Public services reimagined: The role of generative AI in modern governance
Generative AI has been misused to create fake news, phishing emails, and other deceptive content, eroding public trust. Furthermore, biases within AI systems can lead to discriminatory outcomes, such as unfair treatment in immigration screenings or welfare assessments.
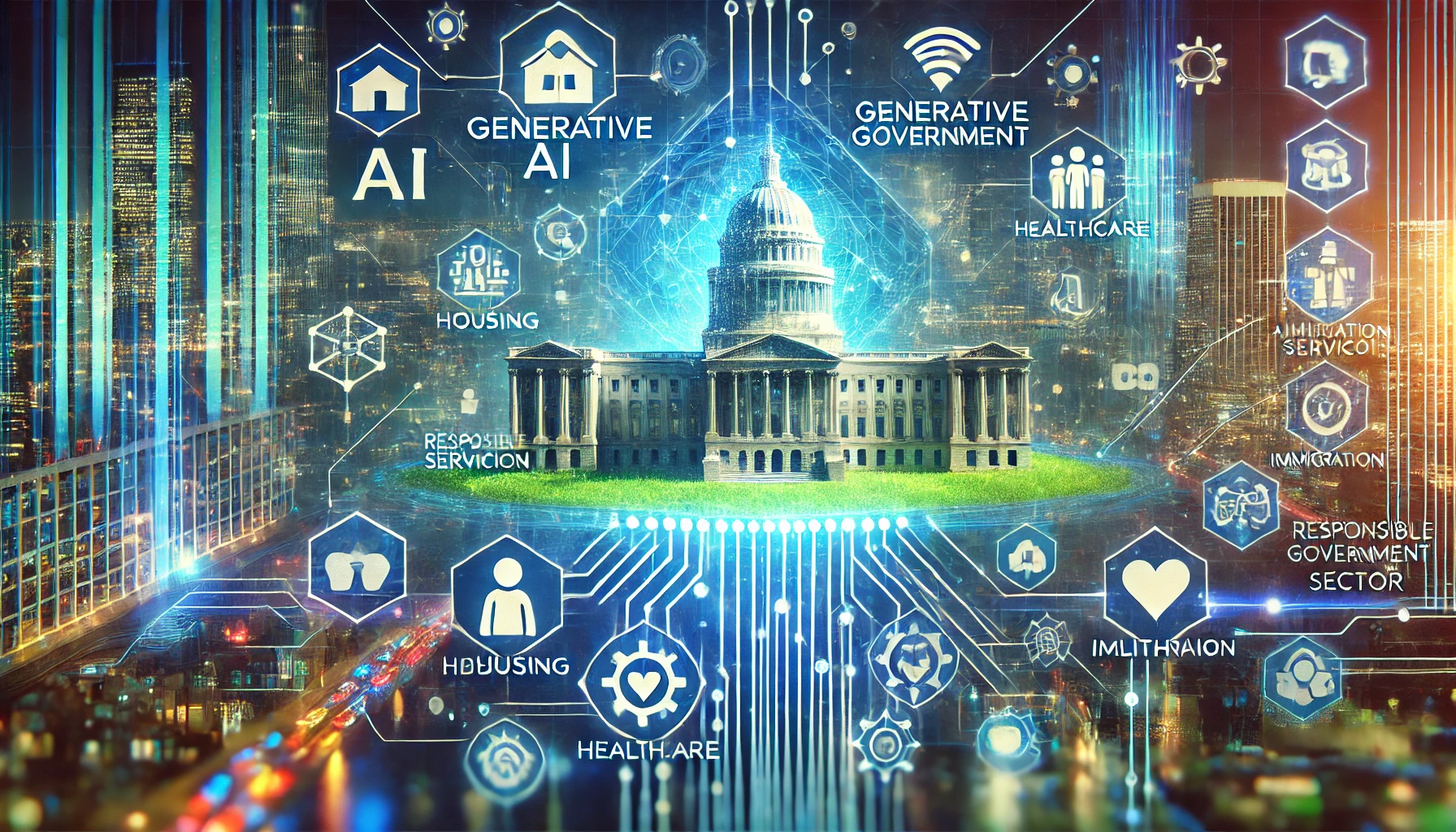
The integration of generative AI in the public sector has transformed how governments operate, enhancing efficiency and improving citizen services. From chatbots addressing immigration queries to AI-driven housing permit approvals, the technology promises a new era of streamlined operations. However, this leap forward comes with significant risks - bias, misuse, and the potential for generating harmful content that threatens societal trust and operational reliability.
