Early detection, better outcomes: The AI breakthrough in heart disease prevention
One of the most promising applications of the EMLP framework lies in its integration with Internet of Things (IoT) devices. Wearable health monitors, for example, can collect real-time data on vital signs and transmit this information to cloud platforms powered by the EMLP model. Such systems can provide instantaneous risk assessments, alerting patients and healthcare providers to potential issues before they escalate.
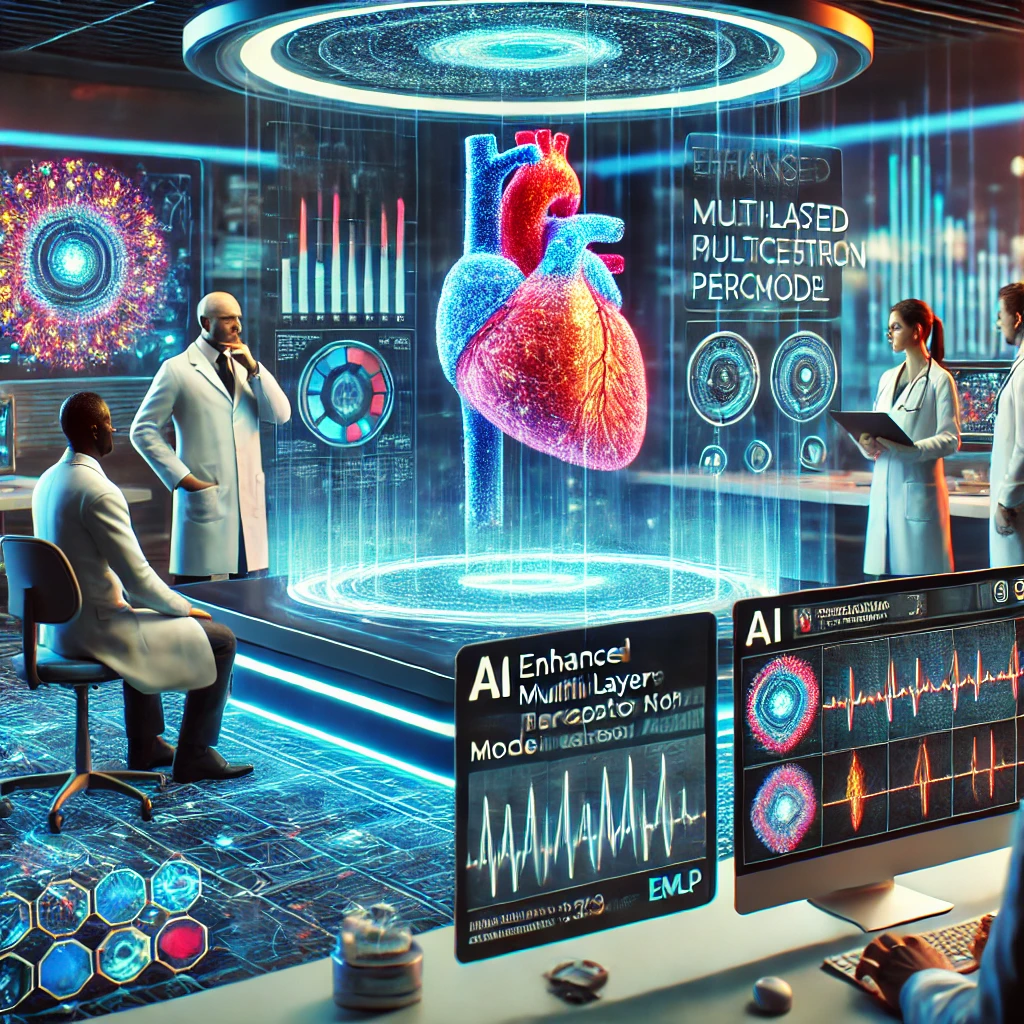
Cardiac disease is one of the leading causes of death globally. Early diagnosis and timely intervention play a critical role in reducing the staggering mortality rate associated with it. However, traditional diagnostic approaches often fall short, either due to limited accessibility or their susceptibility to human error.
Addressing this gap, Monir Abdullah from the University of Bisha presents a novel artificial intelligence-based solution in the study, “Artificial Intelligence-Based Framework for Early Detection of Heart Disease Using Enhanced Multilayer Perceptron,” published in Frontiers in Artificial Intelligence, Volume 7. This research explores the transformative potential of machine learning, specifically the Enhanced Multilayer Perceptron (EMLP), in creating a highly accurate and efficient diagnostic tool for heart disease.
