Plugging the gaps in energy data: AI-driven solutions for smarter grids
The intersection of AI and energy analytics holds immense promise, and this research represents a significant step forward. By bridging data gaps with precision and scalability, the energy sector can harness the full potential of smart grids, driving sustainability and innovation in equal measure.
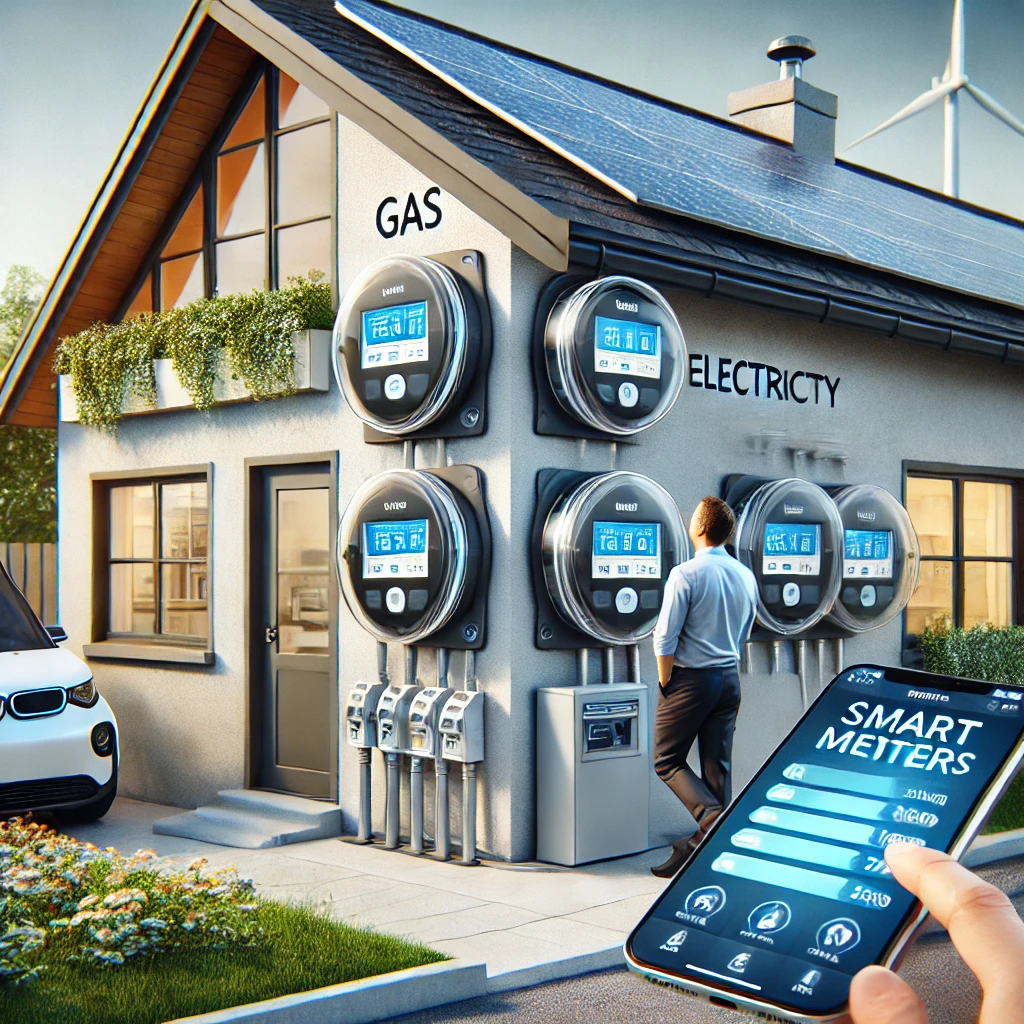
The transition to smart grids has revolutionized energy systems, with smart meters (SMs) providing granular, real-time data on energy consumption. However, as the reliance on these systems increases, the issue of data quality becomes critical. Missing data caused by sensor failures, transmission errors, or external disruptions not only impairs the functionality of predictive models but also affects decision-making processes in the energy sector.
