Beyond the algorithms: Deep learning transforming financial landscapes
As the financial industry becomes increasingly data-driven, the integration of DL technologies will be essential to navigate complexities, mitigate risks, and unlock new opportunities. With a focus on collaboration, transparency, and innovation, deep learning holds the potential to revolutionize the financial landscape, ensuring resilience and inclusivity in an ever-changing global economy.
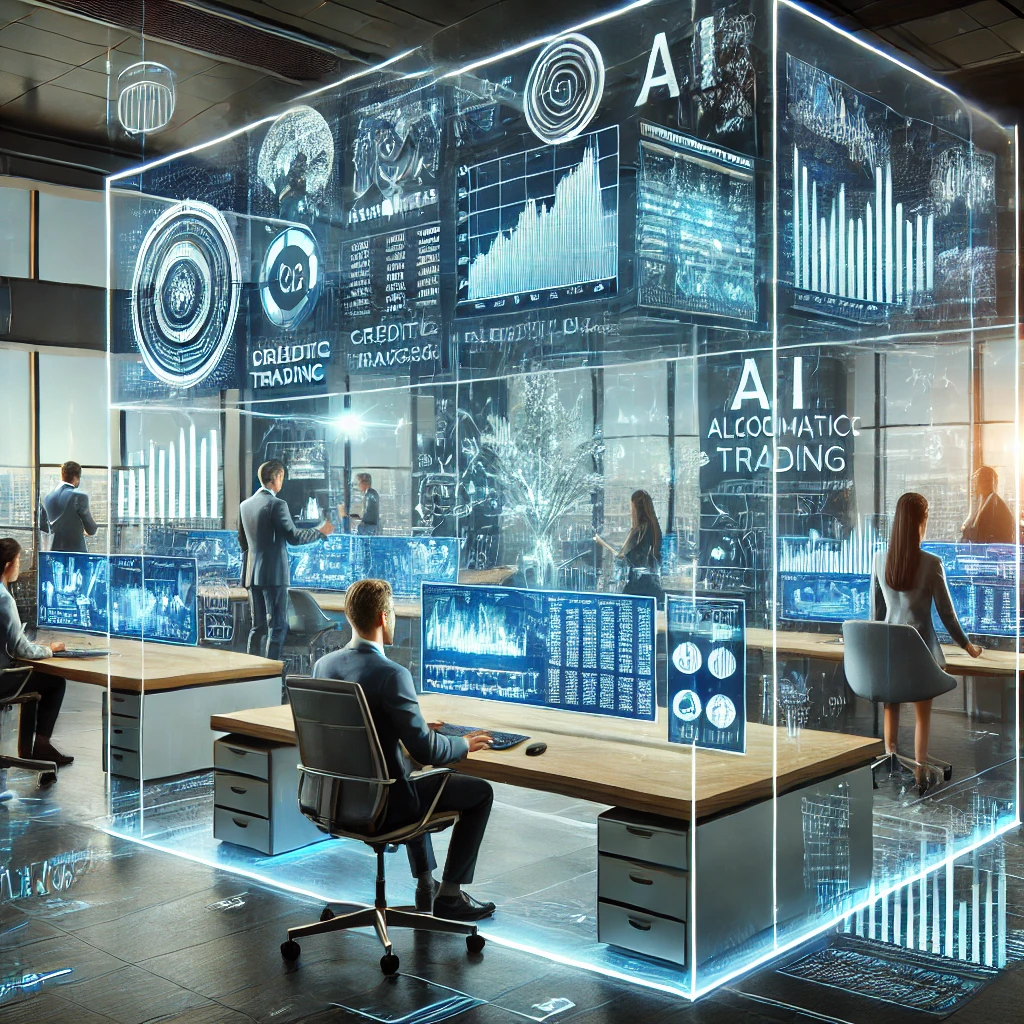
The rapid evolution of artificial intelligence (AI) is driving profound transformations in the financial sector, with deep learning (DL) at the forefront of this change. The research paper, "Deep Learning in Finance: A Survey of Applications and Techniques," authored by Ebikella Mienye, Nobert Jere, George Obaido, Ibomoiye Domor Mienye, and Kehinde Aruleba, and published in AI, delves into how DL is reshaping finance, its diverse applications, the challenges it presents, and the emerging trends poised to redefine the industry. The survey underscores the unparalleled capabilities of DL in processing large, complex datasets, enabling real-time decision-making, and uncovering intricate patterns, which traditional financial models often fail to detect.
