The digital mirror: Reflecting mental health through social media
The research moves beyond social media as a standalone data source by integrating it with other behavioral and physiological signals, such as wearable sensors and smartphone data. This multimodal approach allows for a more comprehensive understanding of mental health. For instance, combining social media data with physical activity metrics and location tracking significantly improved the prediction of mood, stress, and anxiety levels.
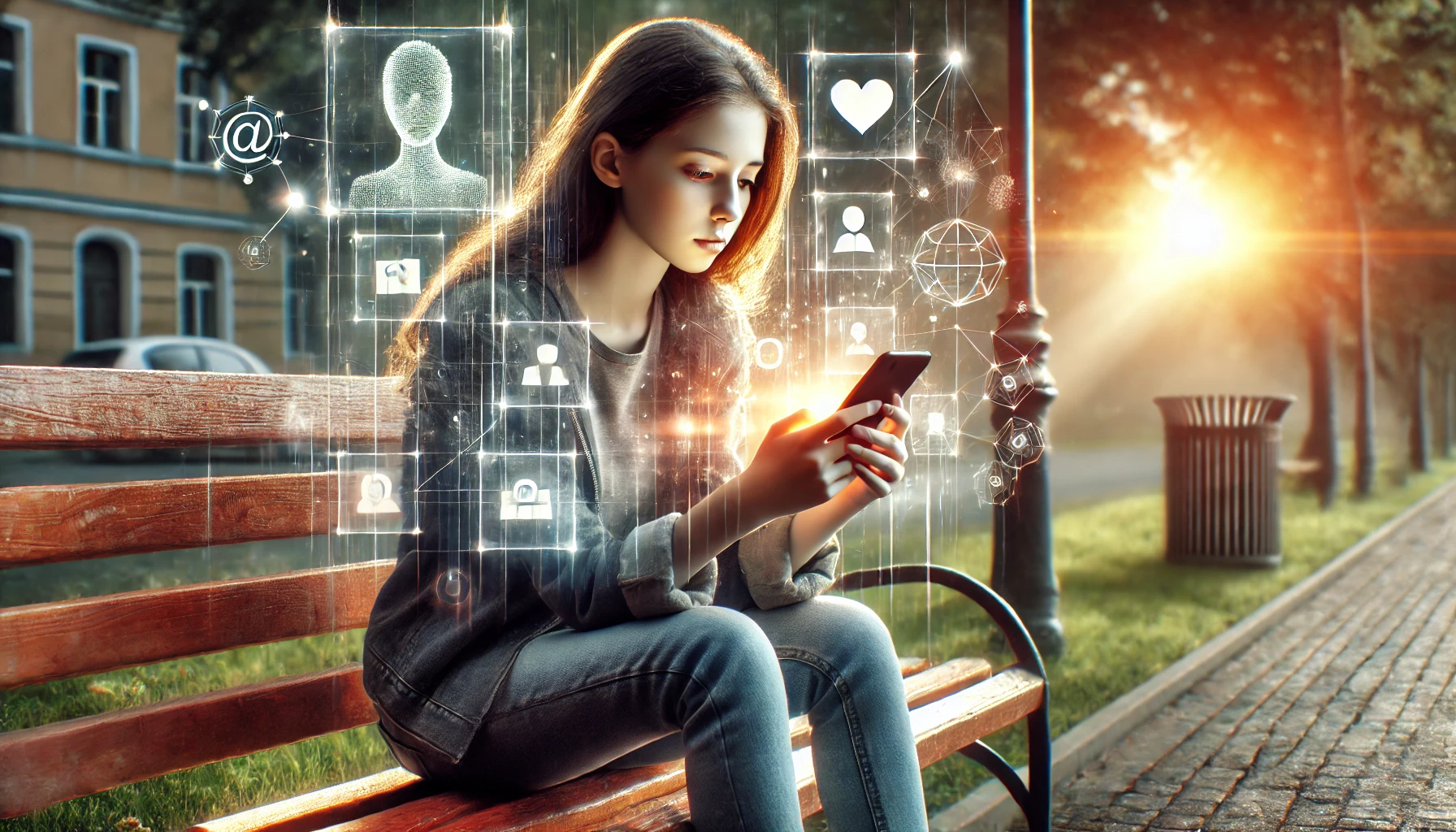
Mental health issues impact millions globally, often leading to severe repercussions in personal and professional spheres. Traditional methods of addressing mental health challenges - ranging from self-reported surveys to clinical interviews - are limited by gaps in accessibility, timeliness, and coverage. In recent years, social media has emerged as a powerful tool for understanding and addressing mental health issues due to the real-time and candid nature of data shared by users.
