Harnessing AI for Accurate Flood Prediction in South Sudan Amid Climate Change
Researchers from Cairo University and Beni Suef University have developed AI models to improve flood prediction in South Sudan, achieving up to 93.7% accuracy, which could significantly enhance disaster preparedness in the region. Their work underscores the potential of AI in mitigating the impacts of climate change-induced flooding.
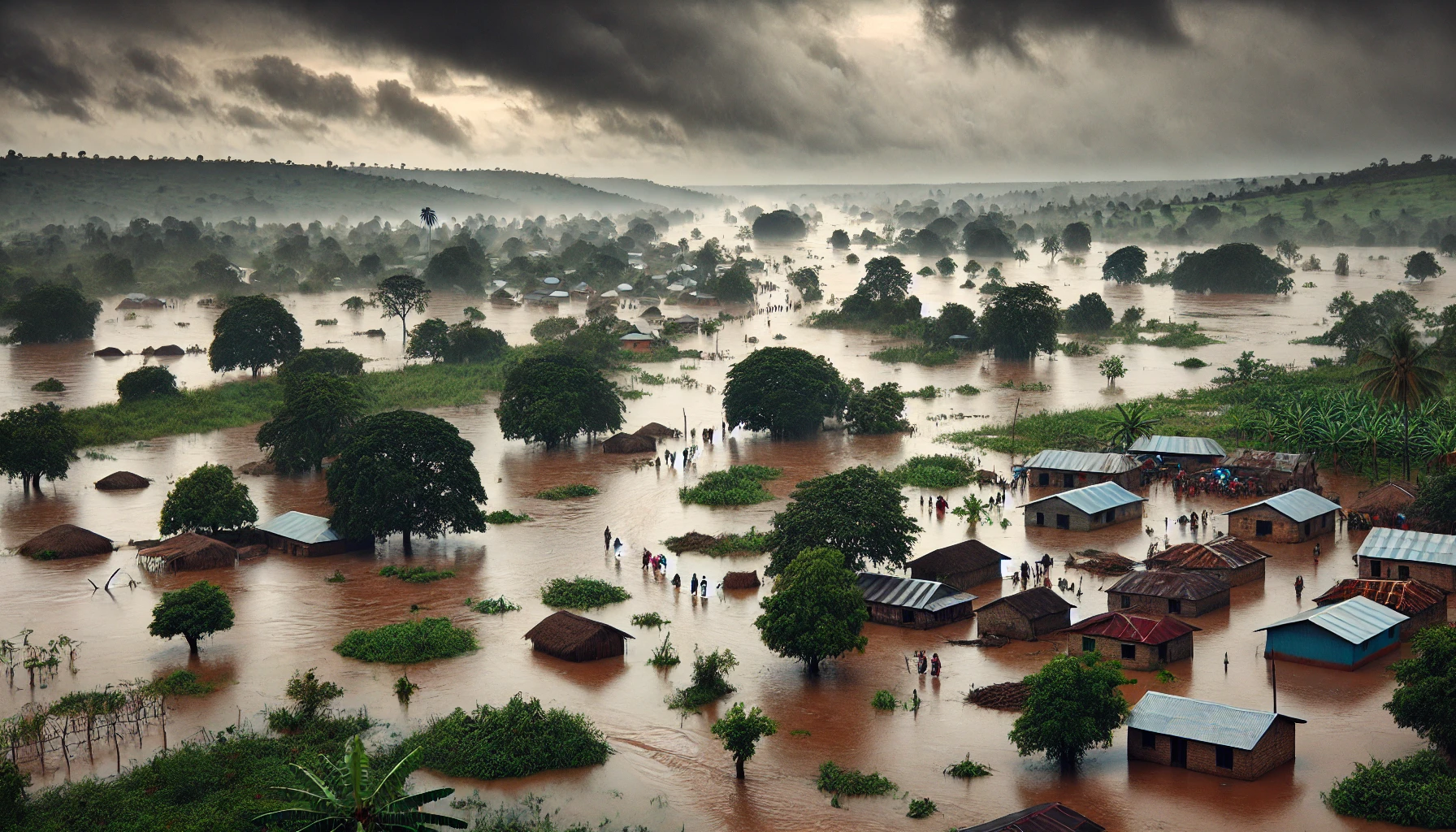
Researchers from Cairo University’s Faculty of African Postgraduate Studies and Beni Suef University’s Faculty of Computers and Artificial Intelligence have conducted a pivotal study on flood classification and prediction in South Sudan using Artificial Intelligence (AI) models, particularly under the pressures of a changing climate. The study addresses the critical issue of flooding in South Sudan, a region severely impacted by the Nile River's overflow during the monsoon season, leading to widespread destruction of infrastructure, displacement of populations, and loss of life. The research utilizes climatic data collected from various stations across South Sudan between 2010 and 2019, applying AI techniques to improve the accuracy of flood predictions. This approach is especially crucial in a region where traditional flood forecasting methods have proven inadequate due to the complexity and variability of contributing factors such as rainfall, river levels, and soil saturation.
