Protecting identities with unified AI: A breakthrough in face recognition
Face recognition technology has significantly evolved, reshaping industries such as security, healthcare, and personalized retail. However, its wide adoption has introduced vulnerabilities, particularly in the form of physical spoofing attacks (e.g., printed images, 3D masks) and digital attacks (e.g., deepfakes, adversarial noise).
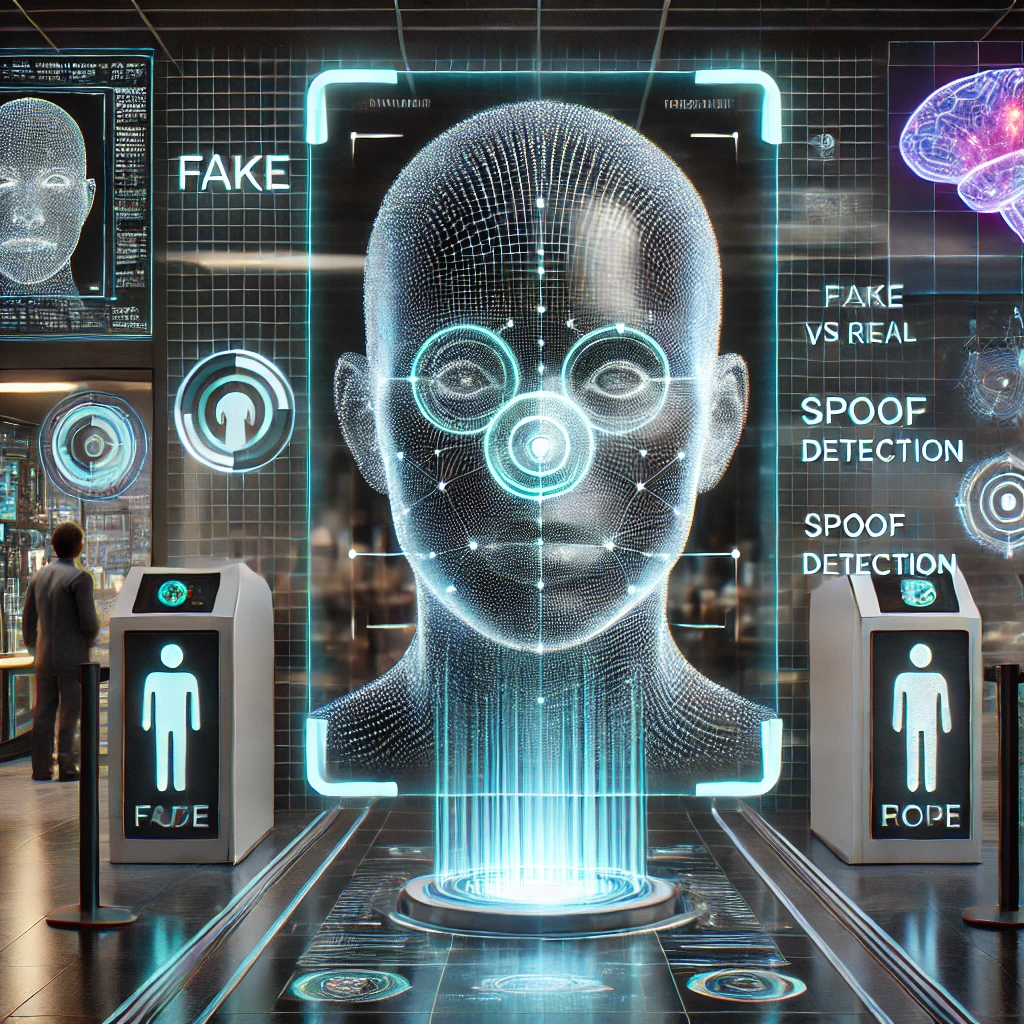
Face recognition technologies, long hailed as a breakthrough in secure identity verification, face evolving threats such as physical spoofing and sophisticated digital attacks like deepfakes. These vulnerabilities demand innovative solutions that not only enhance accuracy but also address these dual challenges under a unified framework.
In their pioneering research titled "Unified Face Matching and Physical-Digital Spoofing Attack Detection," authors Arun Kunwar and Ajita Rattani from the Department of Computer Science and Engineering, University of North Texas, present an innovative framework designed to address significant challenges in face recognition and spoof detection systems. Available on arXiv, the study introduces a unified model leveraging cutting-edge technologies like the Swin Transformer and HiLo attention mechanisms, aiming to optimize both face matching and spoof attack detection under a single framework.
