Real-Time Economic Predictions: Leveraging Regime-Switching Models for Accurate Nowcasting
The paper explores the use of regime-switching dynamic factor models (DFM) and the Expectation-Maximization (EM) algorithm for more accurate and efficient nowcasting of economic activity, particularly U.S. GDP. The model improves forecasting by adapting to shifts in economic conditions, offering timely insights for policymakers.
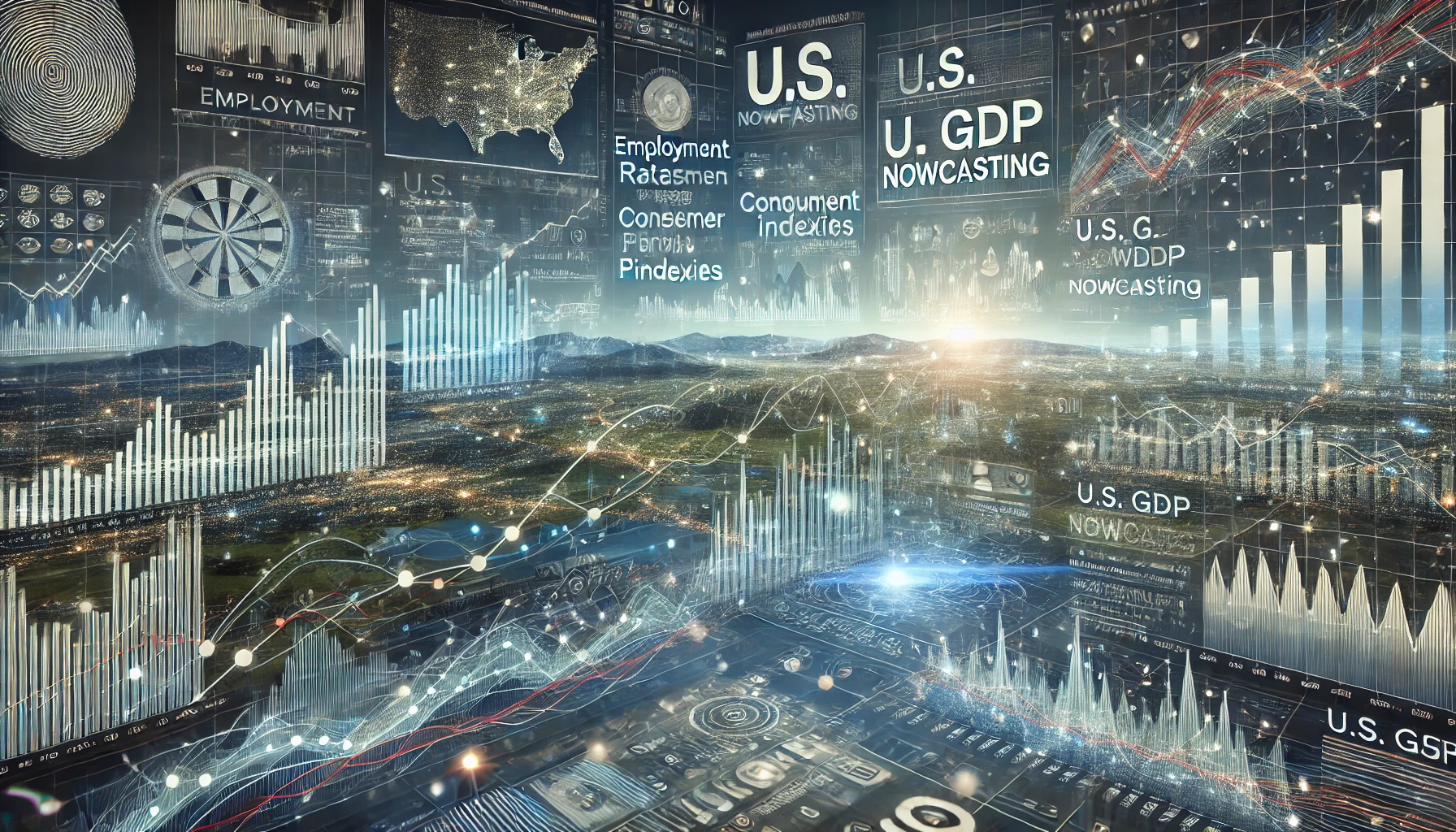
A comprehensive study by Omer Faruk Akbal, published by the International Monetary Fund in 2024, focuses on improving the accuracy and speed of nowcasting models through the application of a regime-switching dynamic factor model (DFM) and the Expectation-Maximization (EM) algorithm. Nowcasting is the practice of predicting current economic conditions, like GDP, using high-frequency data before official statistics are available. Traditional models often fail to capture the turning points in business cycles, such as recessions and expansions, which limits their effectiveness in real-time economic forecasting. Akbal's work addresses this shortcoming by proposing a regime-switching approach that allows the model to adapt to different economic states, providing more accurate predictions of economic activity.
