The battle against misinformation: Novel AI-based approach detects fake news in real-time
Fake news is not a new phenomenon, but the speed and scale at which it spreads in the digital era are unprecedented. Social media platforms, designed to maximize engagement, often amplify false information through algorithms that prioritize virality over accuracy. This has led to misinformation influencing critical events such as elections, public health campaigns, and international relations.
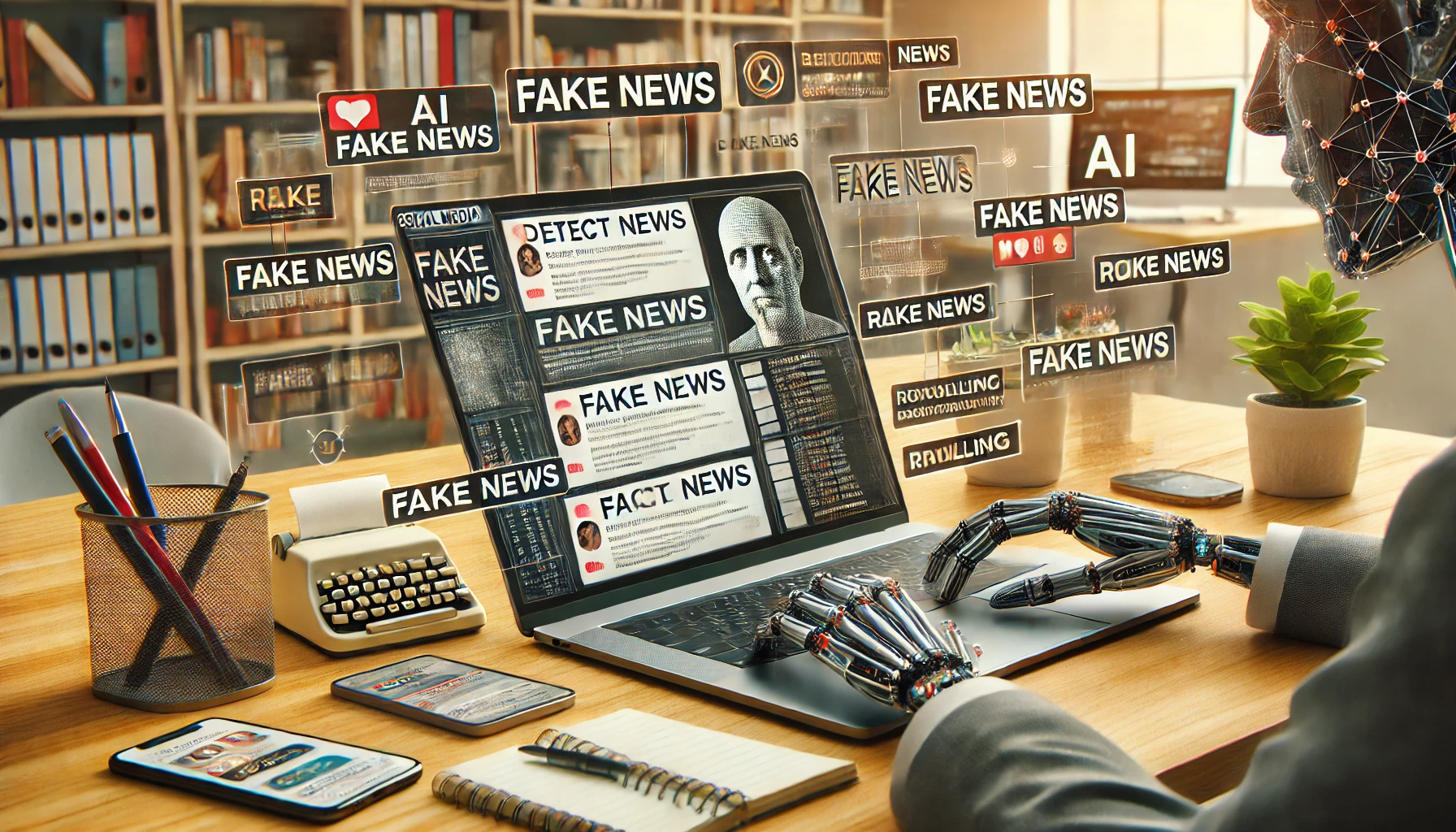
In today’s hyperconnected digital world, social media platforms like Facebook, Twitter, and Instagram have revolutionized how we consume and share information. While these platforms have democratized access to news and opinions, they have also inadvertently facilitated the proliferation of fake news - misinformation that undermines public trust, misguides individuals, and disrupts societies. From spreading false medical advice during pandemics to influencing political outcomes, fake news has emerged as a global threat.
