AI-Powered Mask Detection: Innovative Solutions for Enforcing Mask Mandates in Public Spaces
Researchers from several global institutions have developed a deep learning-based system using Convolutional Neural Networks (CNNs) to detect face masks in real-time, achieving up to 99% accuracy in daylight and addressing enforcement challenges in crowded public spaces during the COVID-19 pandemic. This AI-driven solution can function under various lighting conditions and aims to enhance public health safety by integrating with surveillance technologies.
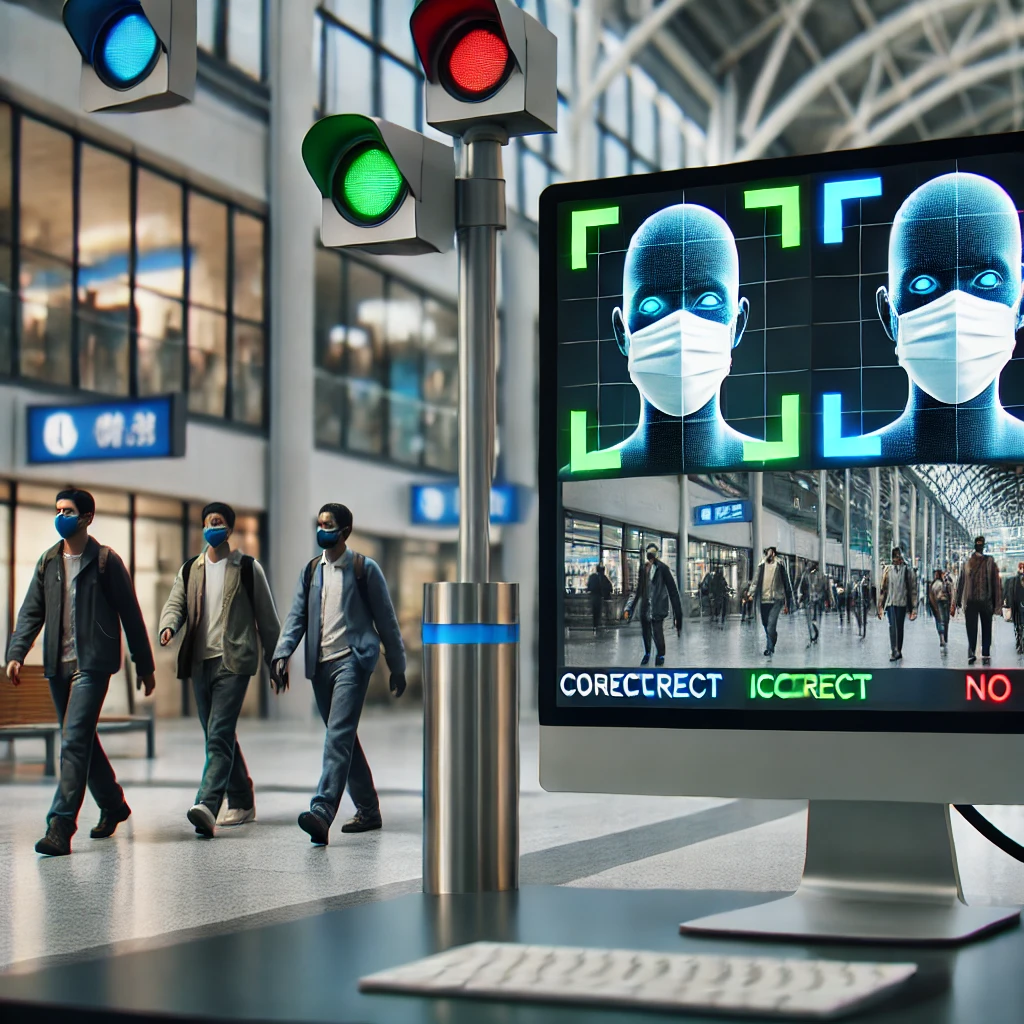
The global COVID-19 pandemic has dramatically changed our lives, with one of the most significant measures to curb its spread being the use of face masks. The World Health Organization (WHO) has recommended mask-wearing in public, especially in crowded places, to reduce transmission. Despite mandates in many countries, enforcing mask compliance in large crowds remains a formidable challenge. Researchers have turned to artificial intelligence (AI) to address this issue.
Cutting-Edge AI Solution for Mask Detection
A new study by researchers at Jamia Hamdard in New Delhi, VIT University in Vellore, Friedrich-Alexander-Universitat Erlangen-Nurnberg in Germany, Arizona State University, and the University of Stirling in the UK proposes a deep learning technique to detect face masks in real-time, using Convolutional Neural Networks (CNNs) that can identify whether a person is wearing a mask correctly, incorrectly, or not at all. This system works with webcam images and videos, both in still and motion and can function under various lighting conditions, including nighttime. The proposed method boasts an impressive accuracy, ranging from 74 percent for multiple people in low light to 99% for a single person in daylight.
Pandemic-Driven Innovation
The initial phase of the COVID-19 pandemic saw WHO advising mask use in public due to high transmission rates in crowded places. The pandemic has driven innovations in AI to monitor mask compliance. For instance, in France, AI software in surveillance cameras is used to ensure mask-wearing in the Paris metro. This software, developed by Dataka Lab, gathers statistical data on mask usage to help predict potential COVID-19 outbreaks. The task of face mask detection involves two main challenges: detecting faces in digital images and classifying whether those faces are wearing masks. While face detection has been widely studied in computer vision, mask classification gained prominence with the pandemic. Numerous studies have been published on this topic, but many do not address real-time detection in varied conditions like low light or crowded scenes.
Building a Comprehensive Dataset
The study's dataset for training the CNN combines two existing datasets: the Masked FaceNet and the Face Mask Dataset. The new combined dataset, called Masked Face Detection trio (MFD_trio), categorizes images into correct mask, incorrect mask, and without mask. The dataset includes 2534 images for training and 293 images for testing, covering various scenarios for both daylight and nightlight. The CNN model processes images with 150x150 pixels and uses multiple layers of convolution and pooling, followed by dense layers for classification. The model achieved a training accuracy of 98.9 percent and a testing accuracy of 98.74 percent. For real-time implementation, the system uses OpenCV to capture video streams and detect faces using a pre-trained Haar Cascade model. The detected faces are then classified by the CNN into one of the three categories. The system draws colored rectangles around faces to indicate mask status: green for correct, blue for incorrect, and red for no mask.
Real-Time Testing and Results
Testing the model in real-time scenarios showed strong performance. In daylight, the system achieved 99 percent accuracy for single-person frames and 98 percent for multiple-person frames. At night, the accuracy was 88 percent for single-person frames and 74 percent for multiple-person frames. The model's quick processing time ensures no lag between the video stream and the detection results. In conclusion, the proposed deep learning-based face mask detection system offers a robust solution for monitoring mask compliance in real time. Its high accuracy and adaptability to different conditions make it a valuable tool in the ongoing fight against COVID-19. Future improvements could include enhancing night-time accuracy with better cameras and identifying different mask types and colors. This technology could be integrated with CCTV systems or drones to monitor large gatherings and help prevent the spread of airborne diseases.
- FIRST PUBLISHED IN:
- Devdiscourse