Breaking barriers: Tackling cybersickness for widespread adoption of virtual reality
By bridging the gap between immersive technology and user-centric design, this research paves the way for a future where VR’s transformative potential can be fully realized without compromise.
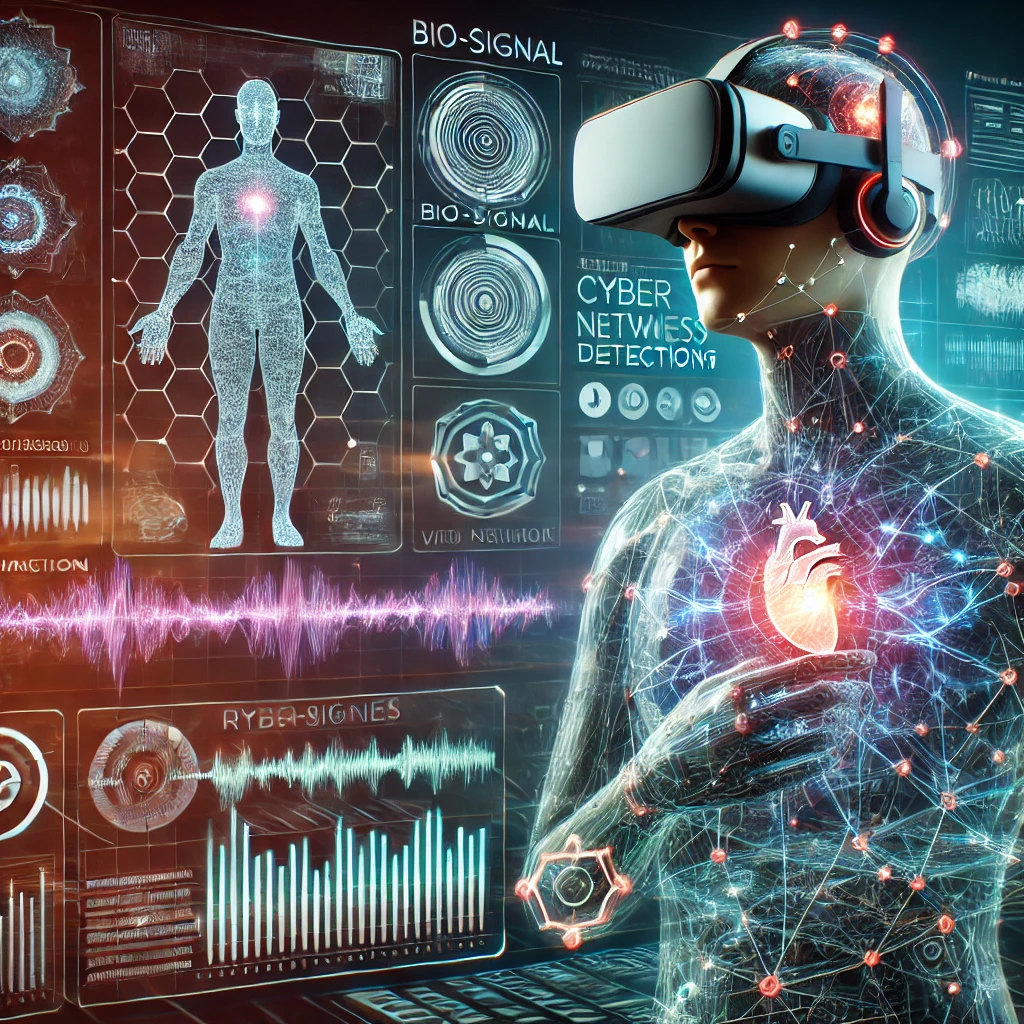
Virtual Reality (VR) has transformed industries such as healthcare, gaming, and industrial safety, offering immersive environments that enhance user interaction and experience. However, the phenomenon of cybersickness—a form of motion sickness characterized by dizziness, nausea, and discomfort—remains a significant obstacle to the widespread adoption of VR technologies. Traditional methods for assessing and mitigating cybersickness often rely on subjective measures like questionnaires, which lack precision and real-time applicability.
