AI takes the wheel: Leveraging LLMs for efficient and adaptive public transportation
The integration of LLMs into public transportation systems has transformative implications. Their ability to analyze historical and real-time data allows for smarter route planning and scheduling, reducing passenger wait times and improving service reliability. In addition, LLMs can significantly enhance communication with passengers, providing real-time updates, multilingual support, and personalized travel assistance.
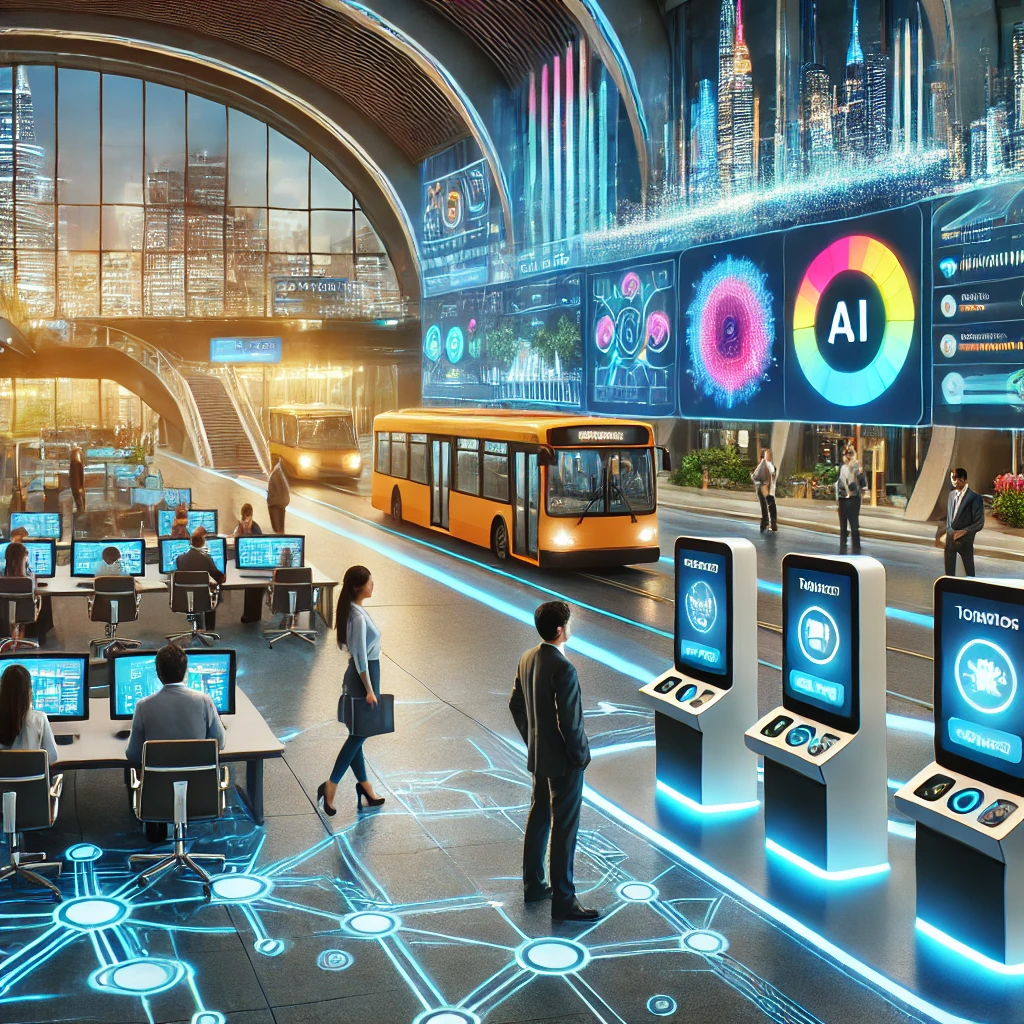
Public transportation systems are the backbone of urban mobility, providing millions of people with access to essential services and opportunities. However, these systems often face challenges such as fluctuating demand, inefficient resource allocation, and gaps in passenger communication. With cities rapidly expanding, these issues are becoming increasingly complex, necessitating innovative solutions.
