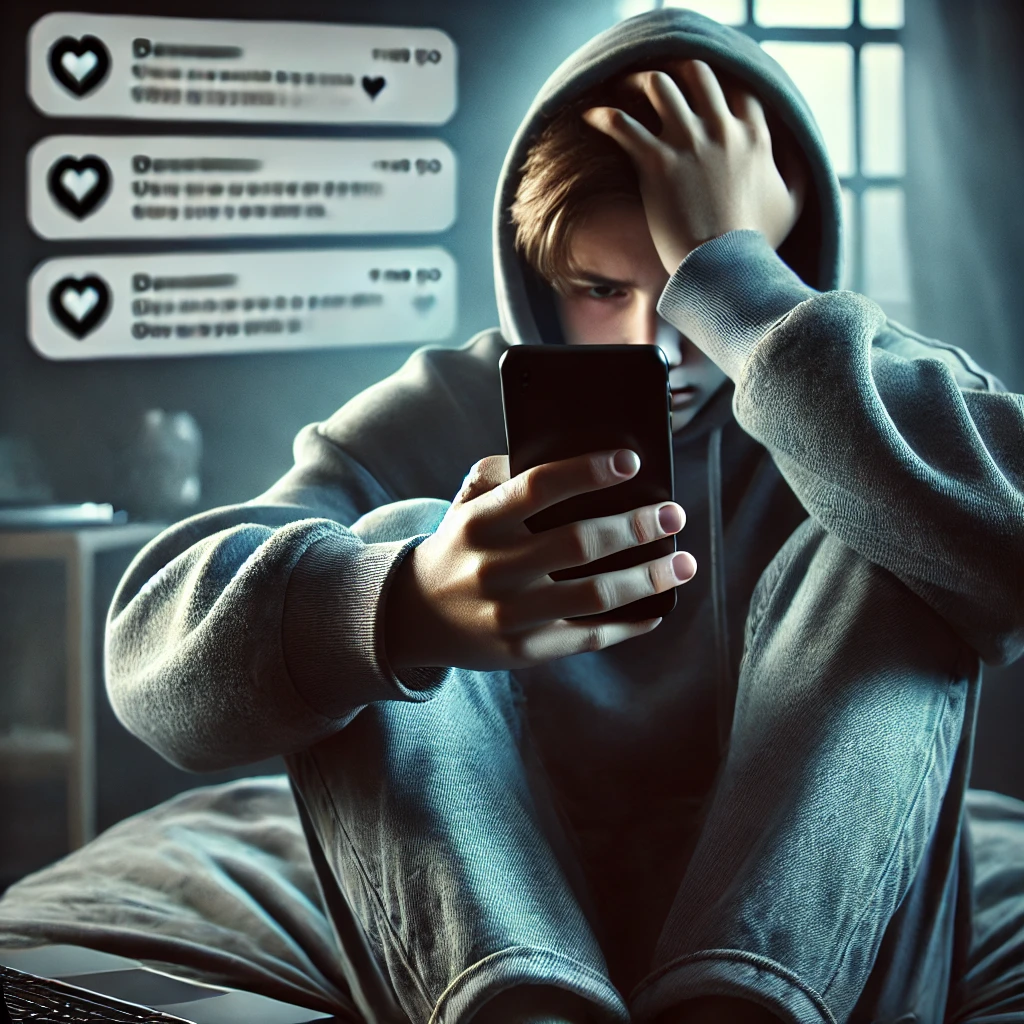
The digital era has brought unparalleled connectivity, reshaping how people communicate, share, and engage. However, this digital connectivity has also given rise to new forms of abuse, such as hate speech, cyberbullying, doxing, trolling, and shaming. In their paper, “A Survey of Textual Cyber Abuse Detection Using Cutting-Edge Language Models and Large Language Models,” J. Angel Diaz-Garcia and Joao Paulo Carvalho explore the potential of advanced AI models to address these challenges.