A paper titled "Real-time Cross-modal Cybersickness Prediction in Virtual Reality", by Yitong Zhu, Tangyao Li, and Yuyang Wang from The Hong Kong University of Science and Technology, presents an innovative solution to this challenge. Available on arXiv, the research proposes a lightweight, transformer-based model capable of real-time cybersickness prediction by integrating bio-signal and visual data.
Merging bio-signal and visual data
The study introduces a cross-modal prediction framework that enhances the accuracy of cybersickness detection by combining physiological signals with visual data. This innovative system comprises three interconnected components. First, the Bio-Signal Encoder processes physiological data such as eye and head movements, which are reliable indicators of discomfort. Utilizing sparse self-attention mechanisms, this module captures long-term temporal dependencies, enabling it to identify patterns associated with cybersickness while maintaining computational efficiency. Second, the Video Encoder, based on the PP-TSN framework, analyzes dynamic visual stimuli from VR content. By extracting critical features, it identifies potential contributors to sensory conflicts, such as abrupt scene transitions or inconsistent motion cues. Finally, the Cross-Modal Fusion Module integrates the outputs from the bio-signal and video encoders into a shared semantic space. This integration allows the system to understand and model the complex interactions between a user's physiological responses and the visual stimuli in VR environments, significantly enhancing its ability to predict cybersickness with high accuracy.
The framework is designed for real-time applications, ensuring low latency without compromising predictive performance. Unlike traditional models, which often rely on single-modality inputs, this cross-modal approach leverages the complementary strengths of bio-signal and visual data to provide a more holistic understanding of cybersickness triggers.
State-of-the-art performance
To evaluate the framework’s effectiveness, the researchers conducted experiments using a public dataset containing VR videos, eye and head tracking data, and other physiological signals. The results demonstrated the model’s superior performance compared to existing methods.
With video data alone, the model achieved a prediction accuracy of 93.13%, significantly outperforming traditional architectures while maintaining a lightweight design. When bio-signal data was integrated into the framework, the accuracy improved further, showcasing the advantages of cross-modal fusion. The findings underscored that combining visual and physiological inputs is essential for capturing the complex, multifaceted nature of cybersickness.
Moreover, the model’s lightweight design ensures compatibility with real-world VR systems, where computational efficiency is critical. This scalability makes the framework suitable for a wide range of applications, from gaming consoles to industrial VR simulations.
Implications
This research has profound implications for the future of VR technology. By enabling real-time prediction of cybersickness, the framework paves the way for adaptive VR systems that can dynamically adjust content to minimize user discomfort. For example, VR applications could modify visual stimuli, reduce motion intensity, or adjust camera angles based on the system’s predictions, creating a more personalized and comfortable experience.
The study also highlights the potential of cross-modal learning in enhancing the usability of immersive technologies. Integrating data from multiple modalities allows for a more comprehensive understanding of user experiences, which could extend beyond VR to fields like augmented reality (AR) and mixed reality (MR).
Future research directions include expanding the dataset to encompass a broader range of VR applications and user demographics. Incorporating additional physiological signals, such as heart rate variability, skin conductance, or even EEG data, could further improve prediction accuracy. Additionally, optimizing the framework for diverse VR hardware platforms would ensure accessibility and adaptability across different industries.
Challenges and opportunities
While the study marks a significant step forward, certain challenges remain. For instance, ensuring the seamless integration of the model into existing VR systems without affecting user experience requires careful engineering. Furthermore, ethical considerations around the collection and use of physiological data must be addressed to protect user privacy and build trust.
Despite these challenges, the opportunities are immense. By reducing cybersickness, VR systems can reach broader audiences, including individuals who previously avoided VR due to discomfort. This could accelerate the adoption of VR in education, training, and therapeutic applications, unlocking its full potential as a transformative technology.
By bridging the gap between immersive technology and user-centric design, this research paves the way for a future where VR’s transformative potential can be fully realized without compromise.
- FIRST PUBLISHED IN:
- Devdiscourse