Harnessing prompt engineering for deeper student engagement and learning
The study offers a compelling vision for the future of education, where AI serves as a co-learner and collaborator in the classroom. By leveraging the Chain-of-Thought approach, LLMs can help students engage more deeply with challenging problems, refine their ideas, and contribute meaningfully to collective knowledge creation.
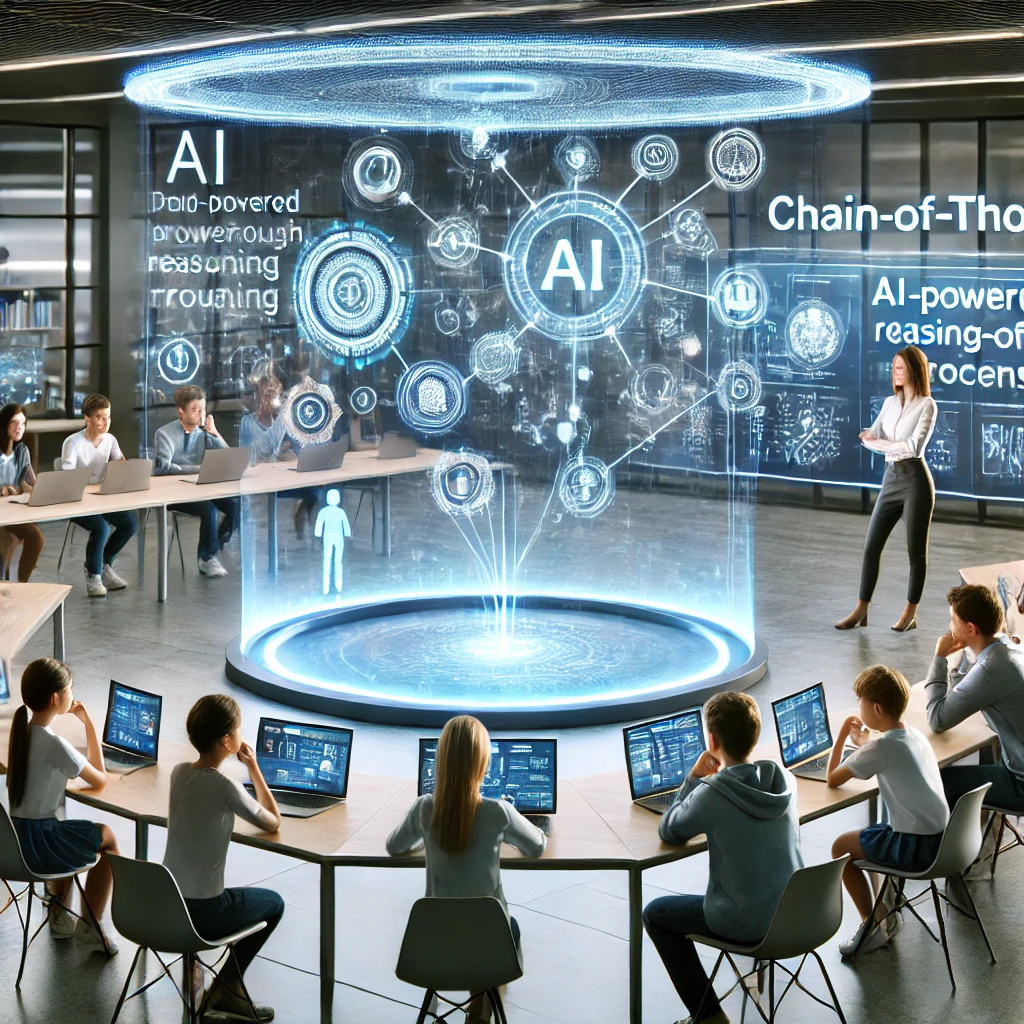
The integration of artificial intelligence (AI) in education is paving the way for transformative learning experiences, enabling students to engage in knowledge creation like never before. The study, "Prompt Engineering for Knowledge Creation: Using Chain-of-Thought to Support Students' Improvable Ideas," authored by Alwyn Vwen Yen Lee, Chew Lee Teo, and Seng Chee Tan, and published in AI (2024), provides an in-depth exploration of how prompt engineering, particularly the Chain-of-Thought (CoT) approach, can elevate learning. By leveraging large language models (LLMs) equipped with CoT capabilities, this research highlights a powerful method to support students in refining their ideas, enhancing critical thinking, and fostering deeper engagement with complex problems.
Challenge of knowledge creation in education
Knowledge creation is a cornerstone of modern education, encompassing the ability to generate, critique, and refine ideas collaboratively. However, traditional learning environments often fail to equip students with the necessary tools to explore knowledge gaps, challenge assumptions, and improve their contributions effectively. Limited access to diverse perspectives, inadequate resources, and time constraints further exacerbate these challenges, leaving students struggling to engage in meaningful discourse.
