AI’s environmental awakening: Toward a greener future in technology
The training and inference stages of AI models are among the most energy-intensive processes in the AI lifecycle. Current hardware architectures, including GPUs and TPUs, struggle to meet the computational demands of increasingly complex models like GPT-3, which comprises 175 billion parameters. Memory bandwidth and latency further exacerbate these performance bottlenecks, leading to inefficiencies in resource utilization.
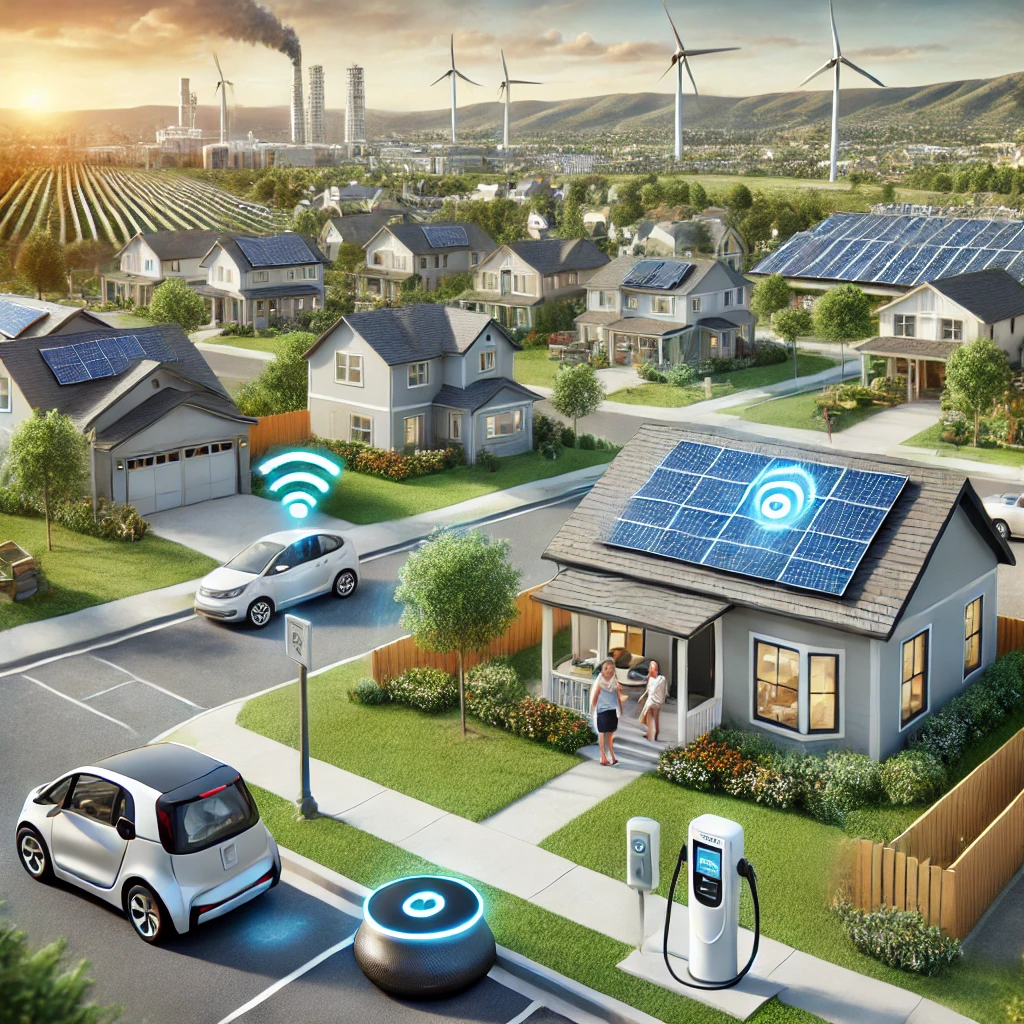
Artificial intelligence (AI) has emerged as a transformative force across industries, but its rapid growth has raised urgent concerns about its environmental and ethical impact. Training and deploying large-scale AI models require immense computational resources, contributing to rising energy consumption and carbon emissions. In their comprehensive study, "Data and System Perspectives of Sustainable Artificial Intelligence," Tao Xie et al. present a thorough examination of these challenges, focusing on sustainable practices in data acquisition, processing, and system optimization. This groundbreaking work provides a roadmap to mitigate environmental costs while advancing AI's capabilities responsibly.
A double-edged sword in AI development
Data acquisition forms the foundation of AI systems, yet its processes are fraught with challenges that undermine sustainability. One significant issue is the environmental footprint of collecting and processing large-scale datasets. Studies have highlighted that training a medium-sized AI model can produce carbon emissions equivalent to the lifetime emissions of five average cars. This environmental toll becomes more severe as datasets grow in size and complexity.