Innovative Use of Artificial Neural Networks
The study explores the use of six different Artificial Neural Network (ANN) models to predict floods, comparing their effectiveness in providing accurate forecasts. These models include feedforward (FFNN), general regression (GRNN), recurrent (RNN), cascaded forward (CFNN), probabilistic (PNN), and standard neural networks. Initial tests revealed that the FFNN model performed exceptionally well, achieving a 95% accuracy rate in predicting flood risks. This high level of accuracy is significant as it demonstrates the potential of AI models to significantly improve flood forecasting, enabling better preparation and response strategies. To further refine the models, the researchers experimented with increasing the number of hidden layers within the neural networks. The idea was to assess how deeper networks, which can capture more complex patterns in the data, might enhance predictive accuracy. These tests showed that increasing the hidden layers did indeed affect accuracy, although the degree of improvement varied among the different models.
Gradient Boosting: The Standout Model
Ultimately, the study found that the Gradient Boosting model provided the highest classification accuracy, reaching 93.7%. This model outperformed the others, including AdaBoost, which also showed strong performance with a classification accuracy of 91.6%. The success of these models underscores the value of machine learning techniques in flood prediction, particularly in regions like South Sudan where the impact of climate change is intensifying. By integrating these AI models into flood forecasting systems, it is possible to provide more timely and reliable warnings to communities at risk, thereby reducing the potential for catastrophic damage. This is particularly important in South Sudan, where floods have repeatedly caused severe disruption, destroying homes, farmlands, and essential infrastructure, and forcing thousands of people to flee their homes.
Broad Implications for Disaster Management
The study also emphasizes the broader implications of using AI in disaster management. The researchers suggest that AI models can be further enhanced by incorporating real-time data and additional variables that influence flood risks, such as changes in land use, deforestation, and urbanization. These factors, which are often linked to climate change, contribute to the increasing frequency and severity of floods in many parts of the world, including South Sudan. The ability of AI to process vast amounts of data quickly and accurately makes it an invaluable tool in predicting such complex natural phenomena. Moreover, the study advocates for the continued development of AI-driven flood forecasting systems, particularly in regions with limited data availability or where traditional methods have proven ineffective. The use of AI in this context not only improves the accuracy of predictions but also helps in optimizing resource allocation and emergency response efforts, ultimately saving lives and reducing economic losses.
Challenges in Data Collection and Processing
In addition to its technical findings, the study also highlights the challenges faced by researchers in gathering and processing the data needed for AI modeling in South Sudan. The region's infrastructure limitations, coupled with the difficulties of accessing reliable data, posed significant obstacles. Despite these challenges, the researchers were able to compile a comprehensive dataset that included various climatic and geographical parameters, which were crucial for training and testing the AI models. The success of this research demonstrates the feasibility of applying advanced AI techniques in data-poor regions, offering a blueprint for similar efforts in other vulnerable areas. Furthermore, the study calls for future research to explore even more sophisticated AI models and to integrate additional data sources, such as satellite imagery and remote sensing technologies, to further enhance the precision and scope of flood predictions.
A Foundation for Future Innovations
This research marks a significant step forward in the use of AI for disaster risk reduction in Africa, particularly in addressing the growing threat of floods exacerbated by climate change. By demonstrating the effectiveness of AI models in predicting floods with high accuracy, the study provides a valuable foundation for future efforts to improve disaster preparedness and resilience in vulnerable regions. The findings suggest that with continued innovation and investment in AI technologies, it will be possible to develop more robust and reliable flood forecasting systems that can save lives and protect communities from the devastating impacts of natural disasters.
- FIRST PUBLISHED IN:
- Devdiscourse