Transforming FDA Clearance: How AI and Human Insight Can Improve Medical Device Safety
Researchers from Indiana University, Harvard Kennedy School, and Emerging Health Consulting propose a combined human-algorithm approach to enhance the FDA's 510(K) medical device clearance process, demonstrating a 38.9% improvement in recall rates and significant cost savings. Their method leverages machine learning to estimate recall risks, aiming to streamline approvals and reduce FDA workload.
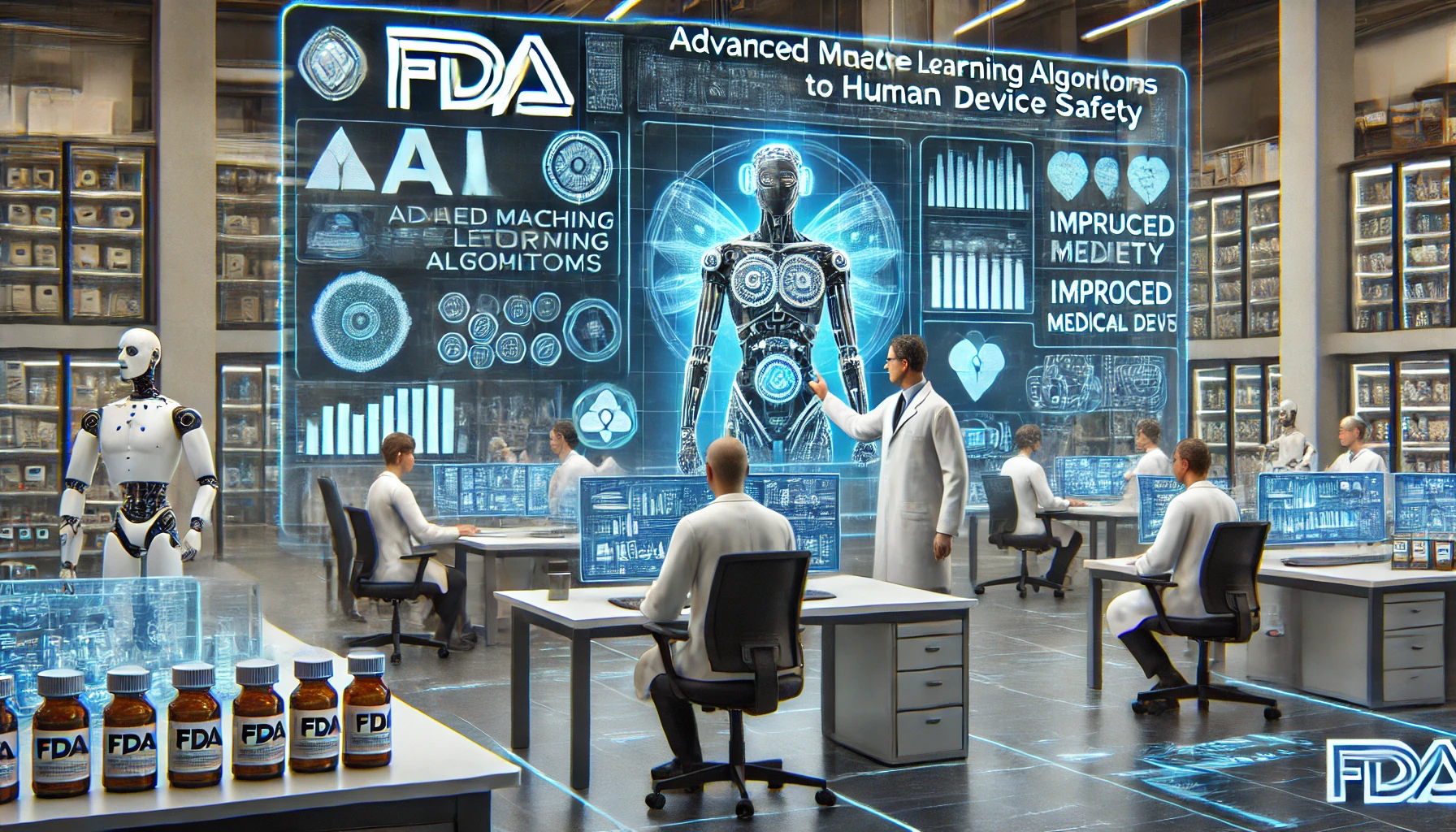
The FDA's Premarket Notification 510(K) pathway has faced increasing scrutiny due to its high recall rates for cleared medical devices. This process allows manufacturers to gain approval by demonstrating substantial equivalence to legally marketed devices, but its inherent ambiguity has raised significant concerns about its efficacy. Researchers Mohammad Zhalechian from Indiana University's Kelley School of Business, Soroush Saghafian from Harvard Kennedy School, and Omar Robles from Emerging Health Consulting have proposed a combined human-algorithm approach to enhance the 510(K) clearance process. They leverage machine learning to estimate recall risks based on submission data, aiming to recommend devices for acceptance, rejection, or deferral for in-depth evaluation. This method could potentially reduce recalls and FDA workload significantly. Their empirical study, which analyzed over 31,000 devices and 12,000 manufacturers, demonstrated a 38.9% improvement in recall rates and a 43% reduction in FDA workload, along with substantial annual cost savings ranging from 2.4 billion dollars to 2.7 billion dollars.
