Can AI truly mimic human writing? A look through the data lens
While LLMs excel in producing coherent and contextually relevant text, their inability to replicate the intricate structural patterns of human writing underscores the need for further innovation. Fine-tuning parameters such as temperature offers a partial solution, but bridging the gap between human and machine language will require advancements in model design and training methodologies.
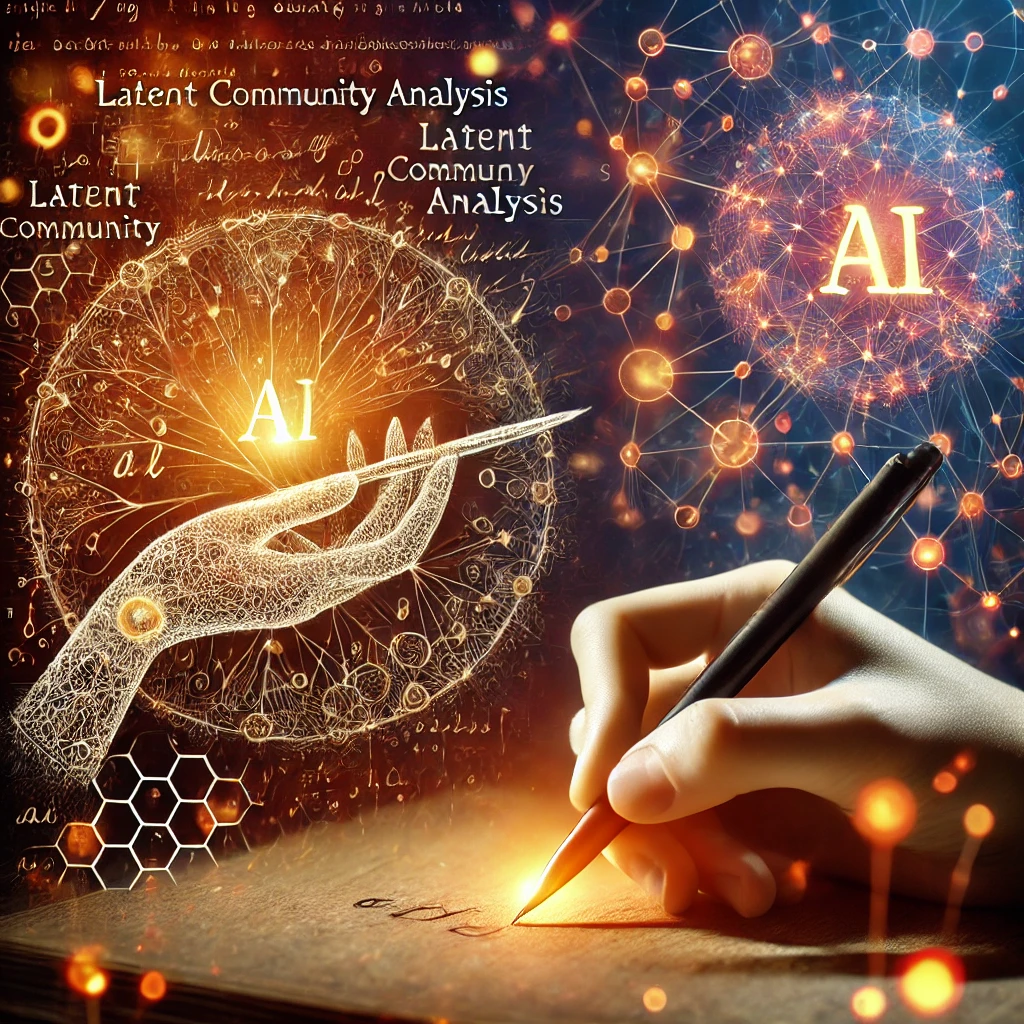
The advent of Large Language Models (LLMs) has transformed the way we interact with technology, from automating customer service to crafting essays and reports. These models, such as OpenAI's GPT-3.5, are celebrated for their ability to produce coherent and contextually relevant text. However, the lingering question remains: how closely does their generated text align with human-authored language in terms of structure, nuance, and creativity?
