Strengthening online payments with GANs: A shield against AI-driven fraud
Generative Adversarial Networks (GANs), a cornerstone of modern AI, have gained recognition for their ability to generate highly realistic synthetic data. While this capability is often exploited for malicious purposes, the research leverages GANs to counteract deepfake fraud. The proposed model employs a generator to create synthetic fraudulent images and a discriminator to distinguish these images from genuine payment data. This adversarial training enhances the model’s ability to identify anomalies in real-world scenarios.
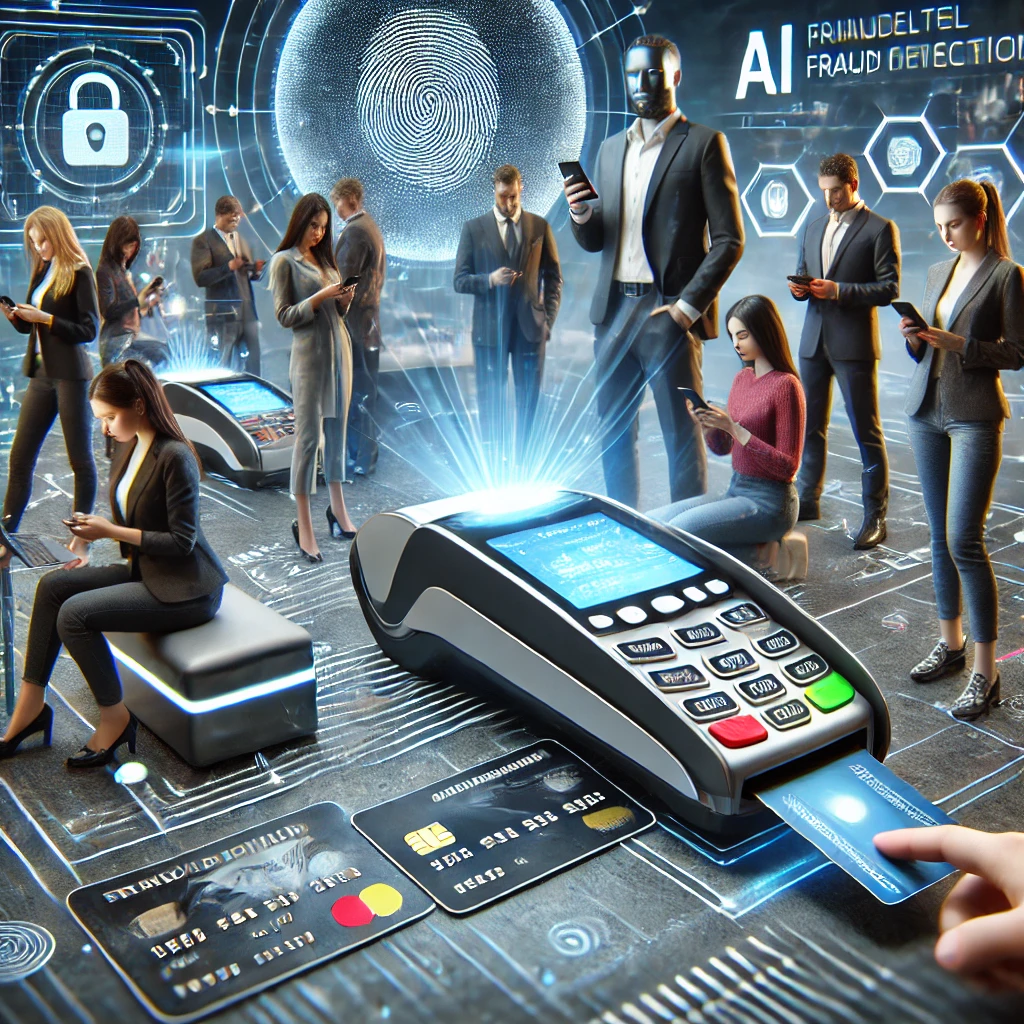
As digital payments become the norm in a rapidly digitizing world, the rise of AI-driven deepfake technologies poses a serious threat to payment security. Deepfakes, capable of manipulating facial features in images and videos, have transitioned from entertainment to malicious domains such as identity theft and fraud in online transactions. The paper "Detection of AI Deepfake and Fraud in Online Payments Using GAN-Based Models" by Zong Ke, Shicheng Zhou, Yining Zhou, Chia Hong Chang, and Rong Zhang, to be published at the 8th International Conference on Advanced Algorithms and Control Engineering (ICAACE 2025) by IEEE, presents a novel solution to this emerging problem.
This research introduces a GAN-based framework that detects deepfake-driven fraud in online payments with unprecedented accuracy. By leveraging advanced generative adversarial networks (GANs), the authors propose a system capable of identifying subtle manipulations in payment images, thereby reinforcing the robustness of financial security frameworks.
