Revolutionizing Poverty Prediction in the Philippines: CatBoost Leads with 90% Accuracy
A study by Erika Lynet V. Salvador from Amherst College and De La Salle University shows that CatBoost, a machine learning model, excels in predicting poverty levels in the Philippines with over 90% accuracy. This research highlights the importance of advanced algorithms for effective poverty alleviation strategies.
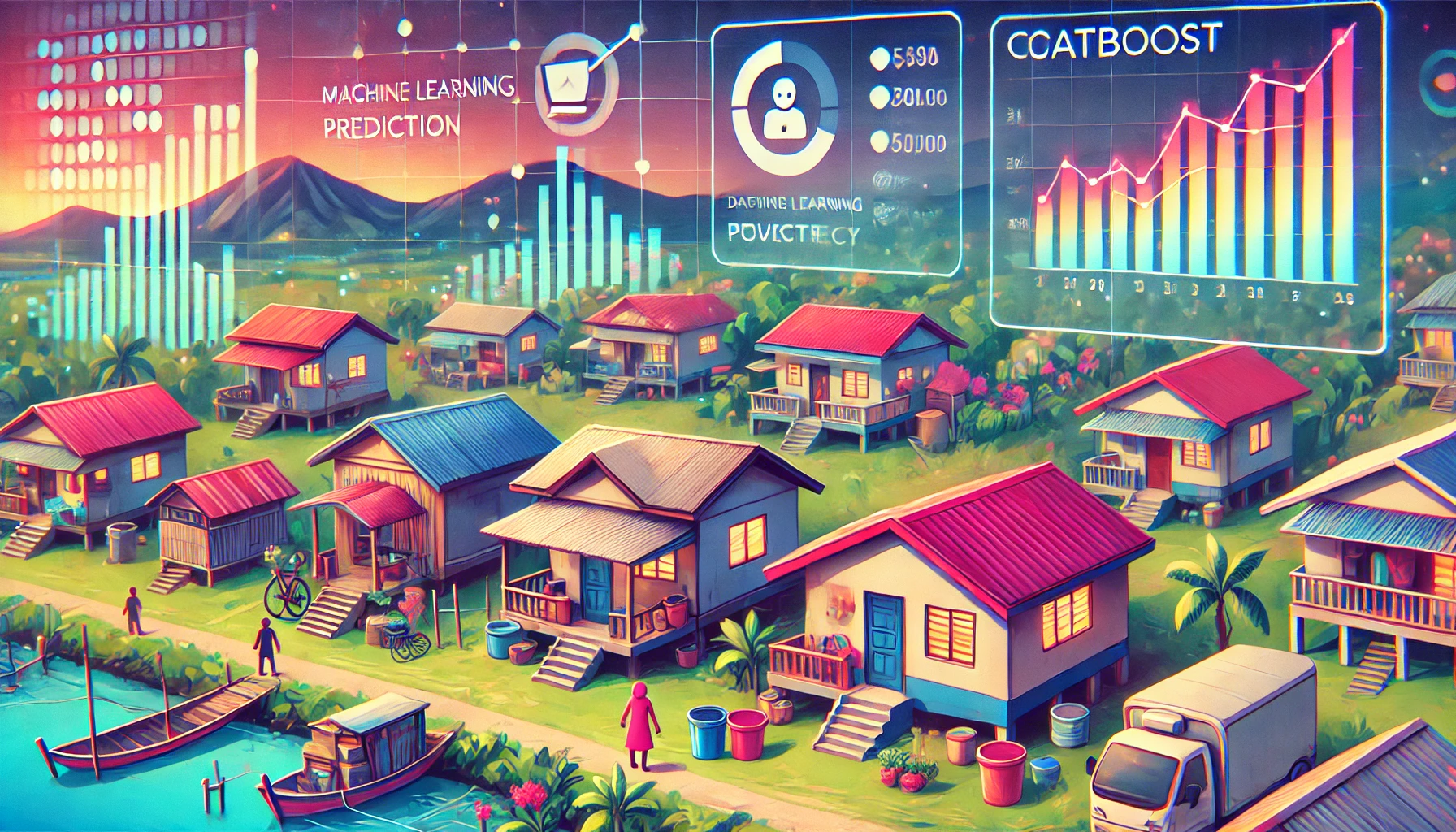
In the Philippines, a novel study by Erika Lynet V. Salvador from the Department of Mathematics and Statistics at Amherst College and the Senior High School Department at De La Salle University Integrated School in Manila delves into the effectiveness of machine learning models in predicting poverty levels. The study compares five boosting algorithms: Adaptive Boosting (AdaBoost), Cat Boosting (CatBoost), Gradient Boosting Machine (GBM), Light Gradient Boosting Machine (LightGBM), and Extreme Gradient Boosting (XGBoost). Among these, CatBoost emerged as the frontrunner, achieving the highest accuracy at 90.93%, followed closely by XGBoost, GBM, and LightGBM, with AdaBoost lagging behind. CatBoost also led in precision, recall, and F1-score, making it the most reliable model for poverty prediction.