In their groundbreaking study, "Exploring the Potential of Large Language Models in Public Transportation: San Antonio Case Study," researchers Ramya Jonnala, Gongbo Liang, Jeong Yang, and Izzat Alsmadi from Texas A&M University-San Antonio investigate the transformative role of Large Language Models (LLMs) like GPT in addressing these challenges. Accepted for presentation at the AAAI 2025 Workshop on AI for Urban Planning, the study demonstrates how AI can optimize transit operations, enhance user experience, and drive data-informed decision-making.
Harnessing LLMs for public transit systems
Large Language Models, known for their remarkable ability to process natural language and analyze vast datasets, are emerging as powerful tools for tackling real-world problems. The researchers explore the potential of LLMs to transform San Antonio’s public transportation system by leveraging data from the General Transit Feed Specification (GTFS). The study focuses on three key areas: optimizing route planning and scheduling, improving passenger communication, and enhancing operational efficiency.
San Antonio, one of the fastest-growing urban areas in the United States, provides a compelling case study. The city's rapid population growth has placed increased pressure on its transit system, making innovative approaches a necessity. LLMs, with their ability to process complex datasets and generate accurate, context-aware responses, offer promising solutions to meet these demands while improving passenger satisfaction and reducing environmental impact.
To evaluate the effectiveness of LLMs in public transit applications, the study conducted five experiments. These focused on two core tasks: understanding transit information and retrieving specific data.
Understanding Transit Information
This task assessed the ability of pre-trained LLMs to comprehend and respond to transportation-related questions. Multiple-choice questions (MCQs) were meticulously crafted from GTFS data, spanning six categories such as file structure, data attributes, and common reasoning. The experiments tested the models' abilities to handle ambiguity, identify relationships in data, and provide accurate answers. GPT-4 consistently outperformed GPT-3.5-turbo, achieving high accuracy in categories like Term Definitions and Attribute Mapping, with scores exceeding 95% in some cases.
Information Retrieval
The second task examined whether LLMs could extract and integrate information from GTFS datasets to answer complex, real-world transit queries. Using a subset of San Antonio’s GTFS feed, the researchers developed 80 short-answer questions requiring data lookups, filtering, and integration across multiple files. The findings revealed that while LLMs excelled in simple retrieval tasks (achieving up to 90.48% accuracy), their performance declined significantly when handling more complex queries involving data integration.
The study revealed several critical insights into the potential and limitations of LLMs in public transit systems. LLMs demonstrated strong performance in simpler tasks, excelling in straightforward reasoning and data retrieval, which positions them as valuable tools for real-time passenger assistance, such as providing schedule updates, answering route queries, and offering travel recommendations.
However, performance gaps became apparent when queries required integrating information across multiple datasets, highlighting the need for enhanced prompt engineering and fine-tuning to improve LLMs’ ability to handle complex, multi-step tasks. Despite challenges, GPT-4 exhibited greater resilience in navigating ambiguous scenarios compared to GPT-3.5-turbo, demonstrating its robustness even when datasets included unclear options like “none of these.” Nonetheless, occasional inconsistencies in responses, particularly for complex or nuanced queries, emphasize the importance of refining LLM outputs to ensure reliability in critical applications such as public transportation.
Transformative implications for urban transit
The integration of LLMs into public transportation systems has transformative implications. Their ability to analyze historical and real-time data allows for smarter route planning and scheduling, reducing passenger wait times and improving service reliability. In addition, LLMs can significantly enhance communication with passengers, providing real-time updates, multilingual support, and personalized travel assistance.
From an operational perspective, LLMs can optimize resource allocation by analyzing demand patterns and identifying inefficiencies. This has the potential to reduce costs, minimize environmental impact, and make transit systems more adaptive to changing urban dynamics. For diverse urban populations, the multilingual capabilities of LLMs also promote inclusivity and accessibility, ensuring equitable access to transit information.
Addressing challenges and the road ahead
While the study highlights the transformative potential of LLMs in public transportation, it also underscores key challenges that must be addressed for their effective deployment. Improving performance in complex queries requiring multi-step reasoning and data integration remains a priority, alongside ensuring data privacy and mitigating biases to support ethical use.
Testing LLMs on larger, diverse transit datasets is essential to validate their scalability and robustness in real-world scenarios. Additionally, developing models capable of adapting to dynamic transit conditions, such as traffic disruptions or weather events, will significantly enhance their practical utility. By addressing these challenges, LLMs have the potential to create smarter, more adaptive, and passenger-focused transit systems in urban environments worldwide.
- FIRST PUBLISHED IN:
- Devdiscourse