A game-changer for the financial sector
Deep learning's applications in finance are extensive and transformative. Credit scoring, a cornerstone of financial risk assessment, benefits significantly from DL's ability to analyze complex borrower behaviors. By processing structured and unstructured data, DL models can offer highly accurate predictions and expand access to credit for underserved populations. In fraud detection, DL’s capacity to analyze transactional data in real time and identify anomalies has strengthened the security of financial systems. Models like Convolutional Neural Networks (CNNs) and Recurrent Neural Networks (RNNs) detect fraudulent patterns, protecting institutions and customers from potential threats. Furthermore, algorithmic trading has been revolutionized by DL, with Long Short-Term Memory (LSTM) networks and Transformers optimizing trading strategies by forecasting market trends and reacting to shifting conditions. These advancements are not only increasing profitability but also enhancing market stability by reducing latency in decision-making.
Another promising domain is portfolio management, where deep reinforcement learning is employed to balance risk and reward dynamically. Unlike static models, these algorithms adapt to market changes, offering superior performance and personalized investment strategies. Additionally, Generative Adversarial Networks (GANs) are playing a transformative role in synthetic data generation, which is vital in financial modeling and stress-testing. This approach helps simulate various economic scenarios, enabling institutions to prepare for potential market disruptions. These innovations demonstrate that DL is not merely an enhancement but a necessity for modern financial systems aiming to remain competitive in an increasingly complex environment.
Deploying deep learning in finance comes with a host of challenges. One critical issue is the quality and availability of financial data. Datasets in finance are often noisy, incomplete, or affected by non-stationarity, which complicates model training. Privacy concerns further restrict access to sensitive data, posing barriers to innovation. The "black-box" nature of DL models is another significant hurdle, as regulatory frameworks in finance demand transparency and explainability. Financial institutions often struggle to balance the trade-off between model accuracy and interpretability, which is essential for gaining stakeholder trust and complying with legal requirements. Moreover, the computational intensity of training DL models creates an additional challenge, particularly for smaller organizations lacking access to advanced infrastructure. Ethical concerns also loom large, with biases in training data potentially leading to discriminatory practices in areas such as loan approvals and credit scoring, underscoring the need for fairness and accountability in AI systems.
Emerging trends
Despite these challenges, the future of deep learning in finance is promising, with emerging trends poised to address many of the existing limitations. Researchers and developers are increasingly focusing on explainable AI (XAI), employing techniques such as SHAP (SHapley Additive exPlanations) and LIME (Local Interpretable Model-Agnostic Explanations) to make DL models more transparent and understandable. Federated learning is another groundbreaking development, enabling institutions to collaborate on model training without compromising data privacy, a critical concern in finance.
Transfer learning, which allows pre-trained models to be fine-tuned for specific tasks, is reducing the dependency on large datasets, making DL more accessible to smaller firms. The paper also explores the potential of quantum computing to revolutionize financial modeling, particularly in solving optimization problems and complex simulations that are beyond the reach of classical computing systems. Ethical AI is gaining traction as regulatory bodies and financial institutions recognize the need for fairness and inclusivity in AI-driven decision-making, addressing biases and ensuring accountability.
Collaboration and innovation
The paper highlights that long-term investments in research and development are essential to fully harness the potential of deep learning in finance. Collaboration among financial institutions, technology developers, and regulatory bodies will play a pivotal role in overcoming the barriers to adoption. Governments and policymakers must create an enabling environment that supports innovation while safeguarding ethical standards and data security. For financial institutions, adopting a proactive approach to integrating DL technologies can lead to enhanced operational efficiency, improved customer experiences, and a competitive edge in the market.
Overall, the study presents a compelling case for the transformative power of deep learning in finance. It not only highlights the current applications but also addresses the critical challenges and charts a path forward for sustainable and responsible adoption. As the financial industry becomes increasingly data-driven, the integration of DL technologies will be essential to navigate complexities, mitigate risks, and unlock new opportunities. With a focus on collaboration, transparency, and innovation, deep learning holds the potential to revolutionize the financial landscape, ensuring resilience and inclusivity in an ever-changing global economy.
- READ MORE ON:
- Deep learning in finance
- AI in financial services
- AI-driven financial models
- Deep learning applications in finance
- Fraud detection with deep learning
- Challenges in deploying AI in financial services
- Ethical concerns in deep learning for finance
- AI for predictive financial analysis
- fintech
- FIRST PUBLISHED IN:
- Devdiscourse