Humans at the helm: Driving innovation and security in AI development
While automation is a valuable tool for scaling red teaming efforts, it cannot replace human judgment and creativity. Subject matter experts (SMEs) are indispensable in evaluating complex or domain-specific risks, such as those involving medical or cultural nuances.
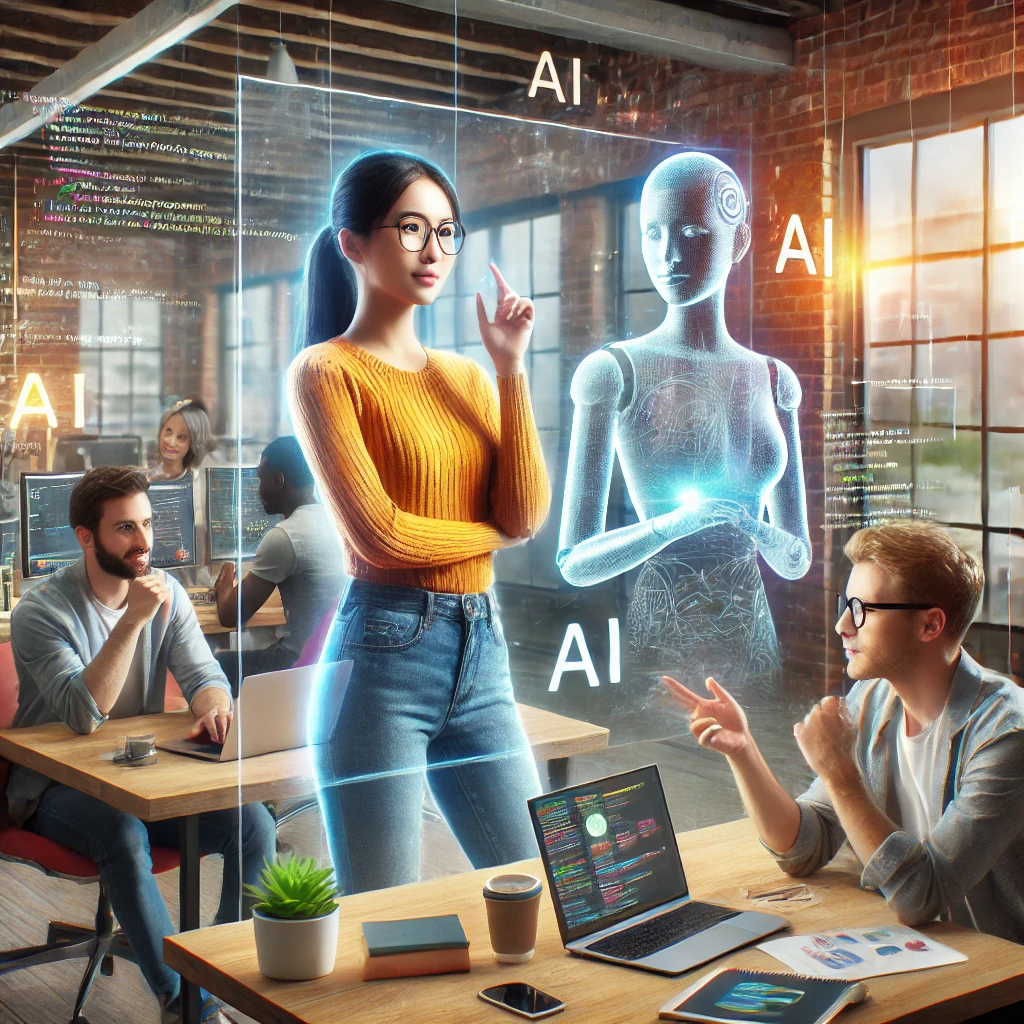
The rapid proliferation of generative AI (GenAI) systems across industries has transformed the technological landscape, but it has also introduced a plethora of safety and security risks. In their latest whitepaper, Lessons from Red Teaming 100 Generative AI Products, the Microsoft AI Red Team provides an in-depth exploration of their efforts to ensure the robustness of GenAI products. This document is not merely a summary of technical findings but a holistic examination of the methodologies, challenges, and learnings derived from red teaming over 100 GenAI systems, with real-world case studies to contextualize their approach.
At its core, red teaming is about understanding the full extent of vulnerabilities within a system, not just at the model level but across its integration with broader applications and workflows. Microsoft’s insights shed light on the criticality of aligning red teaming efforts with realistic risks, demonstrating a shift from traditional safety benchmarking to a more nuanced understanding of AI’s operational contexts.