Munmun De Choudhury’s chapter, "Employing Social Media to Improve Mental Health Outcomes, set to appear in the Handbook of Computational Social Science," Edward Elgar Publishing Ltd., explores the integration of computational tools and social media data to enhance mental health research and interventions. This groundbreaking work examines how predictive models can detect mental health risks, evaluates the ethical considerations involved, and provides a roadmap for leveraging social media as a transformative tool in mental health care.
The power of social media in mental health research
Social media platforms provide a unique opportunity to study mental health through the lens of naturalistic and longitudinal data. Unlike traditional self-reports, which are often retrospective and constrained by time, social media captures real-time expressions of thoughts, emotions, and behaviors. De Choudhury’s research highlights how patterns in language, tone, and engagement can reveal critical insights into mental health states. For instance, studies cited in this chapter have successfully linked linguistic markers such as increased use of first-person pronouns, negative sentiment, and self-referential language to conditions like postpartum depression, schizophrenia, and bipolar disorder.
One notable study leveraged Twitter data to predict the onset of depression with over 71% accuracy by analyzing individuals’ tweets from the prenatal period. Another project employed Reddit to assess acute stress levels following campus gun violence incidents, demonstrating the feasibility of using social media to monitor stress responses in crisis settings. These examples underscore the potential of social media to function as a "verbal sensor," providing actionable insights into mental health.
Challenges and validation of predictive algorithms
While the promise of social media-based mental health models is immense, their real-world application requires rigorous validation. De Choudhury emphasizes that many existing models rely on behavioral proxies rather than clinically validated data, leading to gaps in their psychometric validity. To address this, the research highlights the need for deeper collaborations between computational scientists and clinicians. By integrating social media data with clinical assessments, researchers can develop more accurate models that align with real-world diagnostic standards.
Moreover, the chapter underscores the importance of addressing biases inherent in social media datasets. For example, demographic and platform-specific biases can skew results, limiting the generalizability of predictive models. To overcome these challenges, the research advocates for the creation of benchmark datasets, annotated with clinical information and collected through voluntary consent.
The research moves beyond social media as a standalone data source by integrating it with other behavioral and physiological signals, such as wearable sensors and smartphone data. This multimodal approach allows for a more comprehensive understanding of mental health. For instance, combining social media data with physical activity metrics and location tracking significantly improved the prediction of mood, stress, and anxiety levels.
The chapter also highlights the benefits of personalized machine learning models. By clustering individuals based on their behavioral patterns and building tailored algorithms for each group, researchers achieved a 4–78% improvement in predictive accuracy. These findings reinforce the need for personalized and context-aware models in mental health research.
Exploring causal relationships
Understanding causality is a critical aspect of mental health research, and social media offers a unique platform for studying it. De Choudhury’s work has shown how linguistic shifts on Reddit can predict transitions to suicidal ideation. For instance, heightened self-attentional focus, reduced social engagement, and expressions of hopelessness were identified as early warning signs. By isolating these factors from confounding variables, researchers could build models with an 81% accuracy in predicting suicidal thoughts.
In addition to identifying risk factors, the research explores protective elements such as social support. Analyzing supportive language in mental health communities revealed that esteem and network support significantly reduced the risk of suicidal ideation. These findings pave the way for designing interventions that amplify protective factors in online interactions.
Participatory design and real-world implementation
A recurring theme in De Choudhury’s research is the importance of stakeholder involvement in designing mental health algorithms. By collaborating with clinicians, patients, and public health officials, the research ensures that these tools are ethically grounded and practically useful. For example, a project in partnership with Northwell Health used Facebook data to predict psychotic relapse 30 days in advance with 79% accuracy. These findings were integrated into clinician-facing tools to enhance decision-making and patient care.
Another collaboration with the Centers for Disease Control and Prevention (CDC) developed a machine learning model to estimate weekly suicide rates in near real-time. By combining data from social media, economic indicators, and health services, the model outperformed traditional approaches, reducing annual error margins by nearly 90%.
Ethical considerations
The use of social media in mental health research raises complex ethical issues. Concerns about privacy, consent, and the potential misuse of data are central to this discourse. De Choudhury emphasizes the need for transparent and participatory research practices that respect user autonomy and minimize harm. The chapter also calls for the development of guidelines and best practices for handling sensitive mental health data, ensuring that the benefits of these tools outweigh the risks.
- FIRST PUBLISHED IN:
- Devdiscourse
ALSO READ
Sports Roundup: Mental Health, Trades, and Celebrated Debuts
How COVID-19 Exposed and Strained the Mental Health of Healthcare Professionals
Tragic Pattern: Second Student Suicide in Kota Highlights Mental Health Crisis
Transforming mental health: Machine learning paves the way for predictive care
Adani Vidya Mandir Pioneers India's First Mental Health Education Program