Revolutionary AI tool identifies ideal candidates for clinical trials
TrialTranslator represents a significant leap forward in addressing the limitations of traditional clinical trial generalizability. The framework employs machine learning models to stratify patients into low-risk, medium-risk, and high-risk phenotypes based on their predicted mortality risks.
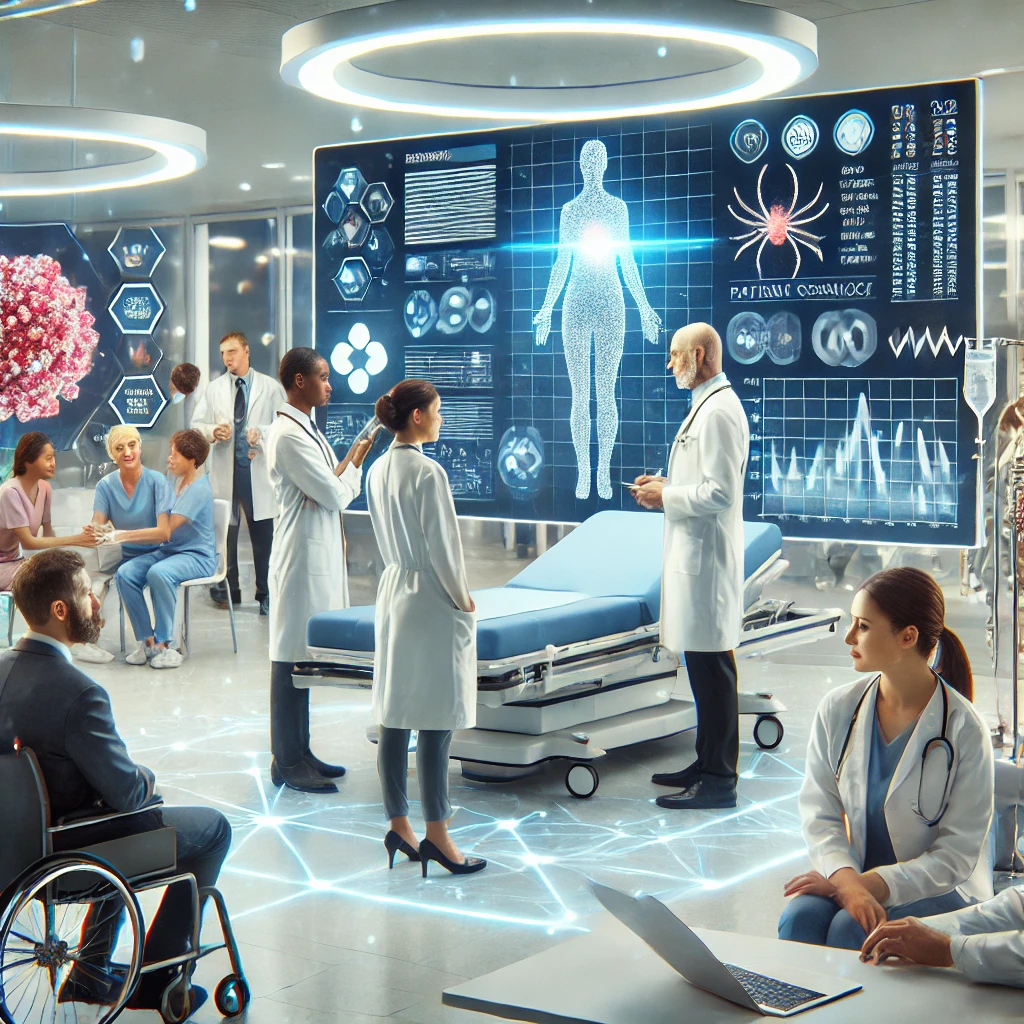
Randomized controlled trials (RCTs) have long been considered the cornerstone of medical research, particularly in oncology, where novel therapies are rigorously evaluated for their efficacy and safety. However, the findings of these trials often fall short in applicability to real-world patients due to highly selective eligibility criteria and a lack of representation of patient diversity.
The study “Evaluating Generalizability of Oncology Trial Results to Real-World Patients Using Machine Learning-Based Trial Emulations”, published in Nature Medicine by Xavier Orcutt, Kan Chen, Ronac Mamtani, Qi Long, and Ravi B. Parikh, seeks to address this fundamental issue. Through the introduction of the TrialTranslator framework, the authors present a machine learning-driven approach to emulate RCT conditions for broader patient populations, ultimately providing insights into the varied efficacy of treatments across real-world clinical settings.
