Generalizing fairness: Advancing deepfake detection for diverse populations
The study sets a new standard for fairness in AI by addressing systemic biases that have long plagued deepfake detection systems. Beyond technical improvements, this framework represents a significant step toward creating ethical and responsible AI technologies that prioritize inclusivity and equity.
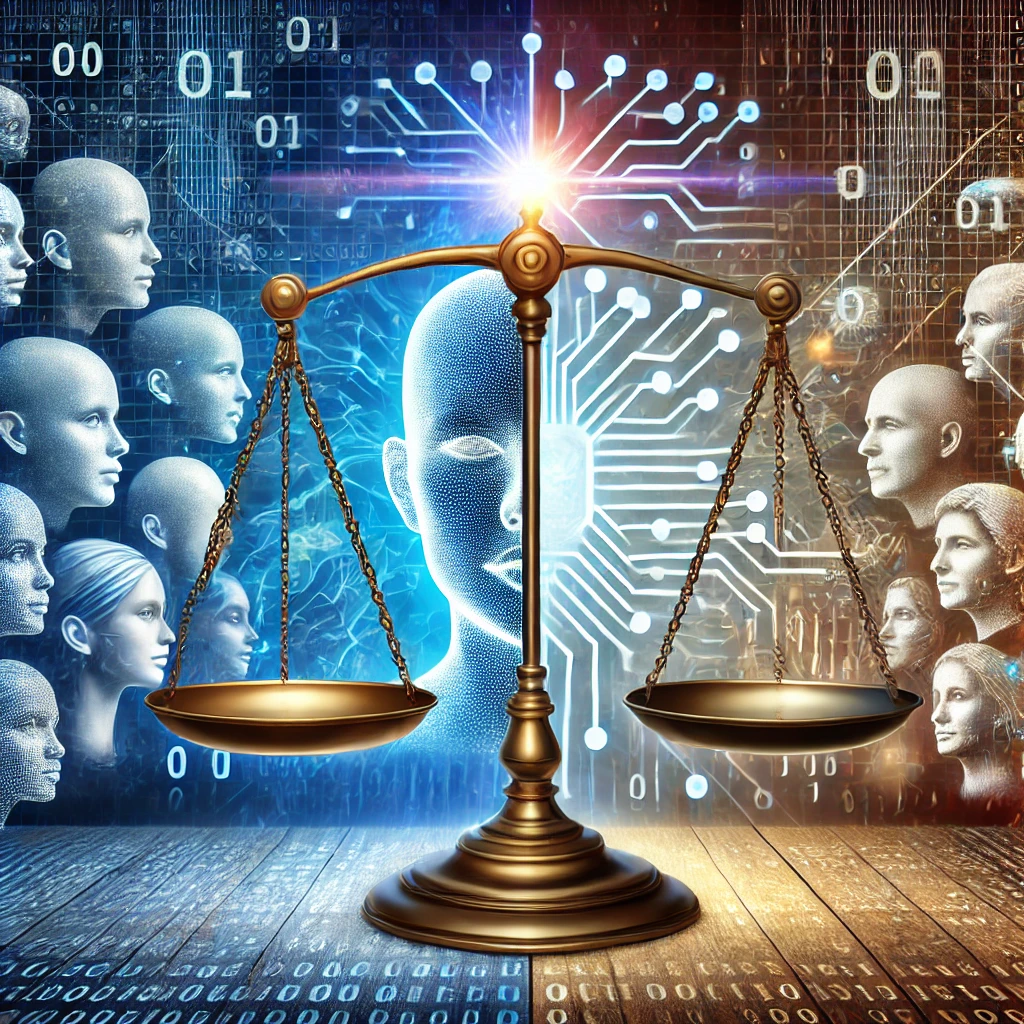
Deepfake technology has emerged as a powerful tool for media manipulation. By leveraging advanced machine learning algorithms, deepfakes enable the creation of hyper-realistic but fraudulent digital content, including videos, images, and audio. While detection systems have advanced in accuracy, they often fail to ensure fairness, with performance disparities across demographic groups - favoring lighter-skinned or male individuals over others. In the study "Data-Driven Fairness Generalization for Deepfake Detection", published by researchers from Florida State University and Oklahoma State University, a significant breakthrough is presented in addressing the fairness and generalization challenges in deepfake detection. This study introduces a novel framework that ensures fairness while maintaining high detection accuracy across diverse demographic groups.
Challenges in deepfake detection and fairness
Current deepfake detection systems often struggle with biases embedded in their training datasets. These biases result in uneven detection performance across demographics, disproportionately affecting underrepresented groups such as individuals with darker skin tones or non-Western ethnicities. Studies reveal that such systems tend to show higher detection accuracy for lighter-skinned individuals, leaving others more vulnerable to malicious exploitation.
