Trustworthy vs reliable AI: How labels shape our confidence in automotive technology
The distinction between trust and reliability in AI is not merely semantic; it has profound implications for how these systems are evaluated and used. Trust implies moral sensitivity, benevolence, and accountability - qualities typically associated with humans. In contrast, reliability focuses purely on performance and consistency, devoid of human-like attributes.
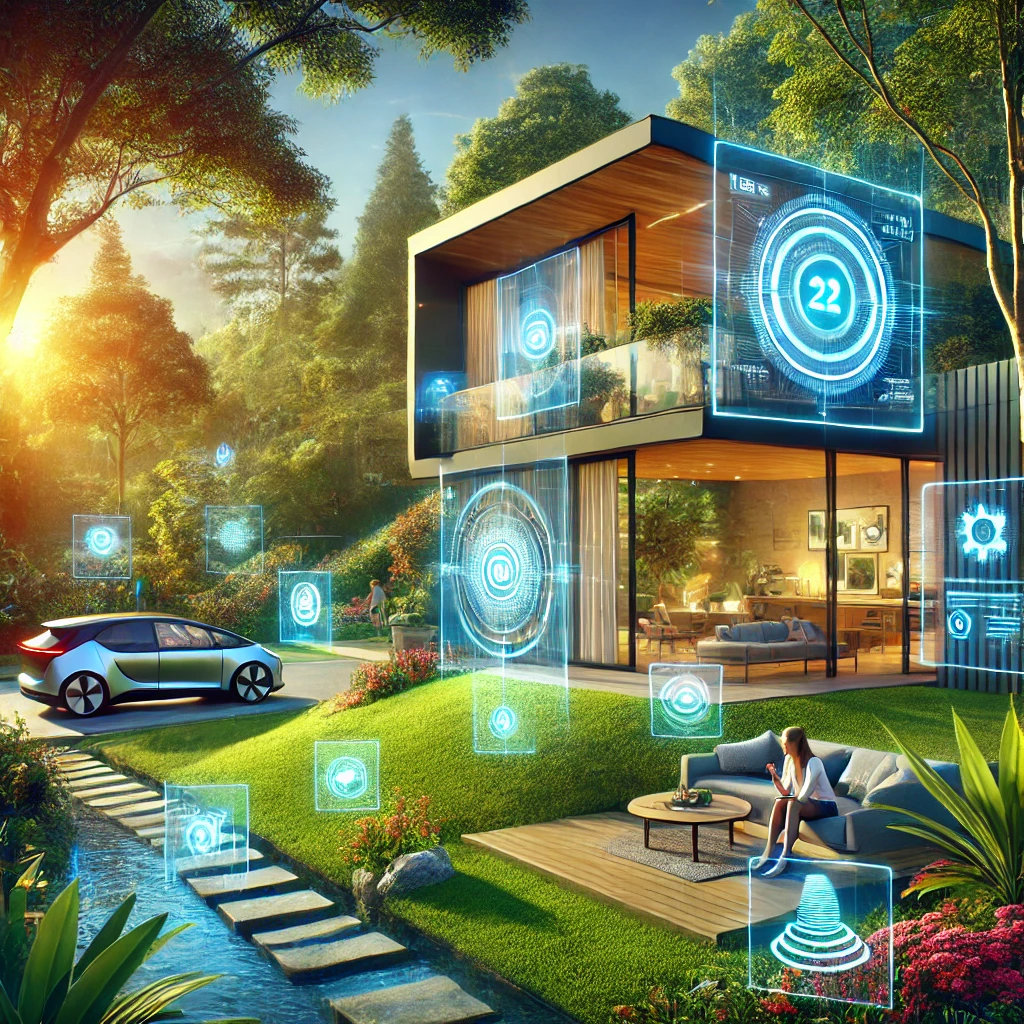
The integration of AI into daily life has brought to light an essential question: how does the way we describe AI influence its acceptance and usability? Labels like "trustworthy AI" and "reliable AI" are commonly used by manufacturers and regulators, but how do these terms shape user attitudes and expectations? A recent study titled “The Impact of Labeling Automotive AI as Trustworthy or Reliable on User Evaluation and Technology Acceptance,” published in Scientific Reports 15, 1481 (2025), seeks to unravel this question. Conducted by John Dorsch and Ophelia Deroy, the research explores how these labels influence perceptions of AI-assisted automotive technologies, shedding light on the intersection of language, ethics, and user behavior.
