Revolutionizing Financial Risk Management with Blockchain and Deep Learning
Researchers from Tongling University developed a model that combines blockchain and deep learning to enhance the security and accuracy of financial data sharing and risk identification in enterprises. The model outperforms existing methods, providing significant improvements in efficiency, security, and decision-making capabilities.
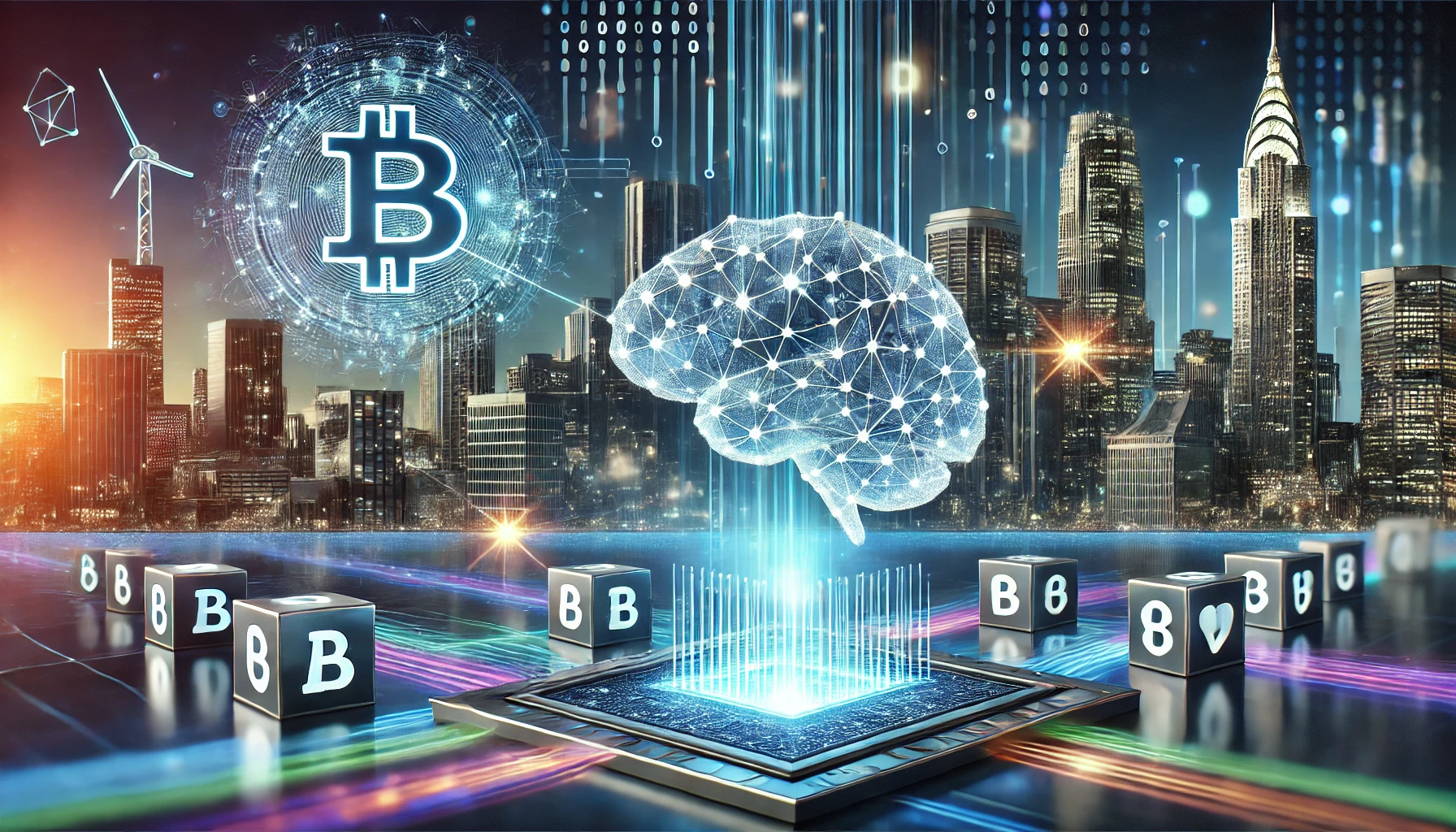
A recent study conducted by researchers at Tongling University in China introduces an innovative model that merges blockchain technology with advanced deep learning algorithms to address the complex challenges of financial data sharing and risk identification in enterprises. This research is particularly timely given the increasing reliance on digital financial transactions and the corresponding rise in security threats.
