Detecting Digital Addiction: EarlySD Framework Targets Short-form Video Overuse
Fang-Yu Kuo's research at National Tsing Hua University develops the EarlySD framework to detect Short-form Video Addiction (SFVA) using social media behavior and large language models, aiming to mitigate its negative impacts. This innovative approach highlights the importance of early intervention to promote healthier digital habits.
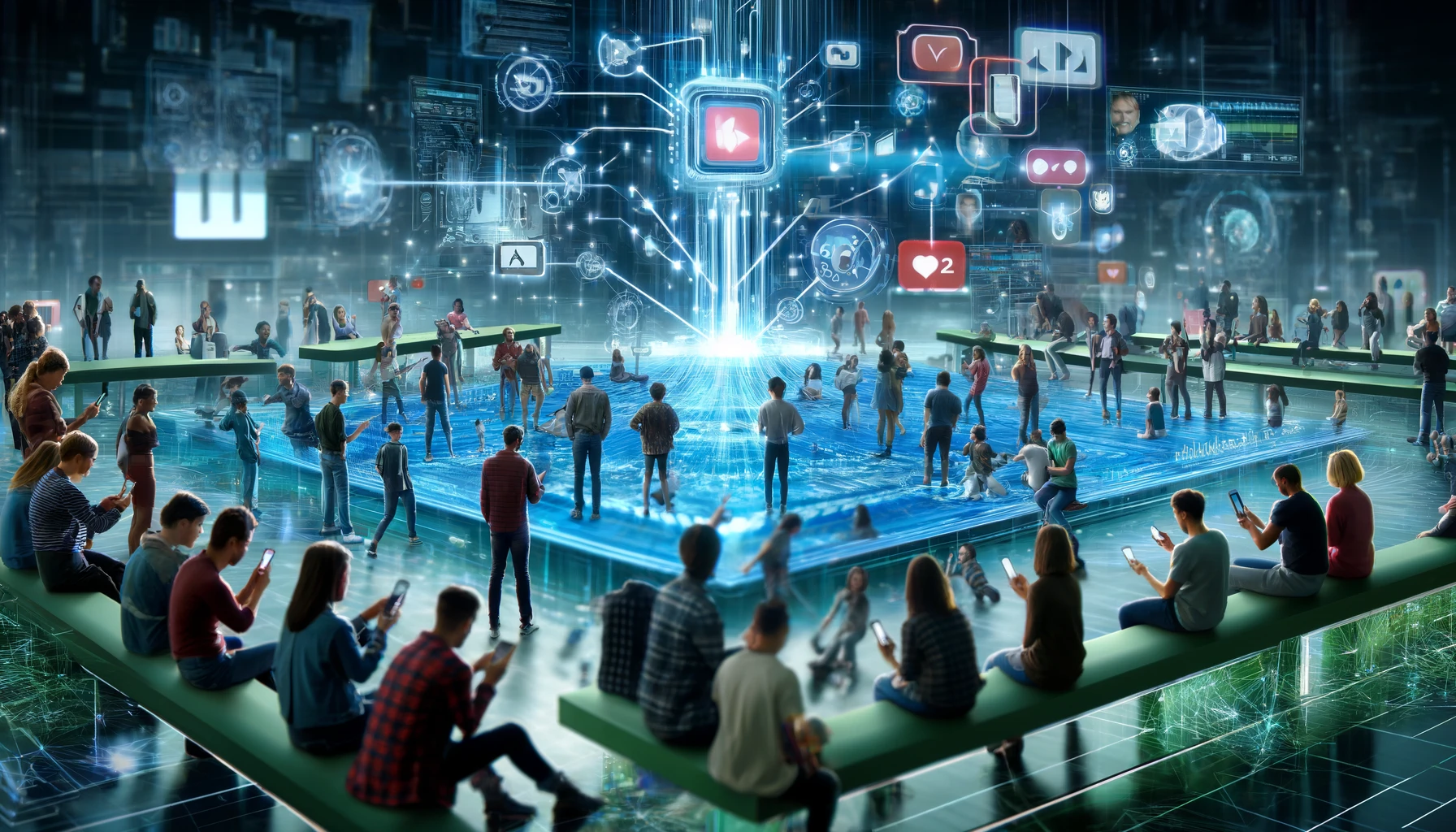
Short-form videos (SFV) have rapidly become a global phenomenon, featured prominently on major social media platforms like Instagram Reels, Facebook Reels, and YouTube Shorts. This surge in popularity, however, has raised significant concerns about Short-form Video Addiction (SFVA), a condition that poses substantial risks to users' physical and psychological well-being. SFVA can lead to decreased attention spans, reduced motivation to learn, and various psychological issues such as social anxiety and a lack of real-life support. Fang-Yu Kuo's research at the Department of Computer Science, National Tsing Hua University in Taiwan, aims to address SFVA by developing an early detection framework using social network data. This study introduces the Early-state Short-Form Video Addiction Detector (EarlySD), which leverages large language models (LLMs) and a heterogeneous social network structure to identify potential addicts based on their online behavior.
