Enhancing Poverty Estimates: The Role of Utility and Food Expenditures in Data Imputation
The World Bank's study explores using survey-to-survey imputation to estimate poverty indicators in countries with inadequate data, showing that adding utility and food expenditures significantly improves accuracy. This approach offers a cost-effective alternative to traditional surveys, particularly in regions with limited statistical capacity.
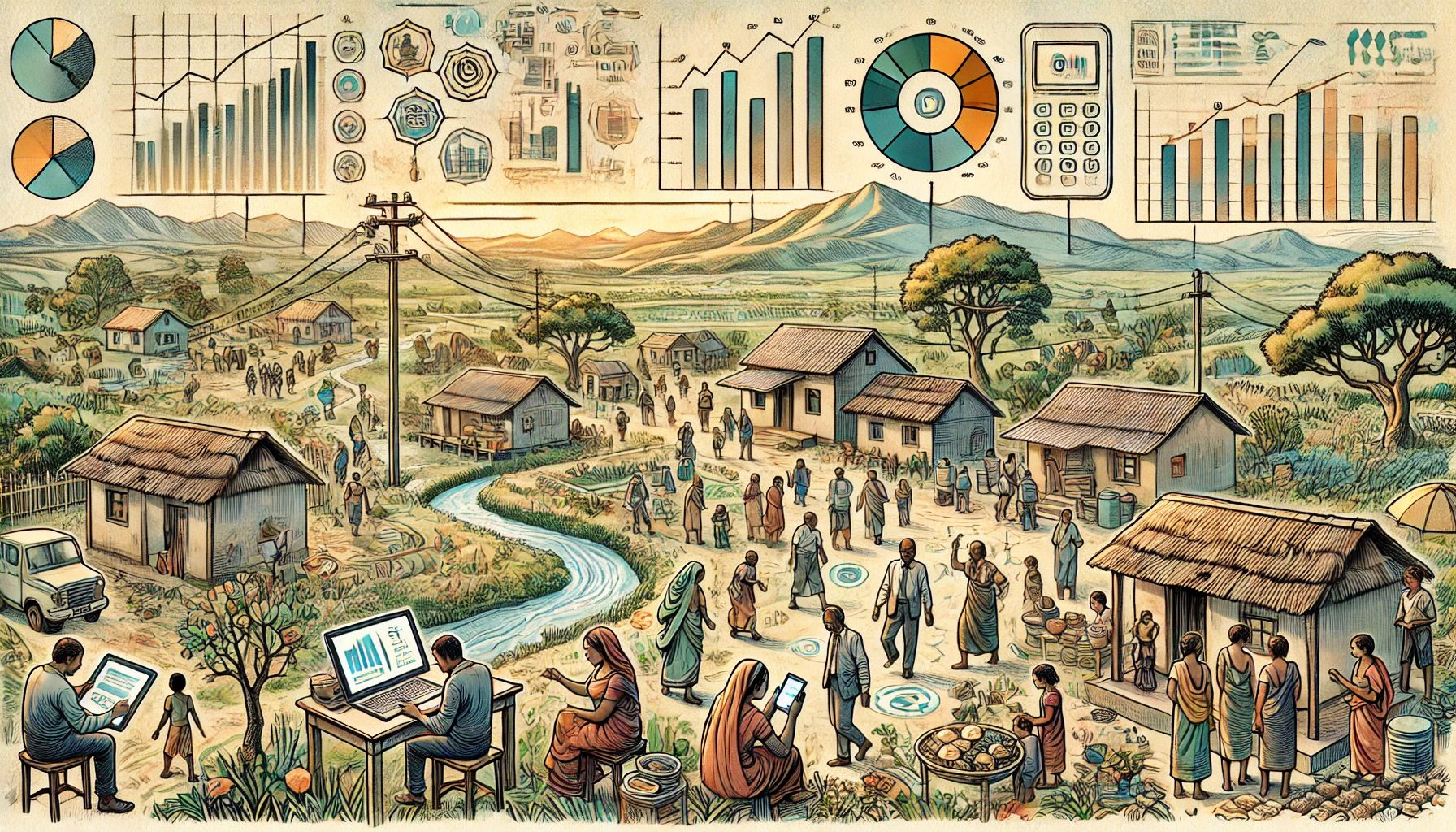
A recent study conducted by researchers from the World Bank's Development Data Group sheds light on significant challenges in accurately measuring poverty, especially in poorer countries where household consumption data is often inadequate, outdated, or inconsistent. The study, led by Hai-Anh H. Dang, Talip Kilic, Kseniya Abanokova, and Calogero Carletto, proposes an alternative approach known as survey-to-survey imputation to address these issues. This method allows for the estimation of various poverty indicators, such as headcount poverty, extreme poverty, the poverty gap, and near-poverty rates, without the need for direct consumption data, which is often difficult to obtain in many developing regions.