The Efficiency of the Expectation-Maximization Algorithm
The central contribution of the paper is the development of an efficient estimation method using the EM algorithm. Unlike traditional approaches that rely on complex numerical maximization techniques, the EM algorithm offers closed-form solutions for parameter estimation, which significantly reduces the computational burden. This makes it especially suitable for large-dimensional datasets that are common in real-time economic forecasting. The regime-switching DFM is particularly effective because it adjusts to changes in the underlying economic conditions, making it possible to capture shifts between different regimes, such as periods of recession or expansion, that would otherwise be missed by static models.
Application to U.S. GDP Data and Key Findings
The regime-switching model introduced in this paper is applied to U.S. GDP data over a 15-year period, using vintage data that reflects the information available at the time each forecast was made. Akbal demonstrates that the regime-switching model outperforms traditional one-regime models, especially when key economic indicators are released. The paper highlights that these indicators, such as employment figures or consumer price indices, often signal significant shifts in economic conditions. By incorporating these signals into the nowcasting process, the regime-switching model can adjust more effectively to new information, resulting in more accurate and timely forecasts. One of the most compelling findings in the paper is the model's ability to closely match the recession dates identified by the National Bureau of Economic Research (NBER), despite having less real-time data than the NBER committees.
Backcasting Recessions and Improved Precision
In addition to nowcasting, the paper explores the potential of regime-switching DFMs for backcasting estimating past economic conditions using current data. Akbal shows that as more data becomes available, the model's estimates of when recessions end become increasingly accurate, typically within a lag of three to six months. This delay aligns with the time it takes for official statistics, such as GDP figures, to be revised and finalized. The ability to predict both the onset and end of recessions with such precision is a key benefit of the regime-switching model, particularly for policymakers who need to make timely decisions in response to economic downturns. The flexibility of the model is another notable feature, allowing it to be applied to various configurations of regime-switching.
Comparing Traditional and Regime-Switching Models
The paper also provides a detailed comparison between the regime-switching model and more traditional approaches. In a replication exercise, Akbal compares the EM algorithm with both a one-step estimation approach, which combines the Kalman and Hamiltonian filters, and a two-step approach, which separates the extraction of factors from the estimation of the model. The results show that while all methods produce similar estimates of recession probabilities, the EM algorithm is significantly faster. This speed advantage is particularly valuable in real-time forecasting, where rapid updates are critical for accurate nowcasting.
Implications for Policymaking and Future Research
The model’s performance was tested using a real-time nowcasting application for U.S. GDP, extending the New York Federal Reserve’s well-known nowcasting model, which was suspended during the COVID-19 pandemic. Akbal's regime-switching modification to this model allowed it to handle the large nonlinearities introduced by the pandemic, demonstrating superior forecasting performance, particularly after 2013. During the COVID-19 pandemic, the regime-switching model's forecasting accuracy improved notably, highlighting its robustness in times of economic crisis. The ability of the regime-switching model to closely track NBER recession dates in real-time, despite having less information than the NBER committees, underscores its practical utility. Akbal's work shows that the model not only excels in forecasting but also in recession dating, providing policymakers with a valuable tool for identifying economic turning points. This can be particularly useful for central banks and economic planners who need to act preemptively in response to economic shifts. The paper concludes that the regime-switching DFM, with its flexible structure and efficient estimation via the EM algorithm, offers a significant advancement in nowcasting methodologies. Its ability to handle large datasets, adapt to changing economic conditions, and provide timely forecasts makes it a powerful tool for both economic researchers and policymakers.
- FIRST PUBLISHED IN:
- Devdiscourse