Transforming mental health: Machine learning paves the way for predictive care
By predicting transitions in diagnosis, healthcare providers can identify high-risk patients early and tailor interventions to prevent the escalation of mental health problems. This predictive capability can also improve the allocation of healthcare resources, directing attention to patients most in need and reducing the burden on overstrained systems.
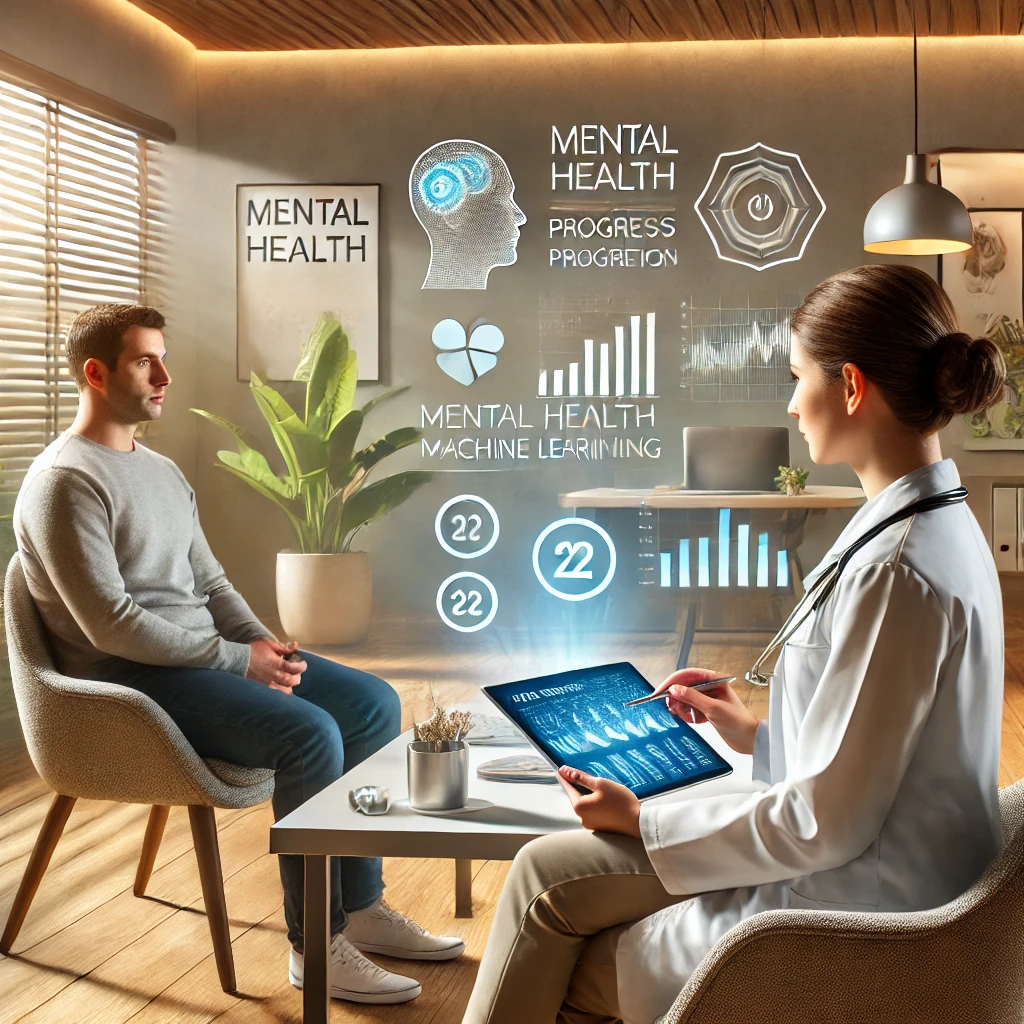
Mental health remains a critical challenge in global healthcare, with addiction and its interplay with other psychological conditions at the forefront of this complex landscape. Advances in machine learning and artificial intelligence are offering novel tools to unravel the intricate relationships between addiction and mental illness, as highlighted by the study titled "Machine Learning for Mental Health: Predicting Transitions from Addiction to Illness." Published in the IAES International Journal of Artificial Intelligence (IJ-AI), this study by Ali Alkhazraji, Fatima Alsafi, Mohamed Dbouk, Zein Al Abidin Ibrahim, and Ihab Sbeity delves into predicting the progression of patients with addiction to other psychiatric conditions. Leveraging real-world data from the Ibn Roshd Hospital for Mental Illness and Addiction Treatment in Baghdad, Iraq, the researchers propose a robust machine learning framework that could transform the diagnosis and treatment of addiction-related mental health issues.
