A shield against the invisible: Tackling insider threats in the AI age
The DS-IID model, while primarily designed to address insider threats, demonstrates versatility that extends beyond this specific domain. Its ability to analyze relational datasets and detect anomalies positions it as a powerful tool for various sectors, including financial fraud detection, healthcare data protection, and industrial control systems security.
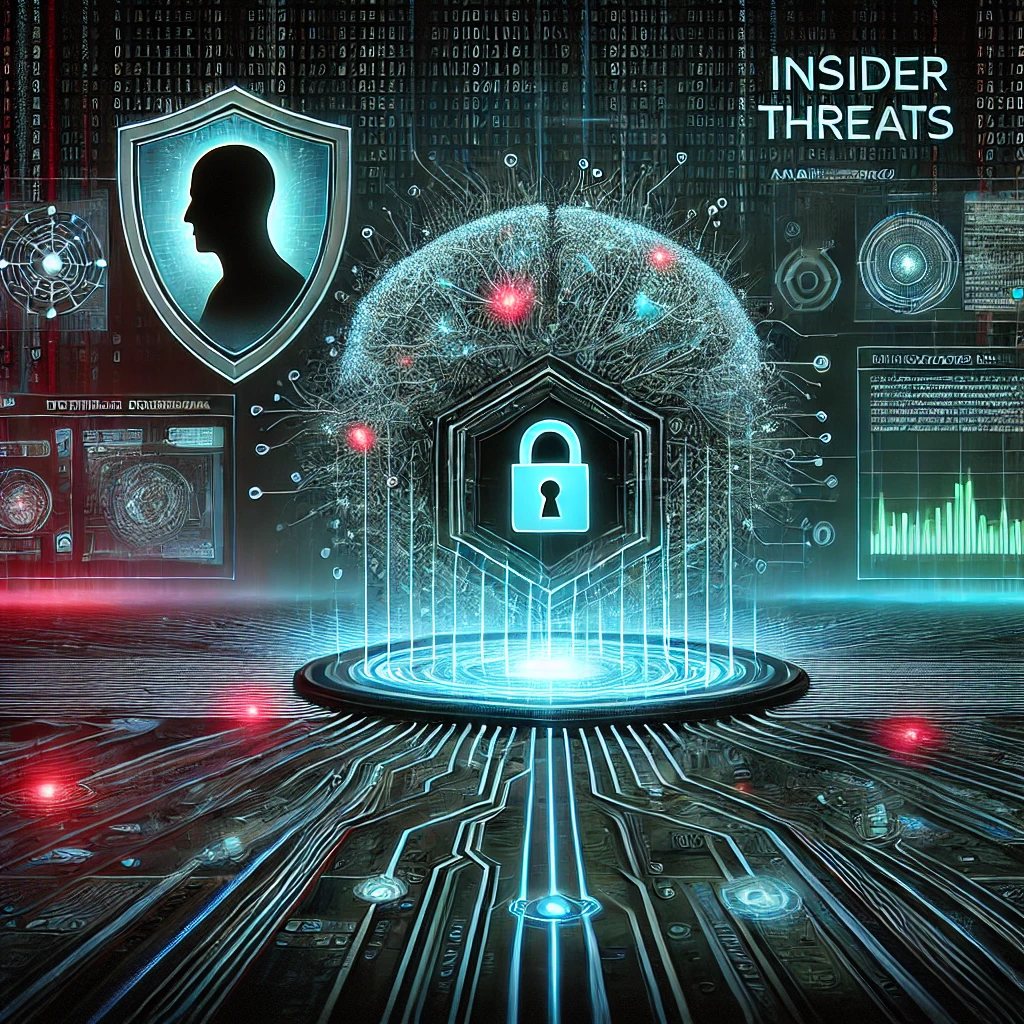
Insider threats are among the most challenging and costly cybersecurity risks organizations face today. Unlike external attacks, these threats arise from within - trusted individuals with legitimate access exploiting their positions. Whether intentional or accidental, insider actions often lead to data breaches, intellectual property theft, or system sabotage. A recent surge in the use of generative AI technologies has exacerbated the problem, enabling the creation of synthetic user profiles and behaviors that closely mimic legitimate actions, making detection more difficult than ever.