A Pathway Under Fire: Scrutiny on FDA's 510(K) Process
The 510(K) pathway was originally designed to ease the burden on manufacturers bringing medium-to-low risk devices to market, requiring them to demonstrate that a new device is substantially equivalent to a predicate device. However, this approach has been criticized for its focus on equivalence rather than safety. Studies have shown that a high percentage of devices cleared through this pathway have been recalled due to safety concerns. For instance, between 2005 and 2009, 71% of devices recalled by the FDA had initially been cleared through the 510(K) pathway. More recent analyses indicate that 11% of devices cleared via the 510(K) pathway between 2003 and 2018 were later subject to Class I or II recalls. Despite these issues, there has been a lack of evidence-based understanding on how the FDA can improve the 510(K) pathway. This gap is evident from the FDA's recent solicitations for feedback and its 2018 Medical Device Safety Action Plan, which aims to modernize the 510(K) pathway.
Leveraging Data-Driven Solutions for Improved Safety
The researchers' primary objective is to develop a data-driven approach that can assist the FDA in improving the 510(K) pathway. They posit that developing a highly accurate predictive model of recall risk can enable the FDA to pinpoint devices that might require more rigorous examination or necessitate stronger evidential support before approval. Such a model requires access to extensive data on both applicant devices and predicates, along with detailed data engineering processes to generate useful insights. The researchers collected and assembled a large-scale dataset from FDA and CMS sources, involving information on over 31,000 medical devices and 12,000 manufacturers. They used this data to develop machine learning models capable of estimating the risk of recall based on the information available at the time of submission. Their best-performing model achieved a cross-validation Area Under the Curve (AUC) score of 0.77.
Balancing Efficiency with Safety: A Two-Step Policy Approach
The proposed policy involves a two-step process. In the first step, the researchers collect and process FDA data to train machine learning models. These models predict the recall risk of an applicant device using available submission data. The second step involves developing a clearance policy that uses these predictions to recommend whether a device should be accepted, rejected, or deferred for further evaluation by human experts. This policy aims to balance precision in decision-making with the workload constraints of the FDA, ensuring that only devices with low predicted recall risks are accepted without further review. Devices with high predicted recall risks are recommended for rejection, while those with intermediate risks are deferred for detailed evaluation by FDA committees. This approach leverages the predictive power of machine learning while incorporating human judgment for complex cases.
Empirical Success: Demonstrated Improvements and Savings
The empirical results of the study indicate that implementing this policy could lead to substantial improvements in the FDA's 510(K) evaluation process. The policy not only reduces the recall rate by 38.9% but also decreases the FDA's workload by 43%, allowing for more efficient use of resources. Additionally, the estimated annual cost savings from implementing this policy range between 2.4 billion dollars and 2.7 billion dollars, highlighting the economic benefits of a data-driven approach. The researchers' findings underscore the importance of integrating machine learning with human expertise to address the limitations of the current 510(K) pathway. By combining algorithmic predictions with human oversight, the proposed policy ensures more accurate and efficient evaluations, ultimately enhancing public health outcomes by reducing the risk of unsafe medical devices entering the market.
A Holistic Approach for Future Regulatory Frameworks
This approach addresses the main concerns raised by critics of the 510(K) process and aligns with the FDA's goals of establishing best practices for evaluating the safety of medical devices. The research provides valuable insights for regulatory frameworks and demonstrates the potential of a holistic, data-driven approach in improving the safety and effectiveness of medical device evaluations.
- FIRST PUBLISHED IN:
- Devdiscourse
ALSO READ
FDA Lifts Hold on BioNTech's Experimental Cancer Drug Study
Granules India Secures USFDA Nod for Generic Glycopyrrolate Oral Solution
USFDA Flags Lapses at Eugia Pharma’s Hyderabad Plant
Alembic Pharmaceuticals Receives USFDA Nod for Psoriasis Treatment
Health Headlines: FDA Expands MDMA Drug Probe, WHO Appeals for $135M to Curb Mpox Outbreak