A newly published study on arXiv, "Bridging Smart Meter Gaps: A Benchmark of Statistical, Machine Learning and Time Series Foundation Models for Data Imputation" by Amir Sartipi, Joaquin Delgado Fernandez, Sergio Potenciano Menci, and Alessio Magitteri, addresses this challenge head-on. The researchers explore the potential of advanced machine learning and time series models to enhance data imputation and maintain the integrity of smart meter analytics.
Data gaps and the imputation challenge
Data gaps in smart meters manifest as incomplete time series, complicating tasks such as load forecasting, anomaly detection, and grid stability analysis. Traditional gap-filling methods, often employed in industry, rely on simplistic techniques like linear interpolation or replicating the last observed value. These methods, while computationally inexpensive, fail to capture the nonlinear and nonstationary patterns typical of energy consumption data.
The study underlines the pivotal role of accurate gap-filling, especially as smart meter datasets grow in complexity. Inefficiencies in data imputation can lead to inaccurate billing, poor energy management decisions, and diminished grid reliability. This underscores the urgency of adopting more sophisticated techniques capable of addressing diverse and dynamic datasets.
Evaluating cutting-edge imputation models
The research evaluates a broad spectrum of models for gap-filling, categorized into traditional statistical methods, machine learning (ML) models, and emerging Time Series Foundation Models (TSFMs). The dataset used includes energy consumption data collected in London, anonymized and enriched with artificial gaps to simulate real-world challenges. The researchers benchmarked the models using five evaluation metrics, including Mean Absolute Error (MAE) and Root Mean Squared Error (RMSE), ensuring a robust comparison of their predictive performance.
Among statistical methods, the Holt-Winters model demonstrated superior performance, adeptly capturing seasonal and trend patterns in the data. ML models like Random Forest and LightGBM also showed strong results, with Random Forest emerging as the most reliable due to its ability to model intricate patterns and handle noisy datasets.
However, it is the TSFMs that steal the spotlight. Models like Time-MoE and Chronos-T5, leveraging advanced architectures like sparse Mixture-of-Experts and transformer-based frameworks, outperformed their counterparts. Time-MoE, in particular, demonstrated exceptional accuracy and stability, indicating its potential as a benchmark for future imputation tasks
Pre-trained TSFMs present a paradigm shift in time series analysis. Unlike traditional models that require extensive feature engineering and parameter tuning, TSFMs leverage vast datasets and state-of-the-art architectures to generalize across diverse applications. In the study, these models delivered superior results even without fine-tuning, highlighting their scalability and efficiency.
The comparison also reveals a trade-off between computational cost and performance. While models like Time-MoE demand higher computational resources, their accuracy and adaptability make them a worthwhile investment for critical applications. Conversely, simpler models may suffice in scenarios where computational efficiency is prioritized over precision.
Implications for the energy sector
The findings of this research carry significant implications for the energy sector, where smart grids and predictive analytics play a crucial role. Accurate imputation enhances the reliability of predictive models, improving load management, anomaly detection, and overall grid stability. By addressing data gaps with advanced methods like Time Series Foundation Models (TSFMs), energy providers can ensure operational efficiency and resilience, even in the face of disruptions. These models also support accurate billing, fostering customer trust and satisfaction, while enabling tailored services such as personalized energy-saving recommendations.
Additionally, the study highlights the importance of establishing standardized benchmarking frameworks for evaluating imputation models. Consistent metrics and datasets are essential to advancing the state of the art and ensuring fair comparisons across techniques. Such standardization will not only help identify the most effective approaches but also encourage collaboration between researchers and industry players, driving innovation and creating scalable solutions for sustainable and reliable energy systems. By adopting these advancements, the energy sector can achieve greater precision, efficiency, and resilience in managing smart grids
Towards robust and scalable solutions
The study paves the way for future research, emphasizing the potential of fine-tuning pre-trained models to specific datasets and exploring their performance on longer data gaps. Another promising direction involves optimizing computational efficiency, ensuring that even resource-constrained organizations can benefit from cutting-edge imputation techniques.
The intersection of AI and energy analytics holds immense promise, and this research represents a significant step forward. By bridging data gaps with precision and scalability, the energy sector can harness the full potential of smart grids, driving sustainability and innovation in equal measure.
- FIRST PUBLISHED IN:
- Devdiscourse