SEE Framework: A Leap Towards Sustainable IoT with 60 percent Energy Savings and High Accuracy
Researchers at Washington State University developed the Sensor-Aware Early Exit (SEE) framework, which significantly reduces energy consumption in IoT devices by making high-confidence predictions with partial data, achieving up to 60% energy savings without sacrificing accuracy. This approach enhances the sustainability and efficiency of time-series applications across diverse fields such as health monitoring and environmental sensing.
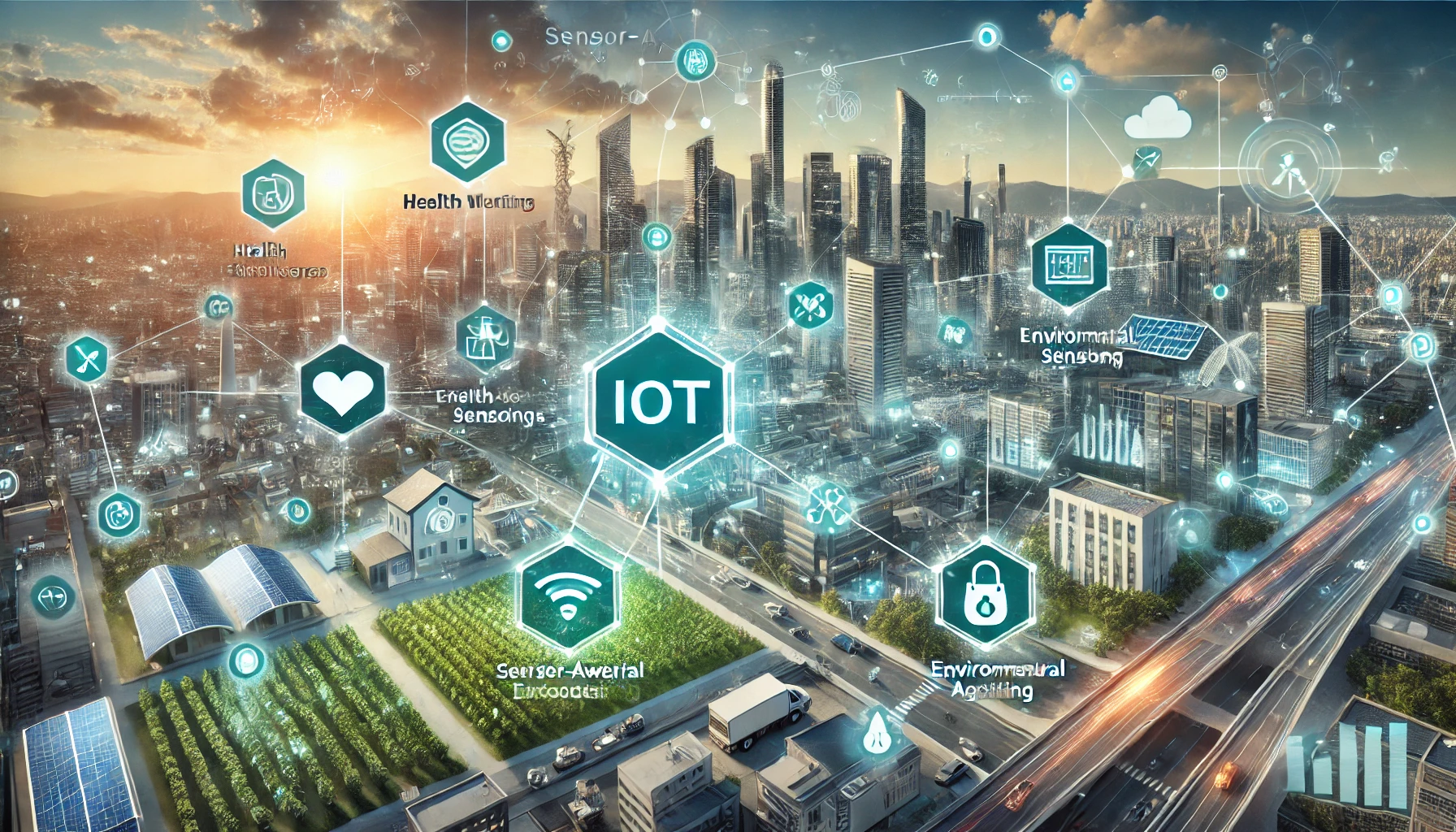
Researchers from Washington State University have introduced a groundbreaking approach to significantly reduce the energy consumption of time-series applications on Internet of Things (IoT) devices. This new method, known as Sensor-Aware Early Exit (SEE), aims to optimize the use of machine learning (ML) models by making accurate predictions with partial data segments, thereby conserving sensor energy. Time-series data processing is pivotal in numerous real-world applications such as health monitoring, environmental sensing, and digital agriculture. Traditionally, these applications accumulate complete sensor data windows before processing, leading to high energy consumption. The research team, consisting of Dina Hussein, Lubah Nelson, and Ganapati Bhat, proposes an innovative solution where early exit classifiers use partial sensor data to make high-confidence inferences. This approach allows sensors to enter low power mode sooner, achieving up to 50-60% energy savings without compromising accuracy.