The problem intensifies when detection models are tested on new datasets with different characteristics. While models may generalize detection accuracy to new datasets, fairness often fails to transfer, creating significant disparities in how systems perform for different demographic groups. This lack of fairness undermines the credibility and trustworthiness of AI systems, especially in critical applications like content moderation, fraud prevention, and media authentication.
The proposed framework: A three-pronged approach
The researchers propose a novel framework to address these challenges, introducing a comprehensive data-driven approach that combines synthetic data generation, multi-task learning, and optimization techniques to enhance both fairness and generalization.
Synthetic Data Balancing
The framework employs a technique based on Self-Blended Images (SBI) to create synthetic datasets that balance demographic representation. By generating diverse samples across demographic groups and combining real and fake images in equal proportions, this method eliminates imbalances in the training dataset. For example, synthetic images are created by blending transformations like scaling, rotation, or color adjustments with real images, ensuring all demographic groups are equally represented in training.
This balancing strategy ensures that the model learns from a representative dataset, addressing one of the root causes of bias in deepfake detection.
Multi-Task Learning Architecture
To further improve fairness, the framework uses a multi-task learning approach. The architecture includes:
- A Real/Fake Classification Head: Focused on detecting whether an image is real or fake, this head captures subtle artifacts and inconsistencies in deepfakes, such as irregular lighting or facial blurring.
- A Demographic Classification Head: This head predicts the demographic group of the input image. By incorporating demographic-specific features into the model, it ensures that the detection process accounts for variations across groups, making the system more equitable.
This dual-task architecture allows the model to simultaneously optimize for accuracy and demographic fairness, addressing biases in both intra-dataset and cross-dataset scenarios.
Sharpness-Aware Minimization (SAM)
The framework incorporates Sharpness-Aware Minimization (SAM), an optimization technique that flattens the loss landscape, making the model more robust to variations in input data. SAM identifies parameter spaces that minimize both loss and sensitivity to perturbations, ensuring consistent performance across diverse and unseen datasets. This approach is particularly valuable for improving fairness generalization in cross-dataset evaluations, where models encounter new demographic distributions and forgery techniques.
Experimental Validation: A New Benchmark in Fairness
The framework was rigorously tested using both intra-dataset and cross-dataset evaluations. By leveraging benchmark datasets such as FaceForensics++, Deepfake Detection Challenge (DFDC), and Celeb-DF, the researchers demonstrated the framework’s ability to outperform state-of-the-art methods in both accuracy and fairness.
Intra-Dataset Testing
When trained and tested on the same dataset distribution, the framework showed remarkable fairness improvements:
- Gender disparities in detection accuracy were reduced to 0.12%, compared to up to 3.87% in baseline methods.
- Racial disparities were minimized to 0.71%, a significant improvement over disparities of up to 5.28% in existing models.
These results underscore the effectiveness of the synthetic data balancing and multi-task learning components in creating equitable detection systems.
Cross-Dataset Testing
In cross-dataset evaluations, where the model was trained on one dataset and tested on entirely new distributions, the framework achieved even greater success:
- On the Celeb-DF dataset, gender accuracy disparities were reduced to 15.47%, compared to 40.66% in baseline methods.
- Racial disparities were minimized to 11.54%, compared to disparities of 26.41% in existing approaches.
These findings highlight the framework’s robustness in maintaining fairness across diverse datasets, making it a viable solution for real-world applications where data diversity is inevitable.
Broader Implications for AI and Society
The study sets a new standard for fairness in AI by addressing systemic biases that have long plagued deepfake detection systems. Beyond technical improvements, this framework represents a significant step toward creating ethical and responsible AI technologies that prioritize inclusivity and equity. The implications extend to various domains, including:
- Media Authentication: Ensuring fair detection of manipulated content across global audiences.
- Content Moderation: Reducing bias in identifying harmful media on social platforms.
- Legal and Forensic Applications: Providing equitable tools for identifying deepfakes in legal investigations.
The use of synthetic data balancing and demographic-aware learning also opens doors for similar fairness-focused innovations in other AI domains, such as facial recognition, natural language processing, and predictive analytics.
Limitations and future directions
While the proposed framework demonstrates significant advancements, it is not without limitations. One major challenge is its dependence on datasets with detailed demographic annotations, which are often difficult to obtain and may fail to fully capture the diversity of real-world populations. Another limitation lies in the trade-offs between fairness and accuracy, as enhancing fairness can sometimes lead to a slight reduction in overall detection performance, underscoring the need for further optimization.
Future research could address these challenges by exploring automated methods for demographic annotation, developing larger and more diverse datasets, and refining techniques to balance fairness and accuracy without compromise. Additionally, extending this approach to other types of media manipulation, such as video deepfakes and audio forgeries, could broaden its applicability and further enhance its impact.
- READ MORE ON:
- deepfake detection
- deepfake
- tech fairness
- tech bias
- AI bias
- Trustworth AI
- AI ethics
- FIRST PUBLISHED IN:
- Devdiscourse