Addressing these vulnerabilities, a paper titled “A Novel Deep Synthesis-Based Insider Intrusion Detection (DS-IID) Model for Malicious Insiders and AI-Generated Threats,” published in Scientific Reports, introduces an innovative approach. Led by Hazem M. Kotb and colleagues, the research proposes a groundbreaking Deep Synthesis Insider Intrusion Detection (DS-IID) model to tackle the complexities of insider threats in the era of generative AI.
The DS-IID Model: A technological leap in intrusion detection
The DS-IID model integrates cutting-edge methodologies to address the complexities of modern insider threats. Its design is a blend of automated feature synthesis, synthetic data generation, and deep learning, providing a robust framework for detecting anomalous behaviors.
The model operates on three core pillars:
- Deep Feature Synthesis (DFS): Deep Feature Synthesis automates the generation of user profiles by analyzing relational datasets. By extracting and synthesizing features from user activity logs, DFS identifies behavioral patterns that deviate from normal operations. This approach eliminates the need for manual feature engineering, making the model scalable and adaptable to various organizational contexts.
- Synthetic User Profile Generation: To counter the threat of AI-generated synthetic profiles, the model employs Conditional Tabular Generative Adversarial Networks (CTGAN) and Tabular Variational Autoencoders (TVAE). These algorithms create synthetic datasets that replicate real-world user behaviors, enabling the model to simulate and learn from potential attack scenarios. This capability is crucial for staying ahead of generative AI-powered threats, which can obscure malicious intent behind layers of plausible behavior.
- Binary Deep Learning Classification: The core of the DS-IID model is its binary deep learning classifier, which is optimized to distinguish between normal and anomalous behaviors. The model leverages neural network architectures to analyze user activity data and determine the likelihood of malicious intent. With an accuracy exceeding 97% and an AUC of 0.99, the classifier demonstrates exceptional reliability, even in complex threat scenarios.
The DS-IID model was rigorously tested using the CERT insider threat dataset, which includes a variety of realistic scenarios such as unauthorized data uploads, exfiltration attempts, and keylogger deployments. The results were groundbreaking, with the model achieving:
- Detection Accuracy: Over 97% in identifying malicious activities.
- Precision Against Synthetic Threats: Over 99% in distinguishing real from synthetic user profiles.
- Balanced Metrics: An optimal balance of recall and precision, minimizing both false positives and negatives.
These results underscore the model’s ability to handle both traditional insider threats and novel AI-generated risks. Its capacity to analyze complex datasets and adapt to evolving threat landscapes makes it a transformative tool for modern cybersecurity challenges.
Implications for cybersecurity
The DS-IID model’s introduction has significant implications for the cybersecurity domain. By providing a scalable, real-time solution for detecting insider threats, it addresses a critical gap in organizational security infrastructures. The integration of automated feature synthesis not only enhances detection capabilities but also reduces the resource intensity of manual monitoring.
Furthermore, the model’s use of synthetic data generation ensures resilience against future threats, including those powered by advanced generative AI technologies. As attackers become more sophisticated, tools like DS-IID offer a proactive defense strategy, enabling organizations to anticipate and neutralize threats before significant damage occurs.
Broader applications
The DS-IID model, while primarily designed to address insider threats, demonstrates versatility that extends beyond this specific domain. Its ability to analyze relational datasets and detect anomalies positions it as a powerful tool for various sectors, including financial fraud detection, healthcare data protection, and industrial control systems security. The framework’s adaptability ensures it can cater to the unique challenges of different industries, providing robust and reliable solutions to pressing cybersecurity needs.
This study also opens exciting avenues for further development and innovation. Expanding the diversity of training datasets would enhance the model’s ability to handle a broader spectrum of threat scenarios, improving its overall resilience. Incorporating multimodal inputs, such as voice or video data, offers the potential to significantly enrich its detection capabilities. Optimizing the model for edge computing would facilitate real-time deployment in distributed environments, ensuring quicker responses to emerging threats. Additionally, integrating the DS-IID framework with threat intelligence platforms could enable a more coordinated and comprehensive approach to organizational security.
- FIRST PUBLISHED IN:
- Devdiscourse