AI-Driven Lag-Llama Model Outperforms Traditional MIDAS in Euro Area Inflation Forecasting
Researchers from the University of Groningen compared the traditional MIDAS regression model and the AI-driven Lag-Llama model for inflation nowcasting in the Euro area, finding Lag-Llama generally outperformed MIDAS across multiple metrics. This study highlights the potential of advanced machine learning techniques in improving economic forecasting accuracy.
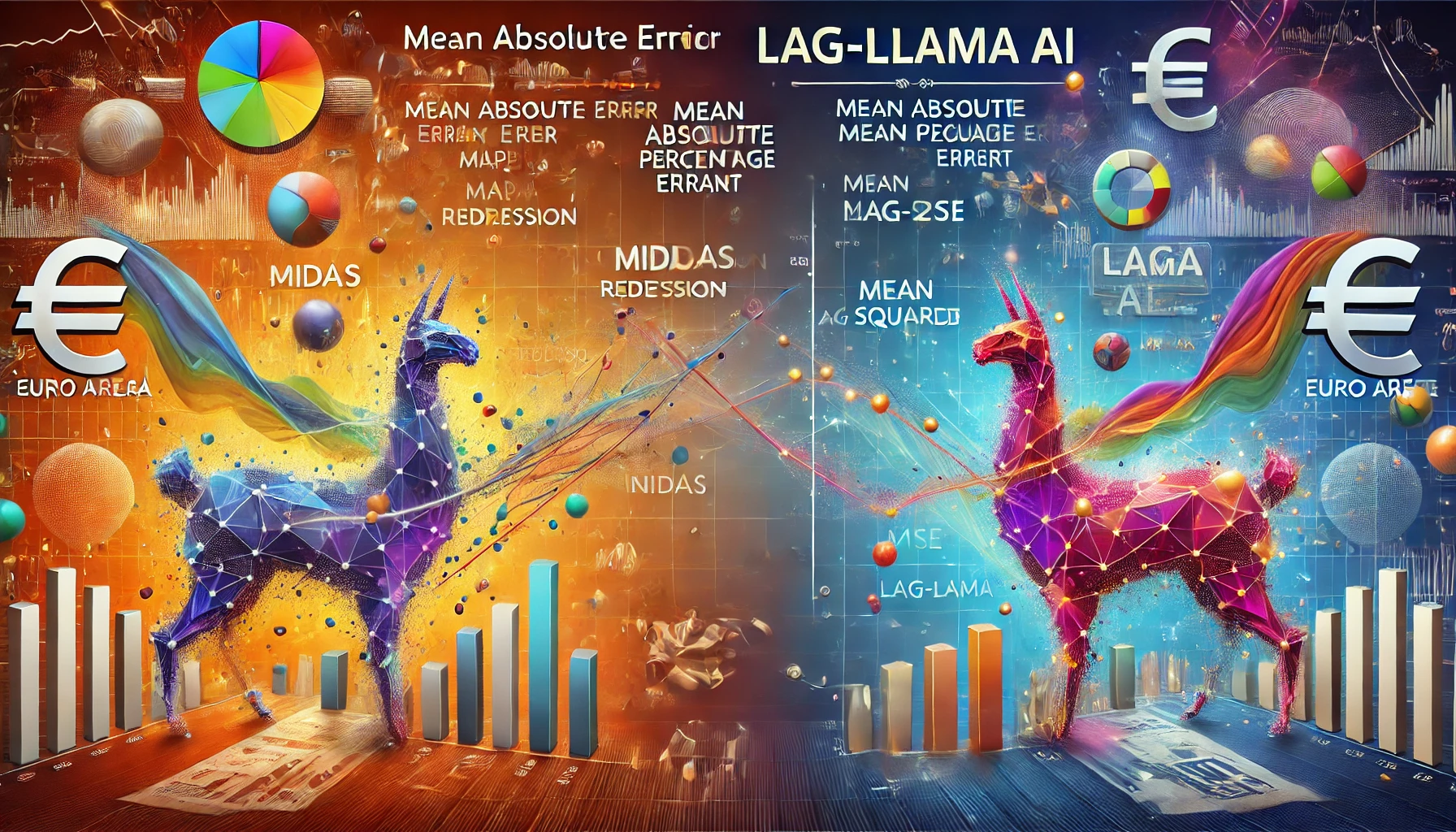
In a recent comparative analysis conducted by researchers from the University of Groningen, Adam Bahelka and Harmen de Weerd investigated the efficacy of two models in nowcasting the Harmonized Index of Consumer Prices (HICP) in the Euro area: the Mixed-Data Sampling (MIDAS) regression and an advanced machine learning model known as Lag-Llama, which is based on Long Short-Term Memory (LSTM) neural networks. Utilizing a comprehensive dataset that spanned from 2010 to 2022, their primary objective was to determine if the AI-driven Lag-Llama could surpass the traditional MIDAS regression in predictive accuracy.
