AI’s cognitive leap: LLMs are thinking more like humans than ever before
The path forward will be marked by collaboration between fields like neuroscience, computer science, and cognitive psychology. Together, they will continue to push the boundaries of what AI can achieve, paving the way for a future where artificial and human intelligence converge seamlessly.
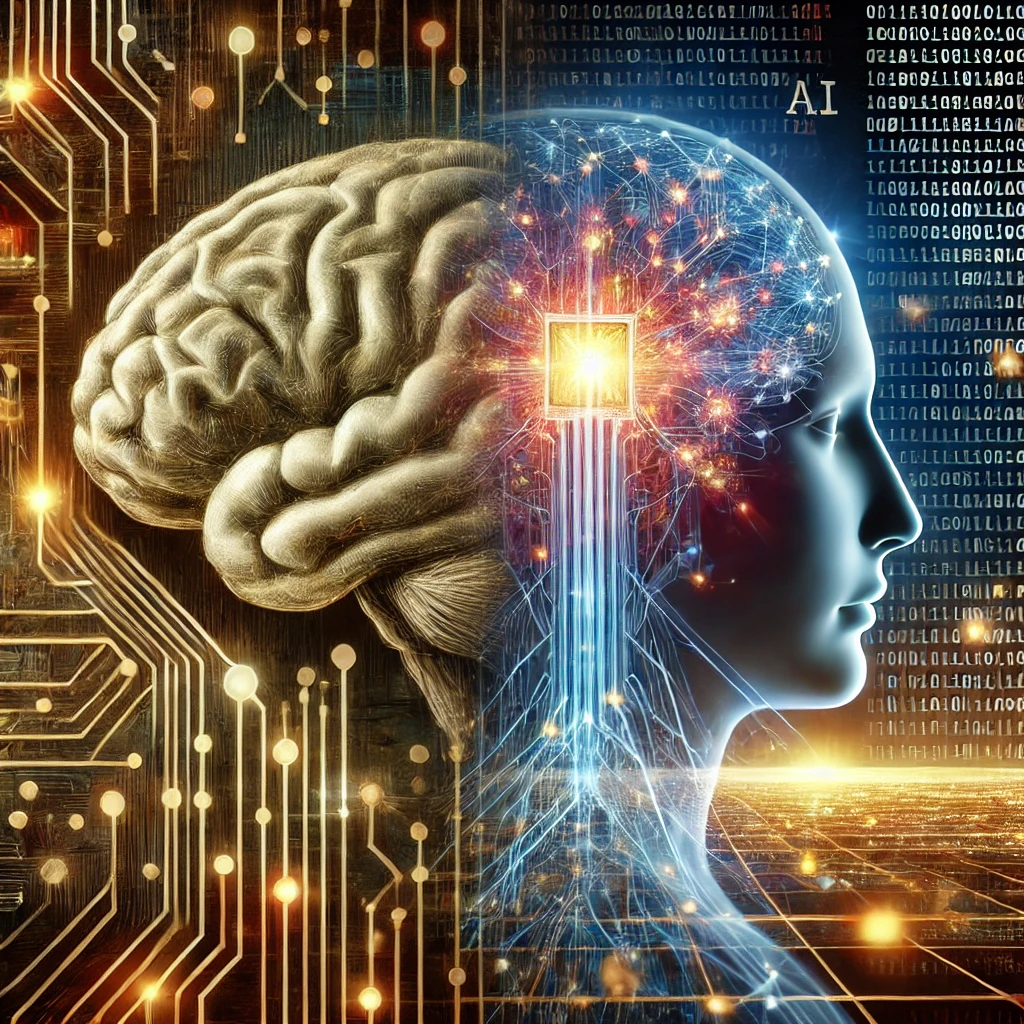
The rapid advancements in artificial intelligence (AI) have sparked discussions about how closely AI systems, particularly large language models (LLMs), can emulate human cognition. From chatbots capable of holding natural conversations to tools that generate contextually rich content, the parallels between AI's language capabilities and the human brain's processing mechanisms are becoming increasingly evident. But what does it truly mean for AI to mimic the brain's language comprehension, and how far have we come in bridging this gap?
