Harnessing AI to Identify Quit-Vaping Intentions: A Collaborative Study on GPT-4’s Potential
Researchers from leading universities collaborated to explore GPT-4's ability to detect quit-vaping intentions from Reddit posts, finding it promising but not yet matching human annotators. Future work will focus on enhancing the model and expanding datasets for improved public health research.
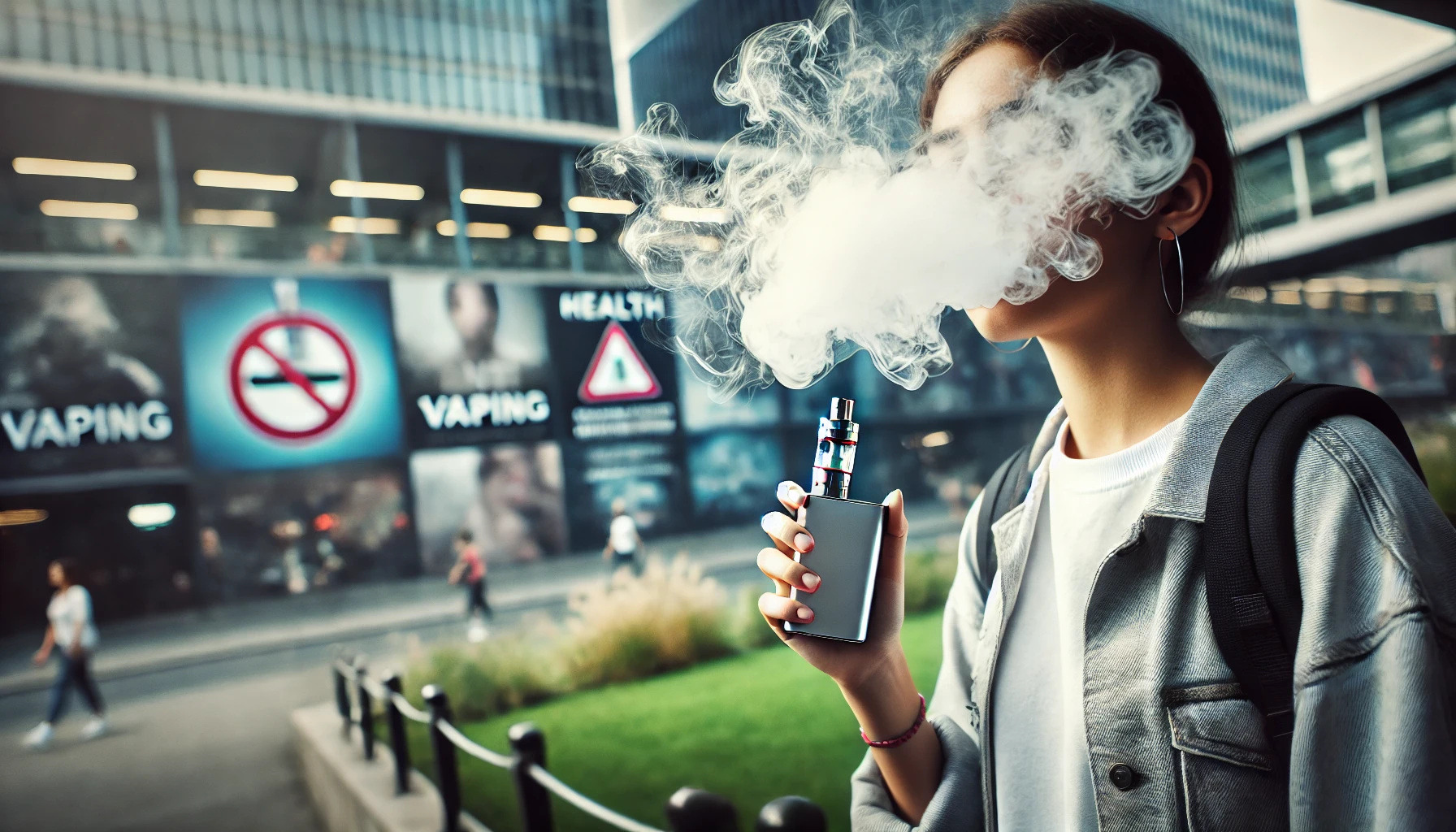
In recent years, the United States has witnessed a significant rise in vaping and e-cigarette use, particularly among adolescents and young adults. This increase has led to a surge in cases of e-cigarette and vaping-associated lung injury (EVALI), highlighting the urgent need to understand vaping behaviors and develop effective cessation strategies. Researchers from the University of South Carolina, Washington University in St. Louis, and the University of Texas Health Science Center have collaborated on a study to explore the potential of OpenAI’s GPT-4 model in detecting quit-vaping intentions by analyzing posts from a vaping sub-community on Reddit. The EVALI outbreak in 2019, which resulted in numerous hospitalizations and fatalities, emphasized the dangers of vaping and the necessity for targeted public health interventions. Social media platforms, which have become integral to communication, connectivity, and information dissemination, offer a valuable resource for public health research. With over 4.7 billion users worldwide, social media platforms like Reddit provide a wealth of organic data that can be leveraged to gain insights into public health trends, including vaping behaviors and cessation intentions.