Generative AI, particularly LLMs, is changing this narrative. These tools, designed to generate human-like text, are proving invaluable in enhancing student learning by offering immediate, diverse, and insightful responses to queries. The Chain-of-Thought (CoT) prompting technique amplifies this capability, enabling LLMs to engage in intermediate reasoning steps, thereby producing outputs that mirror human cognitive processes. This approach not only supports students in refining their ideas but also helps them develop the metacognitive skills necessary for lifelong learning.
How chain-of-thought transforms learning
The Chain-of-Thought prompting technique represents a paradigm shift in how AI interacts with human learners. Unlike standard prompts that elicit direct and often surface-level answers, CoT prompts encourage the AI to reason step-by-step through complex problems. This structured reasoning process leads to more comprehensive, accurate, and actionable outputs, making it an ideal tool for supporting educational activities.
For example, when students posed questions about sustainability during the study, CoT-enabled LLMs provided responses that went beyond basic answers. They included detailed breakdowns of the problem, explored interconnected factors, and suggested practical, innovative solutions. This method aligns with how human cognition operates, encouraging iterative thinking and the incremental improvement of ideas.
Key findings from real-world applications
The study analyzed data from a two-day Knowledge Building Design Studio focused on sustainability, collecting 721 discourse turns, which included 272 question-answer pairs. The responses generated by CoT-augmented LLMs consistently outperformed those from human participants in terms of depth, coherence, and utility.
In one example, students asked about the environmental benefits of plant-based diets. The CoT-enabled AI provided a nuanced response that covered reduced greenhouse gas emissions, conservation of water resources, and mitigation of deforestation. The answer not only addressed the question but also offered students additional pathways for exploration, such as economic and social implications.
Another instance involved exploring harmonious coexistence between humans and wildlife. The AI, using CoT, proposed solutions like urban planning techniques to minimize habitat disruption, community-led conservation initiatives, and educational programs to foster coexistence. These responses demonstrated the AI's ability to synthesize diverse perspectives and present actionable recommendations, making it an invaluable partner in the learning process.
Implications for educators and students
The integration of CoT into educational tools has far-reaching implications for educators and students. For educators, CoT-enabled LLMs can serve as a collaborative partner, providing tailored support for lesson planning, resource generation, and addressing students' questions. Teachers can use AI to encourage deeper inquiry among students, guiding them toward more sophisticated understandings of complex topics.
For students, interacting with CoT-augmented AI offers an opportunity to develop critical thinking and problem-solving skills. By engaging with detailed, step-by-step responses, students learn to analyze problems holistically, consider alternative perspectives, and iteratively improve their ideas. This approach not only enhances individual learning outcomes but also fosters collaborative knowledge-building within groups.
Ethical and practical considerations
As with any technological innovation, the use of CoT in education comes with ethical and practical considerations. Ensuring equitable access to AI tools is paramount to prevent widening educational disparities. Policymakers must work to provide affordable, scalable solutions that benefit all learners, regardless of socioeconomic background.
Additionally, training students in effective prompt engineering is crucial for maximizing the utility of CoT-enabled LLMs. Understanding how to craft effective prompts allows students to extract meaningful insights from AI systems and ensures that the interaction is constructive and relevant to their learning objectives.
Transparency in AI operations is another critical factor. Students and educators must understand how AI generates responses to build trust and use these tools responsibly. Ensuring that AI-generated content is accurate, unbiased, and aligned with educational goals is an ongoing challenge that requires collaboration between developers, educators, and policymakers.
The road ahead
The study offers a compelling vision for the future of education, where AI serves as a co-learner and collaborator in the classroom. By leveraging the Chain-of-Thought approach, LLMs can help students engage more deeply with challenging problems, refine their ideas, and contribute meaningfully to collective knowledge creation.
As educational institutions and policymakers embrace AI-driven solutions, the findings of this research emphasize the importance of aligning technology with pedagogical goals. CoT-augmented LLMs not only enhance individual learning experiences but also promote a culture of collaborative inquiry and innovation. This integration has the potential to democratize education, making high-quality learning resources accessible to students worldwide.
The journey toward AI-enhanced education is just beginning, but studies like this provide a roadmap for harnessing the power of technology to empower learners, support educators, and build a more equitable and innovative future for education.
- READ MORE ON:
- Prompt engineering in education
- Chain-of-Thought AI learning
- AI-driven knowledge creation
- Collaborative learning with AI
- AI tools for students
- Educational prompt engineering techniques
- AI-enhanced classrooms
- Generative AI for knowledge building
- AI prompt engineering for critical thinking
- FIRST PUBLISHED IN:
- Devdiscourse