Moreover, public datasets often suffer from quality and transparency issues, such as bias and mislabeling, which propagate inaccuracies through AI systems. At the same time, valuable private data remains largely untapped due to privacy concerns, despite its potential to enhance model performance. The study also identifies non-textual data, such as acoustic and sensor data, as a largely underutilized resource that could provide critical insights across domains like healthcare, agriculture, and environmental monitoring.
To address these challenges, the authors propose innovative solutions such as crowdsourcing, active learning, and privacy-preserving techniques. Crowdsourcing platforms like Amazon Mechanical Turk enable scalable and cost-effective data collection but require quality control mechanisms like collaborative labeling and consensus strategies to ensure reliability. Active learning methods prioritize the most informative data samples for annotation, reducing the environmental cost of acquiring and processing vast amounts of data. Furthermore, federated learning and homomorphic encryption are highlighted as key technologies for utilizing private data without compromising user privacy.
Streamlining efficiency and reducing waste
Once data is collected, processing it efficiently is paramount for sustainable AI. Real-world datasets often contain noise, errors, and imbalances, leading to significant computational overhead and inefficiencies. For example, feature engineering - a critical step in transforming raw data into meaningful inputs - remains labor-intensive and domain-specific, limiting its scalability.
The study emphasizes the role of automation in addressing these inefficiencies. Tools like OpenRefine and Featuretools automate data cleaning and feature engineering, improving data quality while reducing manual effort. Intelligent data augmentation techniques, such as synthetic data generation and AutoAugment, create diverse and realistic datasets that mitigate data imbalances and enhance model robustness. For instance, synthetic data has been used in healthcare to train AI models on rare diseases, reducing the need for extensive real-world data collection.
The research also highlights the potential of advanced techniques like SMOTE (Synthetic Minority Over-sampling Technique) to generate realistic samples for underrepresented classes. By addressing imbalances in datasets, these approaches improve model fairness and accuracy while reducing computational redundancy.
Innovations in system efficiency
The training and inference stages of AI models are among the most energy-intensive processes in the AI lifecycle. Current hardware architectures, including GPUs and TPUs, struggle to meet the computational demands of increasingly complex models like GPT-3, which comprises 175 billion parameters. Memory bandwidth and latency further exacerbate these performance bottlenecks, leading to inefficiencies in resource utilization.
The study advocates for domain-specific architectures (DSAs) and RISC-V-based AI accelerators as transformative solutions. DSAs, such as Google’s Tensor Processing Units (TPUs), optimize hardware for specific AI tasks, significantly reducing computational redundancies and energy consumption. Similarly, RISC-V’s open-source architecture enables customization for specialized applications, enhancing computational density and energy efficiency.
Hardware-software co-optimization is another critical factor in improving system performance. TensorFlow’s XLA compiler, for example, generates hardware-specific machine code, ensuring efficient resource utilization. This synergy between hardware and software design can lead to substantial gains in energy efficiency and performance.
Sustainability through systemic innovations
The study highlights several systemic innovations that can drive sustainability in AI. Distributed and edge computing, for instance, reduces the reliance on centralized data centers by offloading tasks to local devices, thereby lowering energy usage. Additionally, blockchain technology offers a transparent framework for data traceability, ensuring data quality and provenance while reducing the risk of bias and misinformation.
The authors also explore the role of synthetic data and simulated environments in reducing the need for real-world data collection. In the autonomous vehicle industry, companies like Waymo and NVIDIA use synthetic data to train and test their AI models in diverse scenarios, cutting down on the costs and environmental impacts of physical testing.
Future challenges and the path forward
While the study offers promising solutions, it acknowledges several challenges that must be addressed to achieve sustainable AI. The scalability of AI systems presents a significant hurdle as models grow larger and more complex. Innovations in memory technology, such as HBM2 and DDR5, and advancements in low-precision computing are critical for balancing computational power with energy efficiency.
Legal and ethical considerations surrounding private data utilization require international collaboration to establish standardized frameworks that balance innovation with user privacy. Furthermore, the lack of unified programming models across diverse hardware architectures creates inefficiencies that hinder cross-platform optimization.
Finally, the authors call for the development of accessible tools and platforms that democratize sustainable AI practices. User-friendly interfaces, comprehensive documentation, and pre-built solutions can empower smaller organizations and researchers to adopt sustainable methodologies, driving innovation across the ecosystem.
- READ MORE ON:
- sustainable AI
- AI efficiency
- AI and sustainability
- AI bias
- FIRST PUBLISHED IN:
- Devdiscourse