This research is particularly groundbreaking in its unified approach, bridging the gap between face recognition and the detection of both physical and digital spoofing attacks, a dual threat that undermines traditional biometric systems.
Challenges of face recognition systems
Face recognition technology has significantly evolved, reshaping industries such as security, healthcare, and personalized retail. However, its wide adoption has introduced vulnerabilities, particularly in the form of physical spoofing attacks (e.g., printed images, 3D masks) and digital attacks (e.g., deepfakes, adversarial noise).
Traditional systems treat face recognition and spoof detection as separate tasks, requiring distinct models that increase computational complexity and hinder scalability. This limitation is especially pronounced in resource-constrained environments like mobile devices.
The study addresses this inefficiency through a unified model that integrates these tasks, ensuring enhanced performance and streamlined operations.
Swin Transformer Backbone
The unified model employs the Swin Transformer, renowned for its hierarchical architecture and global self-attention mechanisms. Unlike traditional Convolutional Neural Networks (CNNs), the Swin Transformer effectively captures both local and global image features, overcoming challenges such as variations in lighting, pose, and occlusion. This feature extraction capability is critical for accurate face matching.
HiLo Attention and Unified Attack Detection (UAD) Module
To enhance the detection of spoof attacks, the study introduces a Unified Attack Detection (UAD) module. The UAD module employs HiLo attention mechanisms to simultaneously analyze high-frequency features (e.g., textures and artifacts) and low-frequency contextual structures. This dual focus strengthens the model’s ability to detect subtle spoofing cues, from local manipulations to global distortions, enabling robust detection of both physical and digital spoof attacks.
Augmentation for realistic spoof detection
To enhance the model's capability to detect spoofing attacks effectively, the researchers developed advanced augmentation techniques that replicate real-world scenarios. One such technique, Simulated Physical Spoofing Clues (SPSC), focuses on mimicking the characteristics of physical attacks, such as color distortions, texture inconsistencies, and artifacts commonly associated with print and replay attacks. This augmentation ensures that the model can identify subtle visual anomalies that are typical in physical spoof attempts.
On the other hand, Simulated Digital Spoofing Clues (SDSC) generates synthetic forgery artifacts that represent digital manipulations, including deepfake features and attribute alterations. By incorporating these synthetic clues, the model becomes adept at detecting advanced digital forgeries that exploit generative technologies.
Together, these augmentations significantly enhance the model’s robustness, enabling it to handle a broad spectrum of spoofing methods. Importantly, this approach equips the framework to tackle unseen attack types, a critical requirement for its deployment in dynamic, real-world environments where new threats continuously emerge.
Experimental excellence: Datasets and evaluation
The study conducted a comprehensive evaluation of the proposed unified model using a diverse range of datasets to validate its effectiveness in both face recognition and spoof detection. For face recognition, the model was tested on the widely recognized CASIA-WebFace dataset, while spoof detection evaluations utilized datasets such as FaceForensics++, SiW-Mv2, and the Diverse Fake Face Dataset (DFFD).
The findings were remarkable, highlighting the model’s ability to achieve 99.43% accuracy in face matching on the FaceForensics++ dataset, a performance on par with state-of-the-art face recognition systems. In terms of spoof detection, the model demonstrated its robustness by achieving 97.2% accuracy in detecting deepfakes and 86.8% accuracy in identifying physical spoof attacks.
These results emphasize the model’s versatility and reliability, particularly when dealing with cross-dataset evaluations and unknown attack scenarios. Such consistent performance across different datasets underscores the framework’s potential for real-world applications, where systems must navigate diverse and unpredictable challenges.
Applications and future implications
The unified model proposed by the researchers has transformative potential for enhancing biometric security across various domains. Its lightweight architecture and high performance make it an ideal candidate for real-world deployments where both accuracy and efficiency are critical. In mobile authentication, the model can provide a secure and seamless unlocking experience without compromising speed, making it suitable for resource-constrained devices such as smartphones and tablets.
Similarly, in surveillance systems, the model’s robust ability to detect physical and digital spoofing attacks ensures the reliability of security infrastructure in sensitive environments like airports, banks, and government facilities. The healthcare sector, too, stands to benefit significantly, as the model enables accurate and secure patient identification in telemedicine and hospital management systems, reducing the risks of fraud or misidentification.
Looking ahead, the authors envision extending this unified framework to other biometric modalities, including fingerprint and iris recognition. This expansion could revolutionize the broader field of biometric security by providing a cohesive, efficient, and highly adaptable solution to address emerging challenges in identity verification and access control.
- FIRST PUBLISHED IN:
- Devdiscourse