Understanding the data and predictive framework
The study recognizes addiction as a multifaceted condition, often serving as a gateway to other mental health challenges. By examining patient profiles and their medical histories, the researchers sought to predict these transitions and identify patterns that could inform more targeted interventions. The dataset, derived from patient records at the Iraqi hospital, included demographic details, medical history, lab tests, treatments, and diagnoses. However, handling this data posed significant challenges, such as missing values and unstructured textual information. To overcome these hurdles, the team employed advanced preprocessing techniques, including data normalization, handling missing values, and converting text-based medical records into numerical formats using state-of-the-art natural language processing (NLP) tools like BioBERT and BioALBERT.
A key strength of the study lies in its dual approach to data organization. One method aggregated all visits of a patient into a single record, providing a holistic view of their medical journey. The other retained details of individual visits, capturing temporal variations and contextual nuances. This second approach proved particularly effective, as it allowed the researchers to account for the progression of addiction and its transformation into other conditions over time. The dataset, which initially suffered from significant class imbalances, was balanced to ensure that machine learning models could learn effectively across all classes, reducing bias toward more frequently occurring diagnoses.
The research team explored multiple machine learning models to predict diagnosis transitions, with gradient boosting emerging as the most accurate and reliable classifier. Gradient boosting's ability to handle complex relationships between features and outcomes made it particularly well-suited for this task. The study found that using visit-level data significantly improved model performance, underlining the importance of capturing detailed, time-specific information in medical datasets. Among the NLP models, BioALBERT demonstrated slightly better performance than BioBERT when applied to visit-level data, though both models achieved impressive results in extracting meaningful features from textual patient records.
Implications for mental health care
This work has profound implications for mental health care, particularly in addressing addiction's role as a precursor to broader psychological issues. By predicting transitions in diagnosis, healthcare providers can identify high-risk patients early and tailor interventions to prevent the escalation of mental health problems. This predictive capability can also improve the allocation of healthcare resources, directing attention to patients most in need and reducing the burden on overstrained systems. Additionally, understanding the factors that drive these transitions opens the door to more personalized treatment protocols, helping patients navigate their recovery journeys with greater support and precision.
Despite its promise, the study acknowledges certain limitations. The dataset's relatively small size may have constrained the models' robustness, and the static nature of the data does not fully capture the dynamic, evolving trajectories of mental health conditions. Future work could address these gaps by incorporating larger, longitudinal datasets and time-series analysis to track patient outcomes over extended periods. Moreover, including additional demographic, environmental, and behavioral variables could provide a more comprehensive picture of the interplay between addiction and mental health.
As addiction and mental health issues continue to rise globally, such innovative approaches are urgently needed to enhance diagnosis, treatment, and prevention.
- FIRST PUBLISHED IN:
- Devdiscourse
ALSO READ
The Crucial Connection: Sleep, Memory, and Mental Health
Global Health Watch: China's Mental Health Initiative and Pharma Highlights
CISF's Game-Changing Mental Health Initiatives Slash Suicide Rates
China's Growing Focus on Mental Health: A Nationwide Initiative
AI and empathy: Transforming mental health care with novel approach