A revolutionary AI framework for clinical trials
The disconnect between RCT outcomes and real-world observations has been a persistent challenge in oncology. Real-world patients, often older and burdened with comorbidities, experience markedly different outcomes compared to their counterparts in tightly controlled clinical trials. For instance, studies have reported median overall survival reductions of up to six months in real-world settings when compared to trial outcomes. While eligibility criteria are frequently blamed for this gap, the study delves deeper into the role of patient prognostic heterogeneity. Using the Flatiron Health database, a robust repository of electronic health records (EHRs) from over 280 U.S.-based oncology clinics, the authors demonstrate that discrepancies in survival outcomes are influenced not only by eligibility filters but also by the intrinsic characteristics of patients, such as their risk profiles and comorbid conditions.
TrialTranslator represents a significant leap forward in addressing the limitations of traditional clinical trial generalizability. The framework employs machine learning models to stratify patients into low-risk, medium-risk, and high-risk phenotypes based on their predicted mortality risks. By integrating real-world patient data with the eligibility criteria of landmark phase 3 oncology trials, TrialTranslator systematically emulates trial conditions and evaluates how well RCT results hold across diverse prognostic groups. Unlike conventional approaches, this method allows for a more granular understanding of treatment effects, shedding light on the nuanced ways therapies benefit - or fail to benefit - patients with varying clinical profiles.
The workflow begins with the development of cancer-specific prognostic models, including gradient boosting machines (GBMs), which are trained to predict overall survival using features such as age, weight loss, performance status, and biomarkers. These models are then applied to select real-world patients who meet trial eligibility criteria, after which patients are categorized into risk phenotypes. The final step involves survival analysis, which compares treatment-associated survival benefits among these phenotypes and with the original RCT findings. This innovative process not only highlights the limitations of RCT generalizability but also offers actionable insights for clinicians managing diverse patient populations.
The findings of the study underscore the critical impact of prognostic heterogeneity on the applicability of RCT results. Patients in the low- and medium-risk groups often exhibit survival outcomes and treatment benefits similar to those reported in trials. In stark contrast, high-risk patients consistently show significantly worse survival outcomes and reduced treatment efficacy. For instance, the median overall survival for high-risk patients was, on average, 62% lower than the survival rates reported in the original trials. These results highlight a crucial insight: the generalizability gap is not merely a result of restrictive eligibility criteria but is also deeply tied to the prognostic diversity of real-world patients.
The study also revealed that high-risk phenotypes are less likely to derive meaningful survival benefits from treatments. In more than half of the emulated trials, the survival benefit for high-risk patients was less than three months—a threshold often considered clinically insignificant in oncology. This finding suggests a pressing need for trials to better account for high-risk populations, either through more inclusive eligibility criteria or by designing trials specifically targeted at these patients.
Implications for Oncology practice
The implications of this research extend beyond academic inquiry, offering tangible benefits for clinical practice. TrialTranslator enables oncologists to make more informed decisions by providing survival predictions tailored to individual patient profiles. For example, by stratifying patients into risk phenotypes, clinicians can better assess whether a therapy proven effective in trials will yield similar results for a particular patient. This personalized approach not only improves treatment planning but also helps set realistic expectations for patients and their families.
Moreover, the study calls for a reevaluation of how RCTs are designed. Traditional eligibility criteria often exclude high-risk patients, resulting in a skewed representation of the oncology population. By incorporating tools like TrialTranslator, trial designers can ensure broader representation, making results more applicable to real-world settings. This shift is particularly crucial for emerging therapies, such as immune checkpoint inhibitors, where the delayed treatment effects often observed in trials may not align with the immediate needs of high-risk patients.
Challenges
While TrialTranslator marks a significant step forward, the study acknowledges several limitations and areas for improvement. The lack of external validation across diverse datasets raises questions about the generalizability of the framework itself. Additionally, the reliance on EHR data, while advantageous for its breadth, may introduce biases due to missing or unstructured information. Future iterations of the framework could address these challenges by incorporating more comprehensive datasets and validating models in international cohorts.
The study also highlights the importance of addressing structural inequities in trial participation. Factors such as socioeconomic status and race, which are often linked to prognosis, must be considered in trial design to ensure equitable representation. Furthermore, as the field of oncology continues to evolve, integrating genomic and molecular data into frameworks like TrialTranslator could provide even deeper insights into the individualized effects of therapies.
- FIRST PUBLISHED IN:
- Devdiscourse