Role of GANs in fraud detection
Generative Adversarial Networks (GANs), a cornerstone of modern AI, have gained recognition for their ability to generate highly realistic synthetic data. While this capability is often exploited for malicious purposes, the research leverages GANs to counteract deepfake fraud. The proposed model employs a generator to create synthetic fraudulent images and a discriminator to distinguish these images from genuine payment data. This adversarial training enhances the model’s ability to identify anomalies in real-world scenarios.
The dataset used comprises 10,000 images, split evenly between authentic payment images and AI-generated deepfake samples. Real-world images were sourced from platforms like Google Open Images, Kaggle, and Alipay, while deepfake samples were generated using advanced GAN architectures, such as StyleGAN and DeepFake. By incorporating diverse age groups, genders, and ethnicities, the dataset ensures comprehensive training and testing for the proposed system.
The study’s results highlight the efficacy of the GAN-based model in distinguishing legitimate transactions from fraudulent deepfakes. The system achieved a detection accuracy of 96.2%, with high precision (95.5%) and recall (96.8%) rates. The F1-score, which balances precision and recall, stood at 96.1%, reflecting the model’s robustness in minimizing false positives while effectively identifying fraudulent activities.
The model’s Area Under the Curve (AUC) of 98.2% further underscores its reliability in various decision-making thresholds, making it a significant advancement over traditional fraud detection systems. These metrics illustrate the model’s capability to generalize across diverse scenarios, ensuring effective fraud prevention in real-world applications.
Innovative techniques in model design
The success of the GAN-based model stems from its innovative design, which integrates advanced features to enhance detection capabilities. The generator produces increasingly realistic fraudulent images, while the discriminator iteratively refines its ability to differentiate between real and fake data. This adversarial process results in a balanced and highly accurate detection system.
Additionally, the research incorporates cutting-edge optimization techniques, such as the Adam optimizer, and employs a cross-entropy loss function to fine-tune the model over 10,000 training iterations. These methods ensure that both the generator and discriminator achieve optimal performance, enabling the model to identify even the most subtle manipulations in payment images.
Applications and future directions
The GAN-based framework presents transformative possibilities for strengthening digital payment security in an era of increasing cyber threats. While its primary focus is on detecting deepfake-driven fraud, its design and adaptability enable applications beyond this specific domain. For instance, the model can be extended to identify manipulated documents, counterfeit IDs, and synthetic identities, all of which are growing concerns in online transactions and identity verification processes. These capabilities make the framework invaluable for sectors such as e-commerce, banking, and government services that rely heavily on secure digital operations.
The scalability of the GAN-based framework is another critical advantage. By integrating seamlessly with existing payment systems, the model can provide real-time fraud detection, empowering financial institutions and online merchants to identify and block fraudulent transactions before they occur. This real-time capability is especially crucial in high-frequency transaction environments, such as global payment platforms, where even a slight delay could lead to significant losses.
However, the research also highlights challenges that must be addressed to fully realize the potential of this technology. Edge cases, such as highly sophisticated deepfakes or transactions involving minimal data, pose a significant hurdle. For example, detecting fraud in scenarios where visual data is sparse or ambiguous requires enhanced model capabilities to generalize across diverse conditions. Future iterations of the framework could address these complexities by incorporating multimodal data sources.
The integration of voice recognition, behavioral biometrics, and geolocation data into the model offers a promising avenue for enhancing its versatility. Voice recognition could help identify mismatches in user authentication processes, while behavioral biometrics, such as typing patterns or device usage habits, could add an additional layer of verification. Geolocation data, combined with transactional metadata, could detect anomalies that indicate fraudulent activity, such as purchases made from unexpected locations.
Moreover, deploying the framework in live payment systems introduces the challenge of balancing accuracy with latency. Real-time deployment requires optimization to ensure rapid detection without compromising the user experience. The development of lightweight architectures or hybrid models that combine the GAN-based framework with other AI techniques could provide a solution to this trade-off.
Adversarial attacks, another critical area for future research, represent a significant threat to the framework's reliability. Sophisticated attackers may attempt to exploit vulnerabilities in the GAN-based system, crafting adversarial examples to evade detection or disrupt operations. Addressing these threats will require robust defenses, such as adversarial training and real-time anomaly detection mechanisms, to fortify the system against evolving attack strategies.
Looking ahead, collaboration between researchers, industry stakeholders, and policymakers will be essential to accelerate the adoption of this technology. Establishing standardized protocols for fraud detection and creating shared datasets for benchmarking could drive innovation while ensuring that security measures are uniformly effective across platforms. The potential for the GAN-based framework to safeguard digital payments extends beyond technology - it represents a step toward building trust in an increasingly digital economy.
- FIRST PUBLISHED IN:
- Devdiscourse