A Comprehensive Analysis Across Diverse Regions
The researchers conducted a comprehensive analysis using data from 22 multi-topic household surveys conducted over the past decade in six countries: Bangladesh, Ethiopia, Malawi, Nigeria, Tanzania, and Viet Nam. These countries were chosen because they represent a diverse range of regions, including Sub-Saharan Africa, South Asia, and Southeast Asia, and vary in income levels, from low-income to lower-middle-income countries. This diversity provided a broad testing ground for the imputation models, allowing the researchers to assess the effectiveness of these models across different economic contexts and geographical locations.
Improving Accuracy with Utility and Food Expenditures
One of the key findings of the study is that adding household utility expenditures, such as those for electricity, water, and garbage, or food expenditures to basic imputation models significantly improves the accuracy of poverty estimates. The study shows that these additional variables can increase the probability of accurate imputation by 0.1 to 0.4, depending on the specific poverty indicator being estimated. Moreover, the inclusion of predictors from geospatial data, such as agricultural soil quality or the distance to essential facilities, can further enhance the accuracy of these imputations. However, the researchers also found that the time interval between the base survey (the older survey with complete data) and the target survey (the more recent survey with missing consumption data) is a critical factor in determining the reliability of the imputation. A longer time gap between surveys generally leads to lower accuracy in predicting poverty indicators, highlighting the importance of conducting surveys at regular intervals to maintain the reliability of poverty estimates.
Challenges in Model Accuracy and Fit
The analysis also revealed that a better model goodness-of-fit, often measured by R-squared (R2), does not necessarily lead to more accurate poverty estimates. This finding suggests that while statistical models may fit the data well, they might not always be the best tools for predicting poverty outcomes, particularly in the context of imputation. Instead, the study emphasizes the importance of choosing the right variables for the imputation model, rather than relying solely on traditional measures of model fit. The researchers tested several imputation models, each adding different types of expenditures to a basic set of household demographic and employment variables. Two models stood out in particular: one that added food expenditures and another that added utility expenditures. These models consistently performed better than others, particularly in predicting headcount poverty, extreme poverty, the poverty gap, and mean consumption levels.
Machine Learning: A Promising but Inconsistent Tool
Furthermore, the study explored the potential of machine learning (ML) techniques as an alternative to traditional imputation methods. While ML techniques like LASSO, Elastic Net, and Random Forest are popular in many fields for their predictive power, the researchers found that these methods did not consistently outperform traditional imputation models in this context. The ML techniques were particularly inconsistent in estimating poverty indicators, though they showed some promise in predicting mean consumption levels. This suggests that while ML holds potential, it may not yet be the best approach for poverty imputation in the absence of consumption data, particularly when compared to more straightforward statistical models that have been specifically tailored to the context of poverty measurement.
The study by the World Bank researchers provides valuable insights into the challenges and opportunities in poverty measurement, particularly in settings where data collection is difficult or costly. The findings suggest that survey-to-survey imputation, particularly when combined with additional variables like utility and food expenditures, offers a viable and cost-effective alternative to traditional survey methods. This approach could be especially useful in countries with limited statistical capacity, where the regular collection of detailed consumption data is not feasible. As digitalization continues to advance, particularly in payment transactions, the integration of such data into imputation models may further enhance the accuracy and timeliness of poverty estimates, ultimately supporting more effective poverty reduction policies.
- FIRST PUBLISHED IN:
- Devdiscourse