When every second counts: AI’s game-changing role in disaster management
The incorporation of IoT devices and sensors offers another layer of precision. Ground-based sensors measuring rainfall intensity and water levels can complement satellite data, creating an interconnected network for disaster monitoring. Social media and crowd-sourced data also hold potential for enhancing situational awareness, particularly in urban areas where flooding patterns are complex and dynamic.
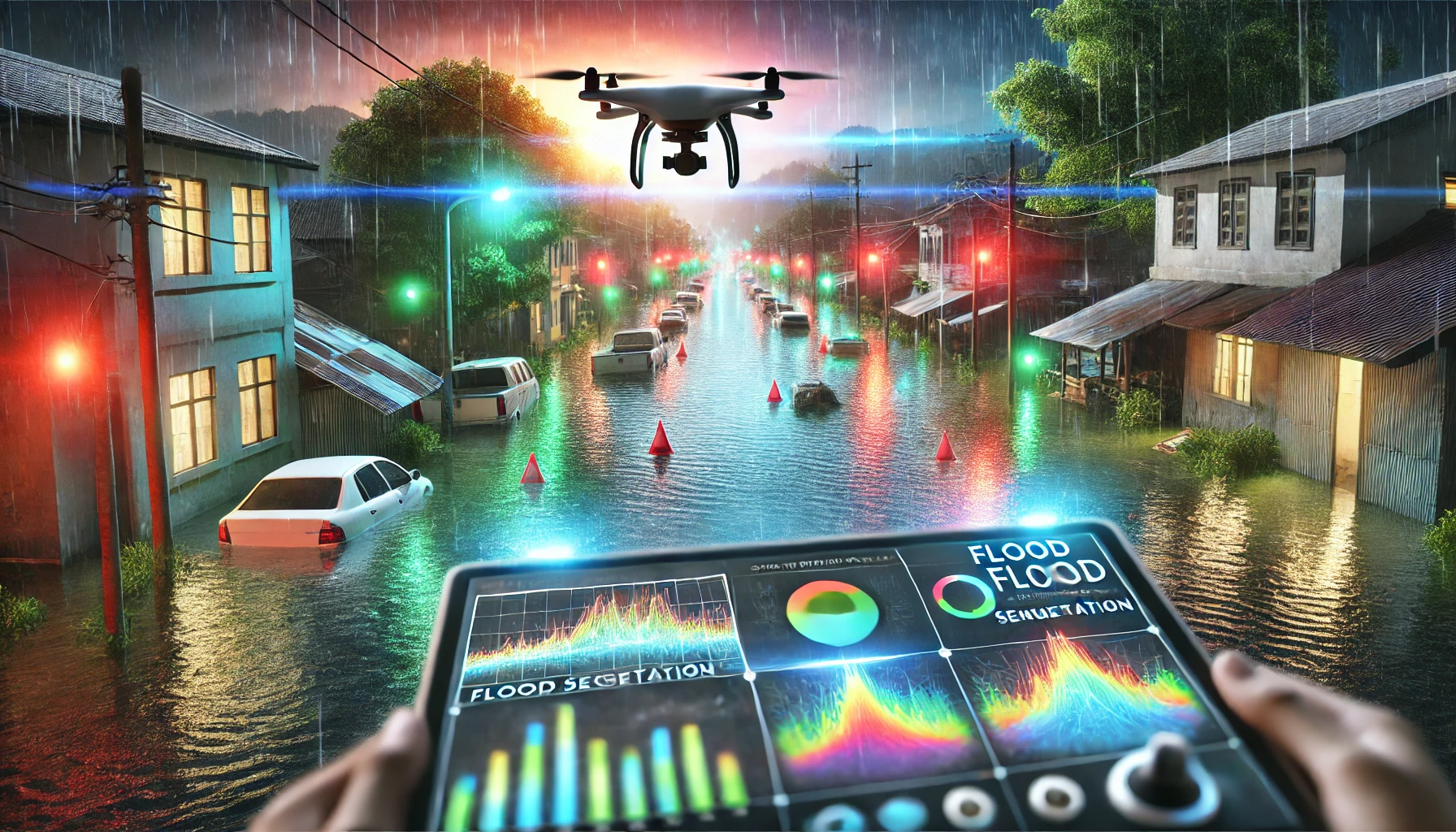
Floods rank among the most catastrophic natural disasters, inflicting substantial damage to infrastructure, disrupting livelihoods, and resulting in significant loss of life. With climate change intensifying the frequency and severity of flooding, rapid and accurate detection has become imperative for mitigating its impacts. Traditionally, flood monitoring relied on manual observation or semi-automated processes, which, although effective to some extent, fall short of the speed and precision required for timely interventions.
Recognizing these limitations, the study Effectiveness of Machine Learning Methods for Water Segmentation with ROI as the Label: A Case Study of the Tuul River in Mongolia by Sanjida Afrin Mou et al., submitted on arXiv, investigates the transformative potential of deep learning models in flood detection. By comparing the performance of three architectures - U-Net, ResNet-50, and DeepLab v3 - the study sets a benchmark for leveraging artificial intelligence to address real-world challenges in disaster management.
