Machine Learning Revolutionizes Crustal Deformation Forecasting Over Tibetan Plateau
The research team employed a range of machine learning techniques, including support vector machines, decision trees, and Gaussian process regression, to accurately model crustal movements.
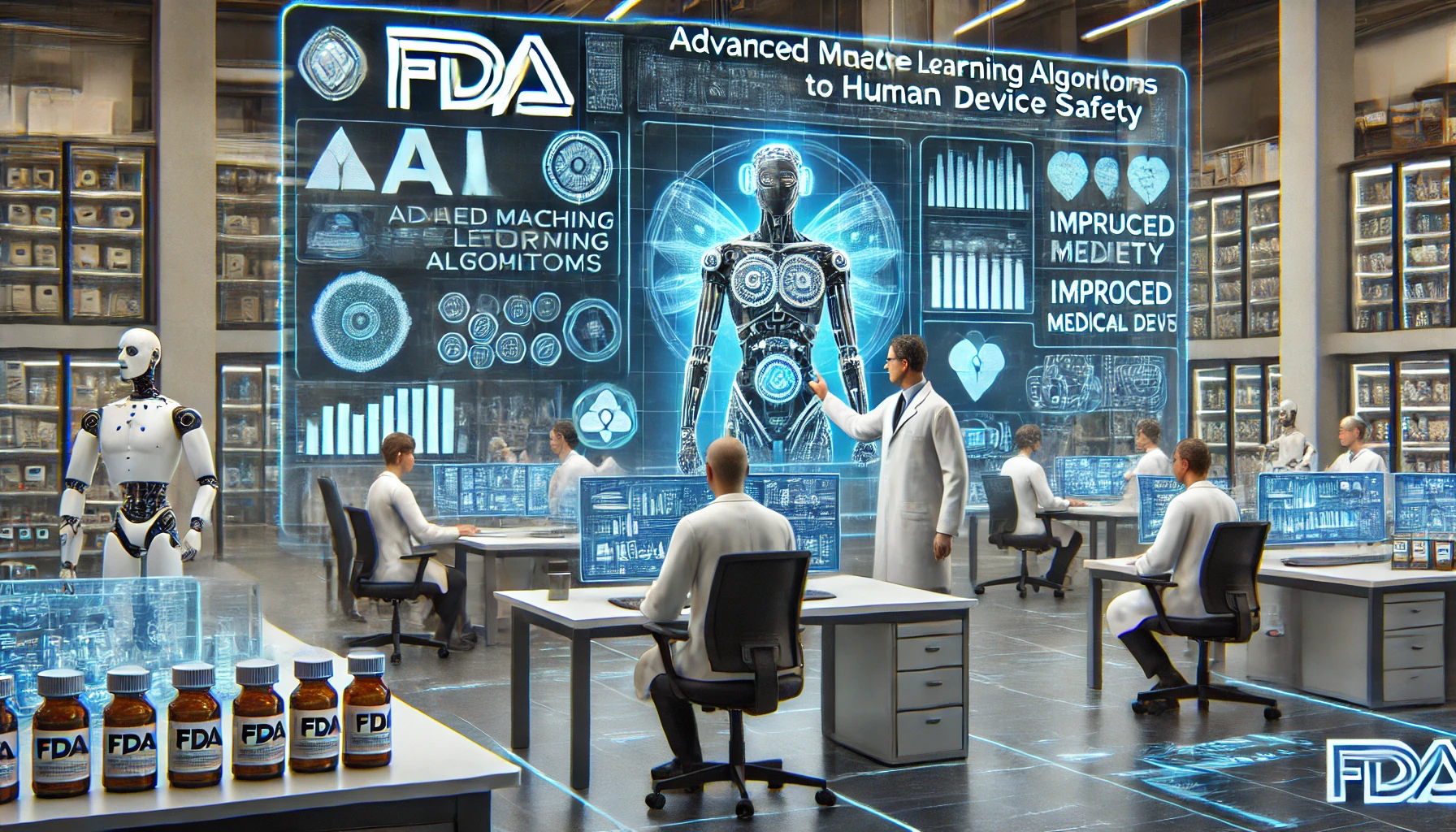
- Country:
- India
Scientists at the Wadia Institute of Himalayan Geology, an autonomous institute under the Department of Science and Technology (DST), Government of India, have successfully implemented machine learning techniques to model crustal deformations over the Tibetan Plateau, leading to significant advancements in the forecasting of velocity vectors and the characterization of plate movements.
Traditionally, the monitoring of crustal deformations relies on a dense network of Continuously Operating Reference Stations (CORS) and campaign-mode GPS surveys to provide accurate data. However, establishing these stations in the desired locations can be logistically challenging, geographically constrained, and expensive. Data gaps due to these limitations often hinder the study of crustal movements. This is where machine learning (ML) comes to the rescue, offering a cost-effective solution for predicting GPS site velocity, critical for crustal deformation research.
The research team employed a range of machine learning techniques, including support vector machines, decision trees, and Gaussian process regression, to accurately model crustal movements. They analyzed data from 1,271 GPS stations—both permanent continuous and campaign-mode—spread across the Tibetan Plateau and its surrounding areas. The dataset was divided into two parts: 892 stations were used for training the ML models, and 379 stations were used for testing.
Published in the Journal of Asian Earth Sciences, the study showcases the effectiveness of these machine learning models in forecasting velocity vectors, specifically the easting velocity (VE) and northing velocity (VN). The correlation between the predicted and actual velocity vectors was found to be highly satisfactory, demonstrating the reliability of these ML models for estimating geodetic velocity vectors.
By utilizing data-driven trends from the trained models, the scientists were able to input the locations of arbitrary GPS sites and predict the VE and VN at those points. The predicted velocities closely mirrored those obtained from nearby GPS stations, highlighting the accuracy and consistency of the machine learning algorithms.
This breakthrough underscores the potential of machine learning in geodetic studies, offering a cost-effective and efficient tool for predicting crustal deformations in regions where traditional monitoring methods are difficult to implement. The success of this study marks a significant achievement in the field of geodesy, providing researchers with a powerful new method to enhance the understanding of plate movements and crustal dynamics.