A group of researchers has developed a groundbreaking solution to address this challenge. Their study, "Fake News Detection on Social Media Using Ensemble Methods," proposes a sophisticated framework for detecting fake news by leveraging ensemble machine learning and deep learning techniques. This research, published in Computers, Materials & Continua, 81(3), 4525–4549, introduces a robust methodology that combines multiple feature extraction and selection techniques to enhance the accuracy and reliability of fake news detection.
Authored by Muhammad Ali Ilyas and a team of experts from renowned institutions across Pakistan, Korea, and Saudi Arabia, the study sheds light on the power of artificial intelligence to combat misinformation and offers a roadmap for deploying scalable solutions in real-world scenarios.
The ubiquitous problem of fake news
Fake news is not a new phenomenon, but the speed and scale at which it spreads in the digital era are unprecedented. Social media platforms, designed to maximize engagement, often amplify false information through algorithms that prioritize virality over accuracy. This has led to misinformation influencing critical events such as elections, public health campaigns, and international relations.
The study emphasizes that existing detection methods often fall short due to the noisy, unstructured nature of data on social media. Many systems rely on singular approaches - either linguistic analysis or metadata assessment - making them ill-equipped to handle high-dimensional datasets or adapt to new and diverse content types. This underscores the need for a more holistic and adaptable approach, which the researchers aim to provide through their ensemble framework.
An innovative multi-faceted approach
At the heart of the proposed solution is the integration of advanced feature extraction and selection methods. The study uses techniques such as Count Vectorizer, Bag of Words (BoW), GloVe, Word2Vec, and TF-IDF to transform raw text data into structured numerical representations. Each of these methods plays a unique role:
- Count Vectorizer and BoW focus on simple word frequency representations.
- TF-IDF (Term Frequency-Inverse Document Frequency) measures the importance of words relative to their frequency across documents.
- GloVe and Word2Vec add a layer of semantic understanding by capturing word relationships and contexts within a vector space.
To enhance the reliability of the extracted features, the researchers employ feature selection techniques such as Information Gain, Chi-Square, Principal Component Analysis (PCA), and Document Frequency. These methods help eliminate irrelevant or redundant features, improving computational efficiency and model performance.
The core innovation lies in the use of ensemble models. By combining machine learning classifiers like Random Forest and Support Vector Machines with deep learning architectures such as Convolutional Neural Networks (CNNs) and Long Short-Term Memory networks (LSTMs), the framework achieves remarkable accuracy. This layered approach leverages the strengths of different models, ensuring robustness and adaptability across datasets.
The ensemble model’s performance was tested on three distinct datasets, achieving accuracy rates of 97%, 99%, and 98%. These results underscore its effectiveness in handling diverse and complex data. By incorporating multiple feature extraction and selection techniques, the framework addresses challenges such as data sparsity and contextual ambiguity, which often hinder traditional detection systems.
For instance, the combination of Random Forest and Support Vector Machine consistently outperformed other models, demonstrating superior precision and recall metrics. This highlights the value of ensemble methods in balancing various aspects of performance, such as minimizing false positives while maximizing detection accuracy.
Real-world applications and implications
The implications of this research extend far beyond academia. In a world where misinformation can have real-world consequences, the ability to detect fake news accurately and efficiently is vital for governments, social media companies, and independent fact-checking organizations. The study’s findings provide actionable insights into how AI can be deployed to monitor and filter content in real-time, potentially preventing the viral spread of harmful misinformation.
Moreover, the framework’s scalability makes it suitable for integration into existing platforms. Social media companies can use such models to flag or downrank suspicious content, while governments can employ them to counter misinformation campaigns targeting public health, national security, or elections.
Expanding the scope
While the proposed framework represents a significant leap forward, the authors highlight areas for further exploration. The integration of multimodal data - such as text combined with images, videos, or metadata - could improve the system’s ability to detect misinformation across different formats. For example, pairing textual analysis with image recognition could help identify fake headlines attached to unrelated or manipulated images, a common tactic in misinformation campaigns.
The researchers also emphasize the importance of real-time processing capabilities. As misinformation spreads rapidly, future systems must be capable of identifying and mitigating fake news almost instantaneously. Additionally, ethical considerations, such as ensuring that detection systems do not infringe on free speech or privacy, must remain a priority.
To sum up, by combining the power of machine learning, deep learning, and advanced feature engineering, the proposed ensemble framework represents a powerful tool in the battle to preserve digital integrity.
- FIRST PUBLISHED IN:
- Devdiscourse