A groundbreaking study, "Contextual Feature Extraction Hierarchies Converge in Large Language Models and the Brain", conducted by researchers from Columbia University and The Feinstein Institutes for Medical Research, dives into these questions. Available on arXiv, the research explores how AI systems align with the brain’s hierarchical processing pathways when it comes to understanding language. Using neural recordings from human brains alongside performance data from cutting-edge LLMs, the study uncovers striking similarities in how both systems process language contextually and hierarchically.
Bridging AI and Neuroscience
The study investigates how state-of-the-art LLMs, such as Mistral and LLaMA, compare to the brain’s mechanisms in understanding language. By analyzing 12 high-performance, open-source LLMs alongside neural recordings from eight neurosurgical patients, the researchers uncovered fascinating correlations between AI-generated embeddings and human brain activity. Notably, better-performing LLMs not only processed language more accurately but also aligned more closely with the brain’s hierarchical language processing pathways.
These insights offer a new lens to examine how LLMs evolve and refine language understanding, bridging the gap between artificial and human intelligence.
Hierarchical processing and context
Both LLMs and the human brain employ hierarchical pathways to decode language, progressing from simpler to more complex representations. The study found that high-performing LLMs mirror this approach. For instance, models like Mistral demonstrated peak brain alignment in earlier layers of processing, similar to how the brain processes speech through primary auditory regions before integrating higher-level linguistic features.
Lower-performing models, by contrast, required deeper layers to align with brain functions, reflecting inefficiencies in their feature extraction processes. This observation underscores the importance of optimizing AI architectures to emulate the brain's efficiency.
One of the study's key findings is the role of context in both AI and brain performance. High-performing LLMs excel in contextual understanding, integrating broader linguistic cues to achieve deeper alignment with the brain's language pathways. The ability to leverage extended contextual windows allows these models to capture nuances in language that mimic human cognition.
For instance, the brain’s ability to retain and integrate preceding sentences when processing a paragraph is reflected in the LLM's capacity to handle long-range dependencies. Models with superior contextual understanding not only performed better in language tasks but also achieved a closer neural resemblance to human cognitive processes.
Implications for AI and beyond
This convergence between LLMs and the brain has profound implications for the future of AI. By identifying shared computational strategies, the study offers a blueprint for designing more brain-like AI systems. Optimizing early-stage processing layers, enhancing contextual comprehension, and refining hierarchical architectures could lead to the development of models that approach human-like efficiency and adaptability.
Such insights could revolutionize not only natural language processing but also broader applications like multimodal AI, where integrating language, vision, and reasoning is crucial. Furthermore, the study raises the possibility of creating models that generalize across domains, moving closer to artificial general intelligence (AGI).
While the study focuses on language processing, its implications extend beyond linguistics. By using LLMs as computational analogs for the brain, researchers can gain new insights into human cognition. For instance, studying how LLMs handle ambiguities or syntactic complexities could inform our understanding of similar processes in the brain.
Moreover, the interplay between AI and neuroscience could lead to practical applications in medicine, such as designing AI systems that assist in diagnosing and treating neurological disorders by simulating brain functions.
Challenges and Opportunities
Despite its promise, aligning AI with human cognition is not without challenges. The brain is far more adaptive and complex, capable of dynamic learning and nuanced reasoning that current LLMs cannot fully replicate. However, this study sets the stage for iterative improvements, where each generation of LLMs incorporates more brain-inspired principles.
The research also invites broader questions about the ethical implications of creating brain-like AI systems. As these technologies advance, ensuring they are deployed responsibly and transparently will be critical.
The path forward will be marked by collaboration between fields like neuroscience, computer science, and cognitive psychology. Together, they will continue to push the boundaries of what AI can achieve, paving the way for a future where artificial and human intelligence converge seamlessly.
- FIRST PUBLISHED IN:
- Devdiscourse