A Collaborative Effort to Combat Vaping
The study aimed to evaluate the performance of GPT-4 against layman and clinical expert annotations in detecting quit-vaping intentions. Various prompting strategies, including zero-shot, one-shot, few-shot, and chain-of-thought prompting, were employed to instruct GPT-4 on the annotation task. These strategies were designed to assess how well GPT-4 could identify users' intentions to quit vaping based on the content of their posts.
The research team extracted 1,000 posts from the r/QuitVaping subreddit, which is dedicated to helping users quit vaping and other tobacco products. From this initial dataset, approximately 120 posts were randomly selected to form a sample dataset. Each post was broken down into sentences using a sentence tokenizer, resulting in 1,059 sentences for annotation. Layman annotators and clinical experts were tasked with labeling these sentences as 'YES' if the speaker explicitly mentioned their intention to quit vaping, and 'NO' otherwise. Discrepancies in the annotations were resolved internally, ensuring a high level of inter-coder reliability.
Insights from Preliminary Findings
The preliminary findings revealed that GPT-4 has significant potential in social media data analysis, particularly in identifying subtle quit-vaping intentions that might be missed by human annotators. However, the model's performance varied depending on the prompting strategy used. The study found that prompts with more detailed instructions generally led to better performance, although too much detail could also be detrimental. High-detail prompts (P5-P8) outperformed low-detail prompts (P1-P4) in terms of accuracy and F1 score, but they also resulted in lower recall values for positive cases.
Qualitative evaluation using Cohen’s Kappa and Jaccard’s similarity scores indicated that layman annotations were closer to the expert annotations compared to GPT-4. Quantitative evaluation showed that while GPT-4 had a lower precision due to a higher number of false positives, its overall performance was promising. The model’s sensitivity decreased with increased detail in the prompts, highlighting the importance of careful prompt construction. Despite these limitations, GPT-4 demonstrated an impressive ability to handle the annotation task, suggesting that it could serve as a valuable tool in social media analytics for public health research.
Strategies for Optimizing GPT-4 Performance
To further improve the performance of GPT-4, the study suggests optimizing the prompts and providing more context to the model. Observing the annotated dataset in the context of the model’s confidence scores and reasoning provided valuable insights that could be used to enhance its accuracy. For example, the model often assigned low confidence scores when making assumptions about the context of a sentence, indicating areas where additional context or clarification could improve its predictions.
The Potential of GPT-4 in Public Health
While GPT-4 cannot yet replace human annotators, it has demonstrated significant potential in the field of social media data analysis for public health research. By continuing to refine the model and expand the dataset, researchers can enhance its ability to detect quit-vaping intentions and provide valuable support for public health initiatives aimed at reducing vaping among adolescents and young adults. This research was generously funded by a NIH R34 grant and a research grant from the University of South Carolina. The authors have no conflicts of interest to declare.
Future Directions in Vaping Research
Future research will focus on expanding the dataset to include a larger and more diverse sample of posts and comments from popular vaping subreddits. This will help to make the model more robust and capable of handling a wider range of vaping behaviors and quitting intentions. Additionally, the study plans to explore multi-label classification to provide more granular insights into users' quitting journeys. By identifying users at different stages of their quitting process, researchers can develop more targeted intervention programs that address the specific needs of each group.
- FIRST PUBLISHED IN:
- Devdiscourse