Inflation Nowcasting: The Role of Mixed-Frequency Data
Inflation nowcasting plays a crucial role for both policymakers and market participants, as it involves predicting the current rate of inflation using the most recent data available. One of the significant challenges in this process is dealing with mixed-frequency data, where inflation is measured monthly, but other relevant indicators, such as financial market data, are available on a daily basis. Traditional econometric models like ARIMA and VAR are not designed to handle such mixed-frequency data effectively, often requiring the aggregation of high-frequency data to match the lower frequency of the target variable. This aggregation can lead to the loss of valuable information, thereby reducing forecast accuracy.
The Advantages of MIDAS Regression
The MIDAS regression model, developed by Ghysels and colleagues, addresses this issue by allowing the use of high-frequency data without aggregation, thus preserving the valuable information inherent in daily data. This model has been widely adopted by both public institutions and private entities to improve the accuracy and timeliness of economic forecasts. On the other hand, the Lag-Llama model represents a recent advancement in the field of machine learning. This LSTM-based model is trained on a vast array of time-series datasets and excels at zero-shot forecasting, meaning it can make accurate predictions on datasets it has not been explicitly trained on. Despite its robust capabilities, the Lag-Llama model does not support mixed-frequency data, which can limit its effectiveness in applications requiring the integration of such data.
Comprehensive Data for Accurate Forecasting
For their study, the researchers created a dataset spanning from June 2010 to June 2022, incorporating daily prices of Brent crude oil, interest rate spreads, short-term and long-term interest rates, exchange rates, and the Eurostoxx50 index. These variables were selected based on their relevance to market and inflation expectations. Various pre-processing techniques were applied to the data, such as calculating daily fractional changes and down-sampling the target variable to a daily frequency for use with the Lag-Llama model.
Lag-Llama Outperforms MIDAS in Key Metrics
The performance of both models was evaluated using several metrics, including Mean Absolute Error (MAE), Mean Absolute Percentage Error (MAPE), Mean Squared Error (MSE), correlation with the target, R-squared, and adjusted R-squared. The findings revealed that the Lag-Llama model demonstrated superior performance across all these metrics when compared to the MIDAS regression. Specifically, Lag-Llama achieved a MAE of 0.21 compared to MIDAS’s 0.23, a MAPE of 0.40% versus MIDAS’s 0.50%, and a MSE of 0.07 against MIDAS’s 0.11. Additionally, the Lag-Llama model exhibited a higher correlation coefficient of 0.92, indicating a stronger alignment with the actual inflation data compared to MIDAS’s 0.88.
Challenges and Future Directions in Economic Forecasting
Despite evaluating the MIDAS regression under optimal conditions, which included in-sample predictions and the use of mixed-frequency data, the Lag-Llama model still managed to outperform it. This suggests that the advanced machine learning techniques employed by Lag-Llama offer significant advantages in terms of predictive accuracy. However, the discussion section of the study also highlighted the challenges associated with using LSTM models in practical applications, particularly within public institutions. The "black box" nature of LSTM models, which obscures the reasoning behind their predictions, can pose significant issues in contexts where transparency and interpretability are essential for decision-making.
The study concludes that while the Lag-Llama model did not surpass the MIDAS regression by the strict margin initially defined, it still demonstrated overall better performance. This finding underscores the potential of integrating advanced machine learning techniques into economic forecasting to enhance the accuracy and timeliness of predictions. The performance of the Lag-Llama model highlights the promising role that foundational models and machine learning architectures can play in the field of econometrics, traditionally dominated by more conventional models. As economic and financial data continue to grow in volume and complexity, the relevance and utility of such advanced models are likely to increase, offering new opportunities for more precise and timely economic forecasting.
- FIRST PUBLISHED IN:
- Devdiscourse