AI that forgets: New approach could revolutionize privacy and efficiency of large models
This new approach could be especially valuable in sensitive sectors like healthcare and finance, where privacy concerns are paramount. Allowing AI models to "forget" specific pieces of information without retraining the entire system could help protect user privacy while reducing the environmental impact of AI development.
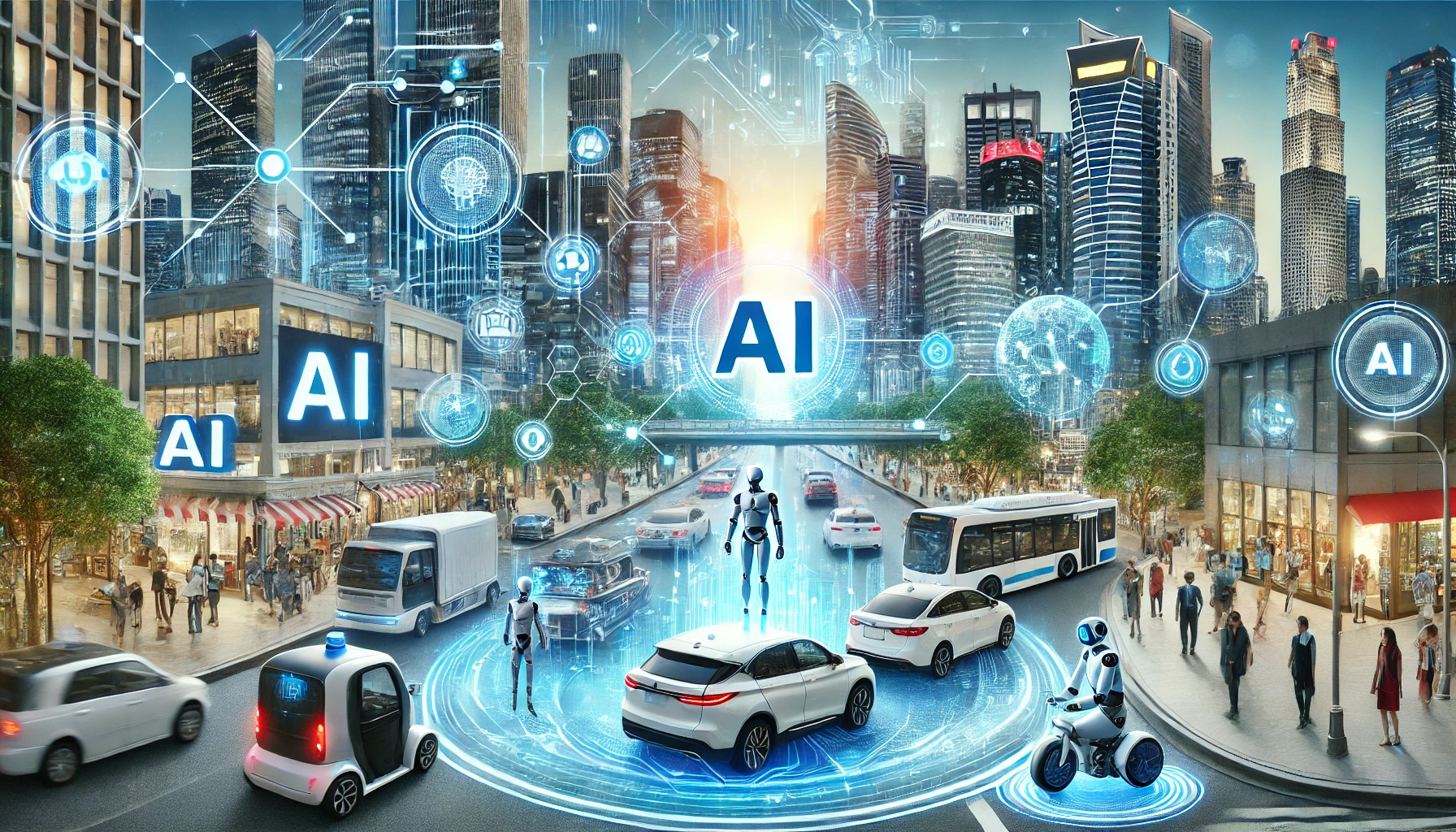
AI is no longer just a buzzword- it is transforming the way we interact with technology, enabling breakthroughs that were once thought impossible. Among the most recent and impressive innovations are large-scale pre-trained AI models, like CLIP and ChatGPT, which are capable of performing a wide range of tasks like generating text, images and videos with remarkable accuracy.
However, the immense energy consumption, lengthy training times, and limited scalability for practical applications make large-scale AI models difficult to deploy efficiently. So, what if there was a way to make them more efficient by allowing them to ‘forget’ unnecessary information? Could selectively erasing irrelevant data enhance performance while reducing resource consumption?