Machine Learning Models Outperform Traditional Methods
The research highlights the importance of accurate data in formulating effective poverty alleviation policies. Traditional econometric models often fail to capture the multidimensional nature of poverty, which includes aspects beyond income, such as education and healthcare deficits. Machine learning models, however, can handle high-dimensional data and uncover hidden patterns, offering a more nuanced understanding of poverty. This capability is crucial for developing targeted interventions that can genuinely uplift impoverished communities. The dataset for this study was sourced from the 2022 Demographic and Health Survey (DHS) in the Philippines, initially comprising 2,099 features from 30,372 households. After rigorous data cleaning and feature selection processes, the dataset was refined to 396 features from 20,679 households. The study employed various performance metrics to evaluate the models, including accuracy, precision, recall, F1-score, and AUC-ROC scores. CatBoost achieved the highest performance across all these metrics, followed by XGBoost, GBM, and LightGBM, with AdaBoost showing significantly lower scores.
Efficiency Meets Accuracy: CatBoost’s Superior Performance
Additionally, the study examined the computational efficiency of the models. AdaBoost had the shortest training time but the longest testing time, while CatBoost, despite its longer training duration, demonstrated exceptional efficiency during testing. GBM, LightGBM, and XGBoost balanced well between training and testing times, with LightGBM and XGBoost also showing smaller model sizes. These findings indicate that CatBoost is not only the most accurate but also efficient in practical applications. The study also underscored the most impactful features in predicting poverty, such as the source of drinking water, type of toilet facility, and ownership of various household items. These insights can guide policymakers in identifying key areas for intervention. Future research could explore how changes in these features affect poverty predictions, incorporating more complex data types like GPS and night light data to enhance predictive accuracy.
Unveiling the Multifaceted Nature of Poverty
One of the key takeaways from this study is the ability of machine learning models to offer a more detailed and accurate representation of poverty compared to traditional methods. By including a wide range of household characteristics and employing sophisticated algorithms, the study was able to capture the multifaceted nature of poverty. This is particularly important for developing countries like the Philippines, where poverty is not solely defined by income but also by access to basic needs and services. The findings from this study can be used to inform policy decisions and target interventions more effectively, ensuring that resources are directed towards the households that need them the most.
Harnessing Advanced Techniques for Social Good
Moreover, the study’s use of boosting algorithms highlights the potential of advanced machine learning techniques in social science research. Boosting algorithms are particularly well-suited for this type of analysis because they can handle large datasets with many features, automatically identifying the most relevant variables and capturing complex interactions. The success of CatBoost in this study underscores its robustness and efficiency, making it a valuable tool for researchers and policymakers alike. However, it is also important to recognize the limitations of this study. While the DHS data provides a comprehensive overview of household characteristics, it may not capture all aspects of poverty, particularly those related to social and cultural factors. Furthermore, the study’s reliance on a single dataset means that the findings may not be generalizable to other contexts. Future research should consider incorporating additional data sources and exploring other machine learning techniques to build on these findings.
Global Implications for Poverty Alleviation
The implications of this study extend beyond the Philippines. As governments worldwide strive to achieve the Sustainable Development Goal of eradicating poverty by 2030, accurate and reliable data is more important than ever. Machine learning models like those used in this study offer a powerful tool for understanding and addressing poverty, enabling more targeted and effective interventions. By leveraging the strengths of machine learning, policymakers can gain deeper insights into the dynamics of poverty and develop strategies that are tailored to the specific needs of their populations. In conclusion, this study represents a significant step forward in the use of machine learning for poverty prediction. By demonstrating the effectiveness of boosting algorithms, particularly CatBoost, in accurately predicting household poverty levels, it provides a valuable contribution to the field of social science research. The insights gained from this study can inform policy decisions and help to create a more targeted and effective approach to poverty alleviation, ultimately improving the lives of those most in need.
- FIRST PUBLISHED IN:
- Devdiscourse