Flood monitoring: Challenges and opportunities
Flood detection and monitoring present complex challenges, particularly in environments characterized by variable topographies and diverse environmental conditions. Existing methodologies often rely on time-intensive manual processes or semi-automated techniques, which are prone to errors and constrained by scalability. These systems frequently struggle to incorporate diverse data sources such as drone imagery, social media content, and satellite data, limiting their applicability in regions with distinct flood patterns like the Tuul River Basin. However, advancements in deep learning have opened new avenues for addressing these challenges. By automating water segmentation, deep learning models reduce human intervention, accelerate response times, and enhance accuracy. They also integrate seamlessly with new technologies such as Internet of Things (IoT) sensors, drones, and CubeSats, making flood detection more robust and adaptive.
The research utilized a comprehensive dataset of 290 high-resolution images representing flood-affected areas alongside binary mask images denoting water regions. To ensure robustness, the dataset incorporated diverse scenarios, including riverine floods, flash floods, and coastal flooding, captured across varying geographical and environmental conditions. Data augmentation techniques such as rotation, flipping, and scaling enhanced the dataset’s variability, allowing the models to generalize effectively across different scenarios. Three deep learning architectures were selected for this study.
DeepLab v3, a state-of-the-art model, leverages atrous convolution for multi-scale context capture, making it highly effective for semantic segmentation. U-Net is known for its U-shaped architecture, which combines contracting and expanding paths for precise localization and segmentation. ResNet-50, a deep residual network, is designed to mitigate vanishing gradient issues and improve feature extraction for accurate predictions. The models were trained using optimized parameters, including early stopping and Adam optimization, to achieve convergence without overfitting. Performance metrics such as accuracy, precision, recall, and F1-score provided a multi-dimensional assessment of their effectiveness.
Results and comparative analysis
The performance of the three models highlighted significant variations in their capabilities for flood detection. DeepLab v3 emerged as the most effective, achieving a validation accuracy of 90.57%. Its ability to capture multi-scale features enabled superior segmentation, especially in distinguishing water bodies from complex backgrounds such as vegetation or urban structures.
Despite its slightly higher validation loss compared to U-Net, its overall accuracy and precision underscored its reliability for real-world applications. ResNet-50 achieved a balanced performance with an accuracy of 88.7%. Its residual learning approach allowed it to maintain stability during training, making it a strong contender for flood segmentation tasks. ResNet’s F1-score of 85.1% reflected its robustness in identifying water regions under varying conditions.
U-Net, while demonstrating the lowest validation loss, had an accuracy of 87.12%, indicating potential challenges in generalizing across diverse environments. Despite its limitations, U-Net’s architecture remains valuable for scenarios requiring fine-grained segmentation. The study’s findings underscore the advantages of using advanced deep learning models like DeepLab v3 for flood detection. By automating the segmentation process, these models reduce the time required to generate flood maps, enabling faster and more informed decision-making during emergencies.
Broader implications for disaster management
The implications of this research extend beyond the technical evaluation of deep learning models. By streamlining flood detection, these methodologies pave the way for more effective disaster preparedness and response strategies. Automated segmentation models significantly reduce manual processing times, allowing emergency response teams to allocate resources more efficiently. Additionally, the integration of diverse data sources - ranging from satellite imagery to crowd-sourced social media content - enhances the adaptability and accuracy of these systems.
The study also highlights the importance of scalability in flood detection technologies. Models like DeepLab v3 can be integrated with low-cost satellites such as CubeSats, enabling monitoring in remote or resource-constrained areas. The ability to process data in real-time further strengthens their utility, offering early warnings to communities at risk and facilitating timely evacuations.
Future directions and innovations
While the study provides a robust foundation for flood detection, it also identifies areas for future research and development. One promising avenue is the integration of multi-modal data sources, combining optical, radar, and thermal imaging to overcome limitations like cloud cover during storms. For instance, radar imagery’s ability to penetrate clouds complements the high spatial resolution of optical data, creating a comprehensive picture of flood dynamics.
The development of hybrid architectures tailored specifically for flood detection tasks is another area of interest. By combining the strengths of models like U-Net and DeepLab v3, researchers can create systems optimized for both accuracy and efficiency. Additionally, advances in computational infrastructure, such as edge computing and cloud-based platforms, could further reduce processing times, enabling real-time monitoring and analysis.
The incorporation of IoT devices and sensors offers another layer of precision. Ground-based sensors measuring rainfall intensity and water levels can complement satellite data, creating an interconnected network for disaster monitoring. Social media and crowd-sourced data also hold potential for enhancing situational awareness, particularly in urban areas where flooding patterns are complex and dynamic.
- FIRST PUBLISHED IN:
- Devdiscourse
ALSO READ
India has built its disaster management capacities due to advances in meteorological sciences, world has also benefited from it: PM Modi.
Piyush Goyal Highlights India's Leadership in Disaster Relief at World Congress on Disaster Management
India Champions Disaster Management for Global South, Sets Example with Proactive Approach
Jamaica’s Gender Divide in Disaster Management: Challenges and Solutions
India has emerged as global leader in disaster management, says Amit Shah.