Early Detection of Digital Addiction
Current methods for detecting SFVA typically identify the condition only after negative consequences have become apparent. However, EarlySD seeks to intervene earlier by analyzing social media interactions such as searching, commenting, and forming bidirectional friendships. This approach categorizes social network behavior data into different modalities, constructing a comprehensive dataset for detecting SFVA. The research involved recruiting 534 Taiwanese participants who completed a detailed questionnaire, including the Short Video Addiction Scale and the Social Interaction Anxiety Scale. The findings revealed significant correlations between SFVA and factors such as age, social anxiety, and neuroticism. SFVA users tended to be younger and exhibited higher levels of social anxiety and neuroticism compared to non-addicts.
Social Media Behaviors Unveiled
The study's findings indicate that social behavior plays a crucial role in distinguishing SFVA users. Addicts were more active in searching for other accounts, especially their friends, and engaged more in interactions like commenting and stories on social platforms. They also had a higher tendency to form bidirectional friendships, indicating closer social connections. This increased activity and connectivity among SFVA users suggest that their addiction might be driven by a deeper need for social interaction and validation, which they seek to fulfill through online platforms.
A Groundbreaking Dataset
One of the key contributions of this research is the creation of the first real SFVA dataset with online social behavior traces. This dataset bridges the gap between online social behavior data and the risk of SFVA, aiming to raise public and academic awareness of this addiction. By conducting quantitative analyses of SFVA users, the researchers were able to create detailed profiles of individuals with varying degrees of addiction tendencies, including SFVA, Potential-SFVA, and Non-SFVA. This was achieved by collecting personal social media data from Instagram, uncovering the correlation between addictive behaviors and social media usage patterns.
Innovative Framework for SFVA Detection
The EarlySD framework addresses several challenges in detecting SFVA, such as data sparsity and feature incompleteness, by utilizing LLMs to optimize the dataset. The framework includes a heterogeneous graph neural network classifier that considers user characteristics and interests to accurately detect SFVA tendencies. Extensive experiments using real-world Instagram data validated the effectiveness of EarlySD, demonstrating its superiority over existing methods in terms of accuracy, precision, recall, and F1-score.
Insights from Social Media Content
The study's preliminary analyses highlighted the potential efficacy of both personal and social features in capturing short video addiction tendencies. By using ANOVA tests, the researchers identified significant differences between SFVA and Non-SFVA groups in certain social behavior indicators. For instance, SFVA users showed a more pronounced interest in others, especially their friends, and were more active in various types of interactions on social platforms. Additionally, they tended to establish bidirectional friendships more frequently than Non-SFVA users, reflecting a greater need for mutual social connections.
Insights from user-generated content on social media also revealed interesting patterns. The types of topics users were interested in and the content recommended by the platform played a significant role in influencing their addiction tendencies. By analyzing the data provided by Instagram, the researchers found that SFVA users exhibited distinct preferences for certain topics, which further contributed to their addiction.
To enhance the social graph used for detection, the researchers employed a two-step approach: expanding the potential topic set based on user-generated content with the help of LLMs and using a link prediction model to address the issue of incomplete user-topic edges. This augmentation process generated potential topics and constructed new user-topic edges, enriching the user-topic relationship in the social graph.
Promoting Healthier Digital Habits
The study's comprehensive approach to detecting SFVA underscores the importance of early intervention and the potential of leveraging social media data for mental health detection. By identifying addicts before severe consequences arise, EarlySD can help mitigate the negative impacts of short-form video addiction, promoting healthier social media usage and psychological well-being. This research not only provides a novel method for early detection of SFVA but also highlights the need for ongoing awareness and preventive measures in the rapidly evolving landscape of social media.
- FIRST PUBLISHED IN:
- Devdiscourse