A recent study by the Tokyo University of Science (TUS) offers a potential solution. Led by Associate Professor Go Irie, the research team has developed a novel methodology called "black-box forgetting" that could significantly improve the efficiency of large-scale AI models. Their groundbreaking work addresses a major challenge in AI - how to optimize these models to perform specialized tasks without the burden of unnecessary information.
Limitations of generalist AI models
Large-scale AI models, such as CLIP, have proven their value with their abilities to handle a wide range of tasks. However, in many real-world applications, this versatility comes at a cost. For example, in autonomous driving, the system only needs to recognize a limited set of objects like cars, pedestrians, and traffic signs. The model’s ability to recognize a broad range of other objects, such as food or furniture, is not only unnecessary but could also reduce the model's accuracy and waste valuable computational resources.
"Retaining the classes that do not need to be recognized may decrease overall classification accuracy, as well as cause operational disadvantages such as the waste of computational resources and the risk of information leakage," explains Dr. Go Irie.
The challenge, then, is how to make these large-scale models more efficient by allowing them to "forget" irrelevant information, without losing their ability to perform critical tasks.
Black-Box Forgetting
In their study, Dr. Irie and his team proposed an innovative solution called "black-box forgetting." Unlike traditional methods that require access to a model’s internal architecture or parameters (called a white-box setting), the new approach allows researchers to optimize models without needing to examine or alter their internal workings. This is especially important because many AI models, particularly those used in commercial applications, are considered “black boxes”- meaning users do not have access to the underlying details of the model’s design due to commercial or ethical reasons.
The researchers applied this methodology to CLIP and developed a process to iteratively optimize the input prompts presented to the model. By doing so, they could selectively "forget" certain classes of objects that were unnecessary for the task at hand.
Overcoming Challenges
One of the major challenges in applying this approach to large-scale AI models is the sheer size of the optimization problem. As the number of classes to be forgotten increases, the complexity of the task grows exponentially. To address this, the team developed a novel technique called "latent context sharing." This method decomposes the latent context derived from input prompts into smaller, more manageable units. By optimizing these smaller elements instead of large chunks of data, the researchers were able to reduce the dimensionality of the problem, making it far more tractable.
The results of the study are promising. Using benchmark image classification datasets, the team was able to get CLIP to forget up to 40% of the classes in a given dataset. This marked the first time a pre-trained vision-language model had been made to forget specific classes under black-box conditions. The success of this novel technique demonstrates the potential for large-scale AI models to be more efficient, specialized, and adaptable to real-world applications.
Implications for AI efficiency and privacy
The potential applications of black-box forgetting extend far beyond improving the efficiency of generalist models. As AI becomes more integrated into our daily lives, concerns about data privacy are growing. In many cases, individuals or organizations may want certain information to be removed from an AI model, but retraining the model from scratch to remove specific data is an energy-intensive and costly process.
Black-box forgetting could provide a more efficient solution to this problem. As Dr. Irie explains, "If a service provider is asked to remove certain information from a model, this can be accomplished by retraining the model from scratch by removing problematic samples from the training data. However, retraining a large-scale model consumes enormous amounts of energy. Selective forgetting, or so-called machine unlearning, may provide an efficient solution to this problem."
This new approach could be especially valuable in sensitive sectors like healthcare and finance, where privacy concerns are paramount. Allowing AI models to "forget" specific pieces of information without retraining the entire system could help protect user privacy while reducing the environmental impact of AI development.
A step toward smarter, more sustainable AI models
The groundbreaking research by Dr. Irie and his team at Tokyo University of Science marks a pivotal moment in the evolution of AI. Their innovative approach to enabling large-scale models to 'forget' irrelevant information is set to make AI more efficient, specialized, and sustainable.
Optimizing large-scale AI models without compromising their performance or accuracy will be crucial for their widespread adoption. With innovative approaches like black-box forgetting, we are one step closer to realizing the full potential of AI - making it not only smarter but also more aligned with sustainability goals and real-world needs.
In the coming years, we can expect AI to become more integrated into our daily lives, with smarter, more specialized models that can handle tasks with precision and efficiency.
- FIRST PUBLISHED IN:
- Devdiscourse
ALSO READ
Texas Attorney General Ken Paxton Targets Tech Platforms Over Minors' Privacy
Apple Clashes with Meta Over Privacy Concerns
Tech Titans Clash: Apple and Meta Spar Over Privacy and Access
Netflix Faces €4.75M Fine for Privacy Lapses
Housekeeper Arrested for Invasion of Privacy: Hidden Camera in Hospital Washroom