Improving Visual Geo-localization: Integrating Textual Data with CLIP for Precision
Researchers from the Shanghai Institute of Microsystem and Information Technology propose a two-stage training method leveraging CLIP's multi-modal capabilities to enhance visual geo-localization accuracy by incorporating textual descriptions, achieving competitive results on large-scale datasets. This approach addresses the limitations of traditional visual methods, fostering more reliable applications in fields like autonomous driving and urban planning.
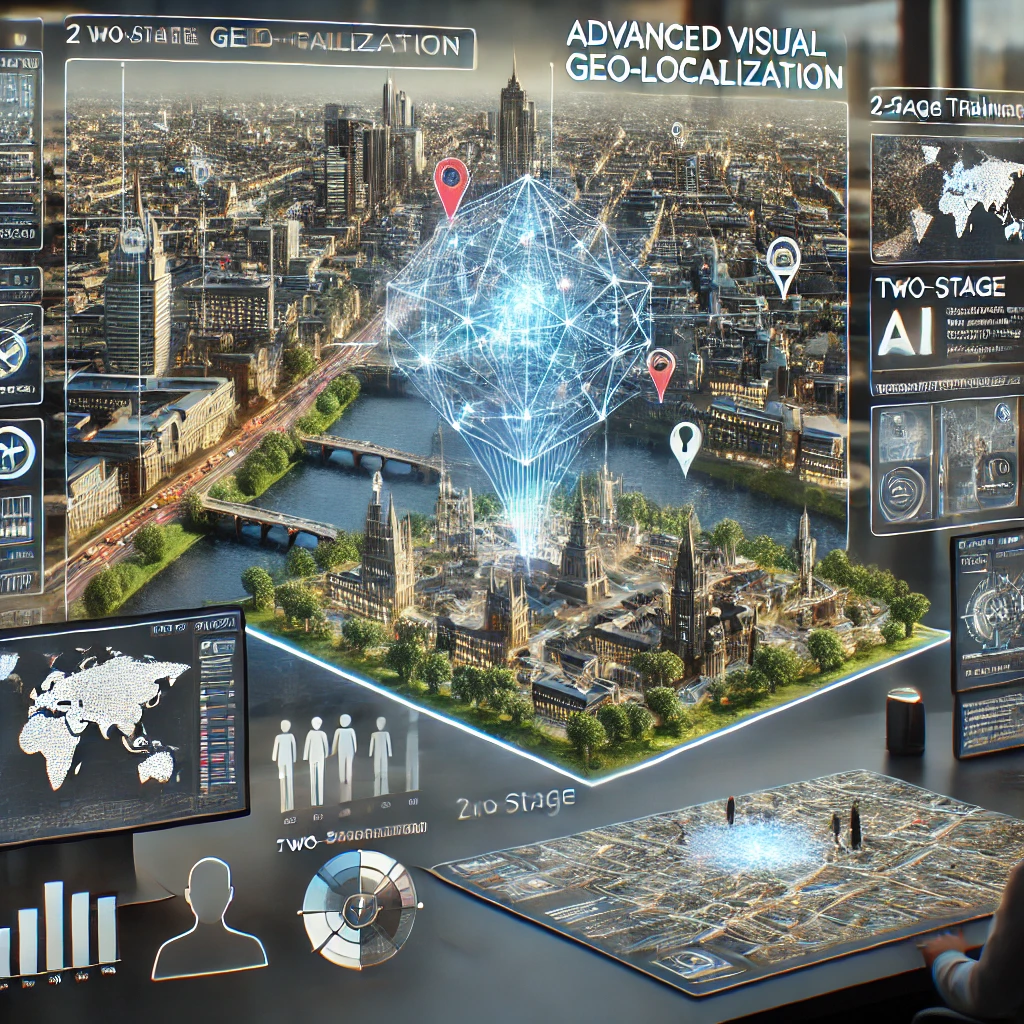
A study by Jingqi Hu and Chen Mao from the Shanghai Institute of Microsystem and Information Technology, Chinese Academy of Sciences, delves into the challenges and advancements in the field of visual geo-localization (VG), a process crucial for applications such as robotics, autonomous driving, augmented reality, and geographic information systems (GIS). VG involves identifying the location depicted in query images, which is inherently challenging due to the variations in perspective, scale, and environmental conditions that images can present. Traditional VG methods primarily rely on visual features extracted using convolutional neural networks (CNNs). These methods, while effective to an extent, often falter under extreme conditions like poor lighting or significant seasonal changes, which lead to considerable appearance differences between the query images and the database images. As a result, the robustness and discriminability of these methods are compromised, limiting their generalizability and practical application.