Addressing Traditional Financial Vulnerabilities
Traditional methods of financial information sharing have long been fraught with vulnerabilities, including risks of data leakage, unauthorized access, tampering, and a lack of transparency. These weaknesses have created significant trust issues among enterprises, especially in environments where large volumes of financial data are exchanged. In response to these challenges, the study proposes a decentralized blockchain framework designed to transform the conventional centralized financial networks into more secure and transparent systems.
Leveraging Blockchain for Secure Financial Transactions
The blockchain technology used in this study is characterized by its decentralization, immutability, and transparency, making it a robust solution for mitigating trust issues and enhancing the security of financial transactions. The researchers have leveraged these characteristics to create a model that not only secures financial data but also improves the overall efficiency of financial information sharing. By decentralizing the data storage and management process, blockchain ensures that financial information remains secure and tamper-proof, thereby fostering greater trust among participating entities.
Innovative Hybrid Model for Risk Identification
A key aspect of the study is the development of a sophisticated financial risk identification model that integrates Bi-directional Long Short-Term Memory (BiLSTM) networks with transformer models. This hybrid approach allows the model to process both textual and visual data, providing a more comprehensive understanding of the financial landscape. The BiLSTM component is particularly effective in capturing the temporal dependencies within financial data, which is crucial for accurate risk assessment. Meanwhile, the transformer model enhances the ability of the system to manage and analyze complex financial sequences, making it possible to identify potential risks with a high degree of precision.
Enhanced Performance and Security
The model classifies financial risks into five distinct levels, ranging from excellent to very high risk. This stratification enables enterprises to better understand their financial positions and take proactive measures to mitigate potential risks. The study's experimental analysis reveals that the proposed model outperforms existing algorithms, such as the Byzantine Fault Tolerance (BFT) algorithm and standard Recurrent Neural Networks (RNNs), in several key areas. For instance, the model achieves a significantly higher throughput, which is the measure of how much data can be processed in a given amount of time. This is particularly important for enterprises that need to handle large volumes of financial data efficiently.
Moreover, the study finds that the model maintains a low data leakage rate and packet loss rate, even as the volume of data increases. This indicates that the model is not only more secure but also more reliable than existing solutions. The researchers report that the accuracy of the model in identifying financial risks exceeds 94%, with an Area Under the Curve (AUC) value of over 0.95. The AUC is a critical metric in evaluating the performance of a risk identification model, as it measures the ability of the model to distinguish between different levels of risk. Additionally, the time required for risk identification is reduced by approximately 10 seconds compared to traditional methods, which is a significant improvement in the context of financial decision-making processes that often require quick and accurate assessments.
Future Research and Practical Implications
The implications of this research are profound for the field of enterprise financial management. By combining the strengths of blockchain technology with advanced deep learning models, the study offers a powerful tool for enhancing the security and efficiency of financial data sharing. This is particularly relevant in today’s rapidly evolving digital economy, where the ability to manage financial risks effectively can make the difference between success and failure for many enterprises. However, the study also acknowledges certain limitations, such as the need for further validation of the model's generalizability across different industries and its performance in real-world scenarios. The researchers suggest that future studies should focus on improving the interpretability of the model and refining its parameters to better align with the specific needs of different types of enterprises.
The study represents a significant advancement in the field of financial data management and risk identification. The blockchain-supported, deep learning-based approach not only addresses many of the security and efficiency issues that have plagued traditional financial systems but also provides a framework for future research and development in this area. The findings suggest that enterprises adopting this model could benefit from enhanced financial decision-making capabilities, greater operational security, and improved trust among stakeholders. As the digital economy continues to grow, the adoption of such innovative solutions will likely become increasingly important for maintaining competitiveness and achieving sustainable growth.
- READ MORE ON:
- blockchain
- blockchain technology
- BiLSTM
- Byzantine Fault Tolerance
- FIRST PUBLISHED IN:
- Devdiscourse