Trust vs. reliability in AI
The distinction between trust and reliability in AI is not merely semantic; it has profound implications for how these systems are evaluated and used. Trust implies moral sensitivity, benevolence, and accountability - qualities typically associated with humans. In contrast, reliability focuses purely on performance and consistency, devoid of human-like attributes. Philosophers argue that while it may be irrational to "trust" machines in a moral sense, emphasizing reliability could offer a more practical and ethically sound framework. This study delves into how these differing labels affect user perceptions, particularly in the context of automotive AI.
The research employed a one-way between-subjects design with 478 participants divided into two groups: one exposed to the term "trustworthy AI" and the other to "reliable AI." Participants were presented with guidelines corresponding to their assigned label and asked to evaluate three AI-assisted driving scenarios - planning, parking, and steering assistance. Responses were measured using a streamlined version of the Technology Acceptance Model (TAM), focusing on factors such as perceived ease of use, usefulness, and trust. The researchers also assessed specific constructs like AI accountability, blameworthiness, and human-like trust through vignette-based surveys.
The study uncovered nuanced differences in how the labels "trustworthy" and "reliable" shaped user perceptions:
-
No Significant Difference in Blame and Accountability: Participants did not exhibit significant differences in their evaluations of AI accountability or blameworthiness between the two labels, indicating that neither term influenced how users attributed responsibility.
-
Perceived Ease of Use and Benevolence: The "trustworthy AI" label significantly enhanced perceptions of ease of use and benevolence, suggesting an anthropomorphic effect where users ascribed human-like qualities, such as care and goodwill, to the AI system.
-
Stereotypes of Human-Like AI: While the "trustworthy AI" label encouraged a positive view of the system's intent, it did not translate to higher confidence in using the technology or a stronger intention to adopt it. This highlights a disconnect between perceptions of trustworthiness and practical usability.
Designing labels for better AI adoption
The findings suggest that labeling AI as "trustworthy" may inadvertently anthropomorphize the technology, leading users to expect qualities that AI cannot deliver. This could erode user confidence when the system falls short of these expectations. By contrast, emphasizing reliability aligns more closely with AI's operational strengths, offering a realistic framework for user interactions.
For developers and policymakers, these insights underscore the importance of choosing labels that manage expectations effectively. Highlighting reliability could mitigate issues like algorithm aversion, where users reject AI despite its proven benefits. Additionally, crafting clear communication strategies around AI's capabilities and limitations could enhance user acceptance and trust in emerging technologies.
Challenges and limitations
The study acknowledges several limitations. First, the observed effects were modest and may have been influenced by the specific wording of the definitions provided to participants. Second, the research focused exclusively on automotive AI, limiting its generalizability to other domains like healthcare or customer service. Future studies should explore how trust and reliability labels impact different types of AI technologies and user demographics.
Another critical limitation is the potential for pre-existing user biases to affect responses. Participants may have formed opinions about AI's trustworthiness or reliability before the experiment, which could have diluted the impact of the labels. Addressing these issues in future research would provide a more comprehensive understanding of the role language plays in shaping perceptions of AI.
Toward ethical and effective AI communication
Future research should expand on these findings by examining the effects of trust and reliability labels in a broader range of applications, including AI-driven healthcare and customer service. Exploring the role of cultural and demographic factors in shaping user perceptions could also yield valuable insights. Moreover, integrating real-world scenarios and longitudinal studies would help assess the long-term impact of these labels on user behavior and technology adoption.
Developers and regulators must also engage in a deeper ethical conversation about the language used to describe AI. While trust may be a compelling marketing term, it risks misleading users about AI's capabilities. A focus on reliability, coupled with transparent communication, offers a path toward building realistic and sustainable user relationships with AI technologies.
- FIRST PUBLISHED IN:
- Devdiscourse