Available on arXiv, the study provides an in-depth analysis of how cutting-edge language models (LMs) and large language models (LLMs) can improve the detection, classification, and mitigation of cyber abuse while also highlighting their dual-use potential to generate harmful content. This research sheds light on the critical intersection of technology and online safety.
The landscape of cyber abuse
Cyber abuse manifests in various forms, including hate speech, cyberbullying, emotional abuse, trolling, and impersonation. These behaviors can lead to severe psychological distress, such as anxiety, depression, and suicidal thoughts, as documented by numerous studies. The paper categorizes cyber abuse into textual forms, focusing on its implications for individuals and communities. For example, hate speech targets specific groups based on race, gender, or religion, often leading to social fragmentation and personal trauma. Similarly, cyberbullying involves repeated and intentional harm, disproportionately affecting adolescents and young adults.
Advanced generative AI technologies have exacerbated the problem by enabling the creation of realistic fake content, deepfakes, and fabricated textual abuse. Despite these challenges, the research highlights how AI-driven tools can detect abusive behavior, offering promising solutions to these persistent problems.
Role of language models in abuse detection
The study explores how LMs and LLMs, such as BERT, GPT, and RoBERTa, have revolutionized cyber abuse detection. These models excel in natural language processing (NLP), allowing for nuanced analysis of abusive language across platforms. Techniques such as hierarchical attention mechanisms, semi-supervised learning, and data augmentation have enhanced these models' capabilities. For example, models like BERT integrate contextual embeddings to improve accuracy in identifying subtle forms of hate speech, while hierarchical attention mechanisms enable multi-layered analysis of text, capturing deeper linguistic features.
Moreover, the study addresses the challenges of unbalanced datasets, a common issue in cyber abuse detection. Techniques such as oversampling, weighted metrics, and generative data augmentation help mitigate biases, improving model performance in underrepresented categories of abuse.
The paper makes several significant contributions to the field:
-
Comprehensive Coverage of Abuse Forms: Unlike prior studies that primarily focus on hate speech and cyberbullying, this survey encompasses less-explored forms of abuse, such as doxing, impersonation, and cancel culture. By broadening the scope, the research identifies critical gaps in existing detection frameworks.
-
Evaluation of Language Models: The study examines how different LMs and LLMs perform across tasks, emphasizing their strengths and weaknesses. For instance, it notes that ensemble methods combining multiple pre-trained models often outperform standalone approaches, particularly in imbalanced datasets.
-
Emerging Trends and Challenges: The research highlights the potential for generative AI to not only detect but also inadvertently amplify cyber abuse. It calls for robust ethical frameworks and transparency in model development to mitigate these risks.
Roadblocks and pathways forward
While LMs and LLMs show promise, the paper identifies key challenges in their application. One major issue is language diversity. Most models are trained predominantly on English datasets, leaving gaps in their ability to detect abuse in other languages or dialects. Additionally, cyber abuse often includes multimodal elements, such as images or videos accompanying abusive text, requiring integrated solutions that analyze multiple data types simultaneously.
Another challenge lies in dataset quality and availability. Existing datasets may be outdated, unbalanced, or limited in scope, leading to biased or incomplete models. Addressing this requires ongoing efforts to create comprehensive, representative datasets that capture the full range of abuse types and contexts.
Explainability in AI is another pressing concern. Detection models often operate as “black boxes,” making their decision-making processes difficult to interpret. This lack of transparency can hinder trust in AI-driven solutions, particularly when addressing sensitive issues like abuse.
The authors advocate for developing multilingual and multimodal models that can handle diverse languages and integrate textual, visual, and behavioral data for holistic abuse detection. Moreover, they stress the need for ethical AI frameworks to ensure responsible deployment. This includes measures to prevent the misuse of generative AI for creating abuse, as well as transparent auditing of AI systems to identify and mitigate biases.
- FIRST PUBLISHED IN:
- Devdiscourse