A Novel Two-Stage Training Method for Visual Geo-localization
To address these limitations, the authors propose a novel two-stage training method that leverages the multi-modal capabilities of the CLIP (Contrastive Language-Image Pretraining) model. This approach aims to enhance the visual performance by incorporating textual descriptions, thus providing a richer semantic understanding of the geographic images. In the first stage of training, the researchers create a set of learnable text prompts for each geographic image feature. These prompts generate vague descriptions that assist the image encoder in learning better and more generalizable visual features. The methodology involves using CLIP's image and text encoders to process the images and texts, respectively, with the goal of making their embeddings more similar through a contrastive learning process. This stage optimizes the correspondence between visual and text features, thereby improving the model's ability to understand and represent the relationship between images and texts.
Enhancing Matching Performance with CosPlace and Triplet Loss
The second stage of training builds on the foundations laid in the first stage. The text prompts generated earlier are now used to guide the training of the image encoder. The researchers employ the CosPlace method to categorize the dataset using UTM coordinates, effectively dividing the database into square geographic cells. During this stage, the text encoder remains frozen, and only the image encoder participates in the training process. This approach helps convert the content of the images into semantic information, which is further enriched by the extracted text features. The training is aimed at enhancing the matching performance between query and database images. Moreover, the introduction of triplet loss, a method commonly used in metric learning, contributes to the model's robustness and ability to generalize to other domains. Triplet loss functions by ensuring that the distance between an anchor image and a positive image (an image of the same location) is smaller than the distance between the anchor image and a negative image (an image of a different location).
Validation through Extensive Experiments
The researchers validate the effectiveness of the ProGEO method through extensive experiments on several large-scale VG datasets, including Pitts30k, Pitts250k, Tokyo24/7, SF-XL, St Lucia, and Mapillary Street Level Sequences (MSLS). The results from these experiments demonstrate that ProGEO achieves competitive performance compared to state-of-the-art methods, particularly in challenging scenarios where fine-grained geographic images lack precise text descriptions. This indicates that the combination of visual and textual information significantly enhances the model's ability to accurately and efficiently perform geo-localization tasks.
Impact and Future Possibilities
A critical aspect of this study is its contribution to the broader field of VG by addressing the limitations of purely visual methods. By integrating textual descriptions through a multi-modal approach, ProGEO not only improves the accuracy of VG tasks but also opens up new possibilities for their application in real-world scenarios. The researchers have made their code and model publicly available, encouraging further exploration and development in this field. This accessibility is likely to foster innovation and refinement of VG techniques, benefiting various applications that rely on accurate location identification from images.
In summary, the ProGEO method represents a significant advancement in the field of visual geo-localization. By effectively leveraging the multi-modal capabilities of the CLIP model and introducing a robust two-stage training process, the researchers have developed a model that is both accurate and generalizable. The integration of textual information into the visual geo-localization task addresses a critical gap in existing methodologies, paving the way for more reliable and efficient applications in diverse fields such as autonomous driving, urban planning, and geographic monitoring.
- FIRST PUBLISHED IN:
- Devdiscourse
ALSO READ
Supreme Court Ruling Tightens Legislative Powers in Pakistan
Haryana assembly poll results will again surprise Congress; BJP will register a big win: CM Nayab Singh Saini to PTI.
Trump's Ambitious Deportation Plan: Legal Feasibility and Logistical Challenges
Man Booked for Molesting Infant Niece, Case Registered in Maharashtra's Thane
PIL Against Union Minister Ravneet Singh Bittu Withdrawn: Multiple FIRs Registered