The Enhanced Multilayer Perceptron: A novel framework
At the core of this research lies the Enhanced Multilayer Perceptron (EMLP), a significant improvement over traditional machine learning algorithms. The EMLP addresses the shortcomings of existing models such as decision trees, Naïve Bayes classifiers, and even deep learning approaches like CNNs and LSTMs, which often suffer from overfitting or demand high computational resources. By integrating advanced techniques such as optimized weight initialization, adaptive learning rates, and additional hidden layers, the EMLP model achieves a balance of precision and resource efficiency.
The study utilized the CDC’s cardiac disease dataset, comprising over 300,000 patient records. This dataset included 17 features - such as age, body mass index (BMI), smoking habits, physical activity levels, and pre-existing conditions like diabetes or stroke history - key indicators often associated with cardiovascular risk. The EMLP was designed to process these features, uncovering subtle patterns that link them to the likelihood of heart disease. Comprehensive data preprocessing ensured the dataset was free of missing values and that categorical variables were numerically encoded to enhance the model’s compatibility and performance.
Unlike traditional models, which often struggle to handle complex, non-linear relationships within datasets, the EMLP leverages its advanced architecture to identify intricate correlations. Its design not only reduces prediction errors but also ensures a robust capability to generalize across diverse patient populations.
Groundbreaking results
The study compared seven machine learning algorithms, ranging from traditional methods like Random Forest and Naïve Bayes to more advanced deep learning models. The EMLP demonstrated superior performance, achieving an accuracy rate of 92%, significantly outperforming Random Forest (90%) and Naïve Bayes (84%). This makes the EMLP one of the most reliable models for predicting heart disease to date.
Beyond accuracy, the EMLP excelled in other critical metrics. It achieved high precision, indicating its ability to accurately predict cases of heart disease without generating excessive false positives. Its recall rates were equally notable, showcasing its efficiency in minimizing false negatives - a particularly crucial factor in medical diagnostics where missed cases can have life-threatening consequences.
The study also analyzed the EMLP’s performance using Receiver Operating Characteristic (ROC) curves and confusion matrices. These evaluations highlighted the model’s ability to maintain high sensitivity and specificity across diverse subsets of data. Unlike conventional models that may struggle in edge cases, the EMLP consistently delivered reliable predictions, underscoring its potential for real-world application.
Transformative implications for healthcare
The implications of this research are far-reaching, particularly in its ability to democratize access to advanced diagnostic tools. The EMLP’s efficiency and scalability make it an ideal candidate for integration into healthcare systems, especially in regions with limited resources. Its reliance on easily obtainable clinical indicators ensures that even basic healthcare facilities can leverage this technology to provide timely and accurate heart disease diagnoses.
One of the most promising applications of the EMLP framework lies in its integration with Internet of Things (IoT) devices. Wearable health monitors, for example, can collect real-time data on vital signs and transmit this information to cloud platforms powered by the EMLP model. Such systems can provide instantaneous risk assessments, alerting patients and healthcare providers to potential issues before they escalate.
Furthermore, the study’s findings have significant implications for personalized medicine. By accurately analyzing individual risk factors, the EMLP framework can help tailor preventive strategies and treatment plans, enhancing patient outcomes while optimizing healthcare resources. In regions where access to specialists is limited, this technology could serve as a critical first line of defense against cardiovascular diseases.
Challenges
While the EMLP represents a significant advancement, it is not without challenges. Data privacy and security remain critical concerns, particularly when dealing with sensitive health information. Ensuring compliance with privacy regulations, such as GDPR and HIPAA, will be essential for the widespread adoption of this technology.
Additionally, the model requires further validation across diverse populations to ensure its generalizability. The current dataset, while comprehensive, may not capture all variations in demographics, genetic predispositions, and environmental factors. Future research should focus on expanding the dataset and incorporating these variations to enhance the model’s robustness.
The authors also propose integrating the EMLP with deep learning techniques and cloud computing infrastructures to further improve its scalability and efficiency. This includes exploring hybrid models that combine the strengths of EMLP with other machine learning paradigms, as well as developing secure, decentralized frameworks to address privacy concerns. The integration of Internet of Medical Things (IoMT) systems could also open new frontiers in real-time, preventive healthcare solutions.
- FIRST PUBLISHED IN:
- Devdiscourse