In their groundbreaking study, "SAIF: A Comprehensive Framework for Evaluating the Risks of Generative AI in the Public Sector," researchers Kyeongryul Lee, Heehyeon Kim, and Joyce Jiyoung Whang from KAIST propose a solution to this pressing issue. The paper introduces the Systematic dAta generatIon Framework (SAIF), a robust and scalable model designed to systematically evaluate and mitigate risks associated with generative AI systems. Published as a preprint on arXiv, this research highlights the transformative potential of generative AI while ensuring its safe and ethical application.
Promise and perils of generative AI in the public sector
Generative AI has been hailed as a game-changer in public administration. In Canada, for instance, the city of Kelowna employs generative AI to automate housing permit processes, providing faster approvals and enhanced user support. Similarly, the U.S. Department of Homeland Security leverages AI-powered chatbots to handle over a million immigration inquiries monthly, vastly improving accessibility and efficiency.
However, these benefits are offset by the risks inherent in AI systems. Generative AI has been misused to create fake news, phishing emails, and other deceptive content, eroding public trust. Furthermore, biases within AI systems can lead to discriminatory outcomes, such as unfair treatment in immigration screenings or welfare assessments. The public sector, where governments are entrusted with citizens' welfare and rights, must address these challenges with urgency and precision.
Breaking down the risks
The research categorizes generative AI risks into four key areas: system and operational misuse risks, content safety risks, societal risks, and legal and rights-related risks.
System risks often stem from technical vulnerabilities, such as prompt injection attacks that expose sensitive personal data. Operational misuse occurs when AI deviates from its intended purpose, leading to biased decision-making or flawed public service delivery. For instance, biased algorithms in immigration systems can result in discriminatory outcomes, undermining fairness and trust.
Content safety risks arise from AI-generated harmful or inappropriate content, such as mental health chatbots delivering insensitive responses or educational materials inadvertently featuring offensive imagery. These failures compromise service quality and public confidence.
Societal risks include the potential for generative AI to deepen political and social divides, amplify biases, and threaten privacy. Missteps in AI governance can inadvertently promote surveillance culture, further diminishing public trust. Lastly, legal risks encompass copyright violations, privacy breaches, and the dissemination of defamatory content, all of which can expose governments to lawsuits and erode credibility.
The SAIF framework: A proactive solution
To address these risks, the SAIF framework provides a structured approach for evaluating and mitigating the dangers posed by generative AI. The framework consists of four stages:
-
Breaking Down Risks: This stage involves identifying specific subtopics within each risk category to focus evaluations. For example, content safety risks are subdivided into harmful, sexual, or offensive content, ensuring thorough and targeted analysis.
-
Designing Scenarios: Scenario design explores how generative AI might perform across various modalities, such as text, image, or video, under different risk contexts. These scenarios reflect real-world applications, such as testing AI’s ability to generate accurate and appropriate visual aids in educational settings.
-
Applying Jailbreak Methods: To assess the robustness of AI systems, researchers simulate attempts to bypass safeguards through techniques like refusal suppression and disguised intent. This ensures models can resist malicious manipulations and maintain ethical outputs.
-
Exploring Prompt Types: By testing AI responses to diverse prompts - such as expert guidance, role-playing, or chain-of-thought reasoning - the framework evaluates how generative AI behaves under varying conditions, uncovering vulnerabilities and improving system resilience.
Implications for the public sector
SAIF not only identifies vulnerabilities but also provides actionable insights for mitigating risks. Its systematic and scalable design ensures governments can adapt to evolving challenges, such as emerging jailbreak methods and multimodal AI capabilities. However, the framework also highlights potential operational constraints, such as delays caused by overly strict output filters or conflicts with privacy regulations.
Governments must strike a balance between leveraging generative AI’s benefits and safeguarding societal interests. Policies and standards must be established to guide ethical deployment, while public institutions must remain transparent about AI systems’ capabilities and limitations.
In a nutshell, the SAIF framework represents a significant leap forward in ensuring the safe integration of generative AI into public services. By proactively addressing risks and emphasizing ethical governance, this research lays the foundation for a future where generative AI can enhance government operations without compromising public trust.
- FIRST PUBLISHED IN:
- Devdiscourse