Central to Microsoft’s methodology is their AI threat model ontology, a structured framework that categorizes vulnerabilities into key components. These include the system itself, the actors (both adversarial and benign), the tactics and techniques employed, and the weaknesses and impacts identified. By focusing on this ontology, Microsoft establishes a comprehensive way to map and analyze risks, moving beyond conventional adversarial scenarios to include unintended failures triggered by benign users.
This framework recognizes that AI systems do not operate in isolation. They exist as part of larger ecosystems, where external applications, data sources, and user interactions introduce new attack vectors. By considering vulnerabilities at both the system and model levels, the ontology reflects a nuanced approach to AI security, ensuring that red teaming captures the complexity of real-world scenarios.
A new paradigm in AI risk assessment
From their extensive experience, Microsoft has distilled eight key lessons, each offering profound insights into the evolving nature of AI red teaming. Unlike traditional safety assessments, red teaming focuses on probing the boundaries of what AI systems can do and identifying risks in their downstream applications. This requires a shift from theoretical models to practical, context-driven evaluations.
For instance, understanding an AI system’s capabilities and constraints forms the foundation of effective risk assessment. Larger models, with their enhanced ability to understand complex instructions, may offer greater utility but are simultaneously more susceptible to exploitation. Similarly, applications that leverage these models such as healthcare tools or financial systems present unique risks depending on their context of use.
One of the most striking realizations is the effectiveness of simple, real-world techniques over computationally intensive gradient-based attacks. While academic research often emphasizes sophisticated methods, adversaries in practice rely on basic strategies such as prompt engineering or exploiting input-output workflows. This insight underscores the importance of adopting a system-level adversarial mindset, which looks beyond individual model vulnerabilities to evaluate the entire AI ecosystem.
Case studies
The whitepaper is rich with illustrative case studies that bring these lessons to life. In one instance, Microsoft red teamers successfully bypassed the safety guardrails of a vision-language model by embedding malicious instructions within images. This highlights the multifaceted nature of vulnerabilities, where text and visual inputs interact in unexpected ways. Similarly, they explored how a large language model (LLM) could be manipulated to create an automated scamming system by integrating text-to-speech and speech-to-text functionalities. These case studies serve as powerful reminders of the creativity and resourcefulness required in identifying and mitigating AI risks.
Another compelling example involves probing a text-to-image generator for bias. By analyzing outputs for scenarios where gender was not specified, the team identified the model’s tendency to reinforce stereotypes, such as depicting secretaries as female and bosses as male. Such findings underline the broader societal implications of generative AI and the need for inclusive safety evaluations.
Role of automation and human expertise
While automation is a valuable tool for scaling red teaming efforts, it cannot replace human judgment and creativity. Microsoft’s development of the PyRIT framework exemplifies how automation can enhance efficiency by generating diverse attack scenarios and analyzing outputs at scale. However, the team emphasizes that tools like PyRIT should augment, not replace, the human element. Subject matter experts (SMEs) are indispensable in evaluating complex or domain-specific risks, such as those involving medical or cultural nuances.
The importance of emotional intelligence is also highlighted, particularly in assessing how AI systems respond to users in distress. Microsoft’s collaboration with psychologists and sociologists to develop guidelines for probing such scenarios reflects their commitment to addressing not just technical vulnerabilities but also psychosocial harms. This human-centric approach ensures that AI systems are evaluated not just for their functionality but for their ethical and emotional impact on users.
Addressing responsible AI harms and security risks
The pervasive nature of responsible AI (RAI) harms such as the generation of biased, harmful, or offensive content poses a unique challenge. Unlike traditional security vulnerabilities, RAI harms are often subjective and context-dependent, requiring tailored approaches for evaluation and mitigation. Microsoft’s distinction between adversarial and benign user scenarios is particularly insightful, as it underscores the importance of designing systems that are resilient to unintentional failures.
In addition to addressing model-specific risks, the integration of generative AI into larger applications has introduced new attack vectors. For example, server-side request forgery (SSRF) vulnerabilities in a video-processing system underscore the importance of securing not just AI models but also their surrounding infrastructure.
Continuous improvement and collaboration
Microsoft’s whitepaper concludes with a call to action for the AI community. They emphasize that the work of securing AI systems will never be complete, as new capabilities and risks continue to emerge. This requires a commitment to iterative “break-fix” cycles, where vulnerabilities are continuously identified and addressed, and to fostering collaboration across organizations and disciplines.
Furthermore, the need for regulatory frameworks that balance innovation with accountability is clear. By aligning technical advancements with policy and economic incentives, the industry can create a more secure foundation for AI development.
- FIRST PUBLISHED IN:
- Devdiscourse