The study titled "Does a Large Language Model Really Speak in Human-Like Language?" conducted by Mose Park, Yunjin Choi, and Jong-June Jeon from the University of Seoul's Department of Statistical Data Science, delves into this intriguing question. Available on arXiv, the research uses a statistical lens to analyze the latent community structures in text, shedding light on the similarities and disparities between human and LLM-generated language.
A deep dive into latent community structures
The study employed an innovative approach by analyzing three distinct datasets to understand the structural differences between human-written and LLM-generated text. The first dataset consisted of original human-authored reviews, representing natural linguistic patterns and serving as the baseline for comparison. The second dataset contained paraphrased versions of these reviews generated by GPT-3.5, reflecting the LLM's interpretation of human text. The third dataset took this a step further, using GPT-3.5 to paraphrase its own output, creating a twice-paraphrased dataset.
To compare these datasets, the researchers utilized latent community structure analysis, a technique that examines the underlying relationships and groupings within textual data. By applying hypothesis testing, they assessed whether the structural characteristics of the LLM-generated text aligned with those of human-authored text. The analysis revealed significant insights: while GPT-3.5 generated grammatically correct and contextually coherent text, its latent structures exhibited notable differences from human-authored language. The asymmetry in structural gaps between human and LLM-generated datasets highlighted the inability of current LLMs to fully replicate the nuanced patterns inherent in human writing.
Fine-tuning LLM outputs
Another critical aspect of the study explored the impact of adjusting GPT-3.5’s temperature parameter. This parameter governs the variability of generated text, with lower values producing more deterministic and predictable outputs, and higher values generating diverse and creative responses.
At lower temperature settings (e.g., 0.1), GPT-3.5's paraphrased text closely resembled the input, emphasizing accuracy and consistency. However, this came at the expense of creativity, as the generated text lacked the stylistic diversity characteristic of human writing. Conversely, higher temperatures (e.g., 1.5) introduced variability, resulting in text that was more creative but often diverged in tone and content from the original.
This experiment underscored the delicate balance between creativity and fidelity in LLM-generated text. The findings suggest that while parameter adjustments can enhance certain aspects of output, achieving human-like structural complexity remains a challenge.
The study’s nuanced analysis revealed that human-authored language possesses a depth of latent community structures that LLMs struggle to replicate. These structures, which reflect the implicit relationships and patterns in text, are shaped by human cognition, cultural context, and individual creativity. In contrast, LLM-generated text, while coherent, is constrained by the limitations of its training data and algorithmic design.
Furthermore, the study demonstrated that the structural differences between human and machine-generated text persist even when LLMs are tasked with paraphrasing their own outputs. This suggests that current LLM architectures may lack the inherent flexibility required to fully mimic human linguistic patterns.
Bridging the gap between human and machine language
The study offers critical insights into the capabilities and limitations of LLMs like GPT-3.5. Through rigorous statistical analysis, the researchers illuminate the challenges of achieving human-like text generation, highlighting the distinct latent structures that define human-authored language.
While LLMs excel in producing coherent and contextually relevant text, their inability to replicate the intricate structural patterns of human writing underscores the need for further innovation. Fine-tuning parameters such as temperature offers a partial solution, but bridging the gap between human and machine language will require advancements in model design and training methodologies.
As LLMs continue to permeate various industries, understanding their linguistic limitations and potential remains essential. This research not only enriches our comprehension of LLM capabilities but also paves the way for future efforts to enhance the human-like quality of AI-generated text. By addressing these challenges, we can unlock the full potential of LLMs, ensuring they serve as reliable, creative, and insightful tools in an increasingly AI-driven world.
- FIRST PUBLISHED IN:
- Devdiscourse