Sensor-Aware Early Exit: A Game-Changer for IoT Efficiency
The SEE framework leverages neural networks and random forest classifiers to validate its effectiveness. By implementing early exit layers within these ML models, the researchers demonstrated that significant energy savings are possible. Their evaluations on six datasets, covering diverse applications such as activity recognition and health monitoring, confirm that the SEE approach can maintain or even improve classification accuracy while drastically reducing energy usage. This research addresses a critical challenge for IoT devices, which often operate under strict energy constraints. By optimizing sensor energy consumption, the SEE framework holds promise for extending the operational life of battery-powered devices and enabling the deployment of time-series applications in remote areas with limited energy resources. The study underscores the potential of early exit classifiers to enhance the efficiency and sustainability of IoT systems.
Redefining Time-Series Data Processing
In typical time-series applications, sensors collect data over a window of time before processing it to generate results. This method, while effective, is energy-intensive because it requires sensors to be active for the entire duration of data collection. The SEE approach changes this paradigm by allowing ML models to exit early when a high-confidence prediction can be made with partial data. For example, in health monitoring applications, some activities like sitting or standing can be accurately identified with less data compared to more complex activities like walking or running. The SEE framework introduces multiple exit points within the ML models, which assess prediction confidence at various stages. If the confidence threshold is met at an early exit, the prediction is made, and sensors can be turned off, thus saving energy.
Proven Success Across Diverse Datasets
The researchers evaluated the SEE approach using six diverse datasets. These included WESAD for wearable stress and affect detection, the Shoaib dataset for physical activity recognition, PAMAP2 for activity monitoring, EMG for physical action detection, Epilepsy for seizure recognition, and SelfRegulationSCP1 for EEG data analysis. The results were impressive, showing that the SEE models could achieve energy savings of up to 60% without sacrificing accuracy. In some cases, the accuracy of the SEE models even surpassed that of traditional models because the SEE framework could better train later layers of the neural network to handle more difficult classification tasks.
Versatility and Feasibility of SEE Framework
One of the significant advantages of the SEE approach is its generalizability. It was effective across various ML models, including convolutional neural networks (CNNs) and random forest classifiers. This versatility makes it a robust solution for a wide range of time-series applications. The SEE CNN models, for instance, integrated early exit and late input blocks to dynamically incorporate new sensor data as it became available, ensuring comprehensive analysis without unnecessary energy expenditure. Random forest classifiers were adapted to form an ensemble, with each classifier processing increasing amounts of sensor data, allowing for early exits based on prediction confidence.
A Step Towards Sustainable IoT Devices
The study also addressed the practical aspects of deploying SEE classifiers on resource-constrained devices. The memory overhead of the SEE models was found to be within acceptable limits for devices like the Nvidia AGX Xavier, commonly used for edge applications. This makes the SEE framework not only energy-efficient but also feasible for real-world implementation. Additionally, the computational energy required for the extra layers and blocks in SEE models was negligible compared to the energy savings achieved through reduced sensor operation.
The SEE framework represents a significant advancement in the field of IoT by addressing the dual challenges of maintaining high accuracy and reducing energy consumption in time-series applications. This innovative approach opens up new possibilities for the deployment of IoT devices in energy-constrained environments, such as remote health monitoring and environmental sensing. By ensuring that sensors operate only as long as necessary to achieve accurate predictions, the SEE framework can greatly extend the battery life of IoT devices, making them more sustainable and reliable for long-term use. This research marks a crucial step forward in enhancing the efficiency and viability of IoT systems in various applications.
- FIRST PUBLISHED IN:
- Devdiscourse