FairJob Dataset: Enhancing Fairness and Reducing Bias in Online Job Recommendation Systems
The FairJob dataset, developed to enhance fairness in online job recommendation systems, includes anonymized data and a gender proxy to address biases without compromising privacy. It provides a comprehensive resource for studying and mitigating algorithmic biases, ensuring ethical AI practices in high-impact domains like job advertising.
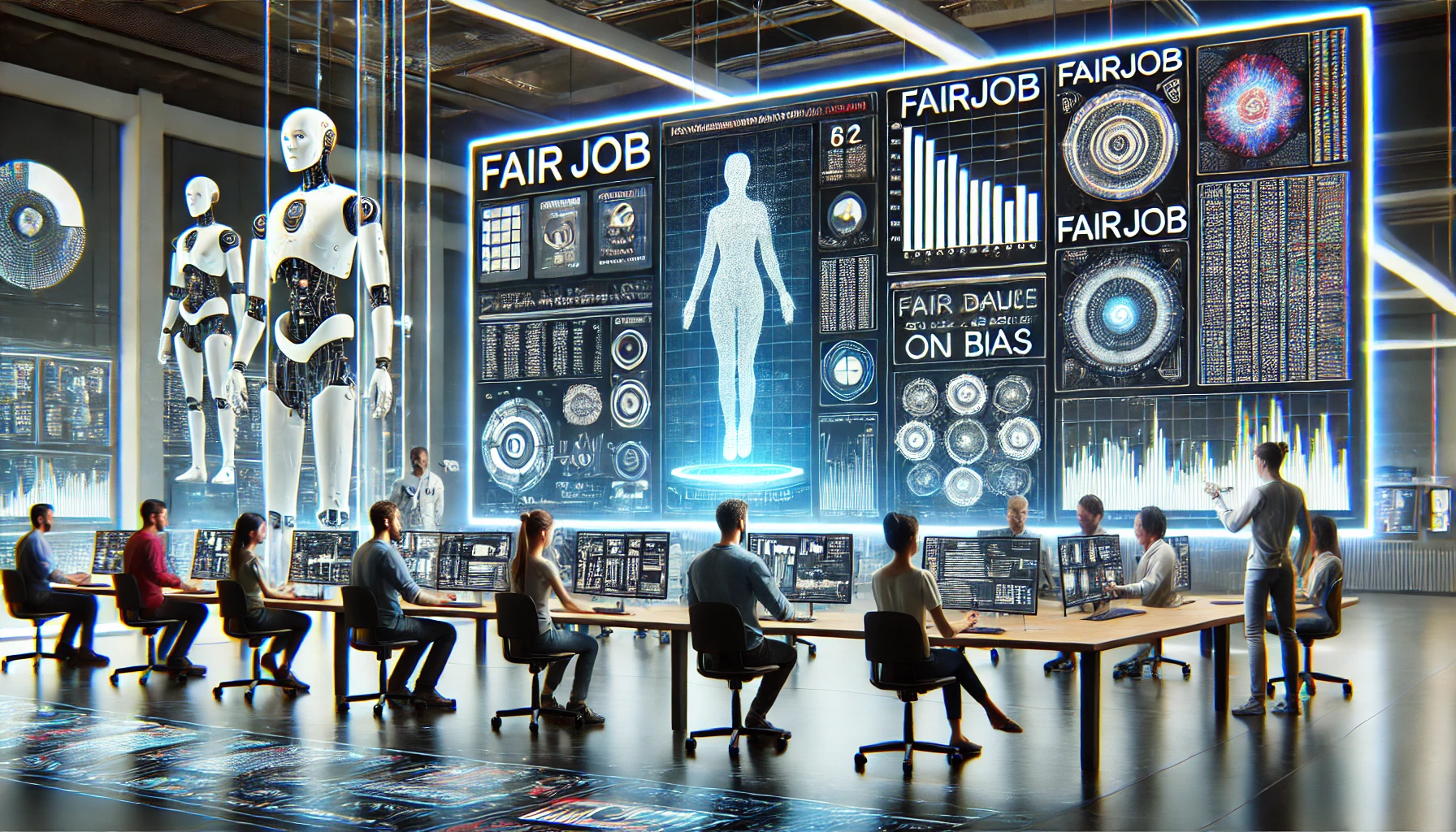
The FairJob dataset is a pioneering initiative aimed at addressing fairness in online job recommendation systems. Created by Mariia Vladimirova and Eustache Diemert from Criteo AI Lab, along with Federico Pavone from Universite Paris Dauphine-PSL, this dataset was meticulously designed to comply with privacy standards and business confidentiality while fostering research in algorithmic fairness. It offers a unique challenge by including a proxy for sensitive attributes like gender, ensuring that predictive power is maintained despite anonymization.
The Intersection of AI and Human Dynamics
In the realm of artificial intelligence, the intersection of technology and human dynamics brings both opportunities and challenges. Historical biases ingrained in data often influence AI model outcomes, perpetuating societal inequalities. This issue is particularly significant in high-impact domains such as job advertising, where algorithmic fairness is crucial. The dataset highlights the need for careful auditing and bias mitigation to ensure that AI systems do not amplify existing disparities.
A Comprehensive Dataset for Fairness Analysis
The dataset was collected over five months from a targeted job campaign and includes over a million rows, each representing a job ad and various user and product features. Notably, the dataset includes a gender proxy derived from user interactions with gendered products, which, while not directly indicating gender, serves as a relevant attribute for fairness analysis. This proxy and other anonymization techniques ensure that the dataset remains a realistic benchmark without compromising user privacy. FairJob addresses several key stages in the advertising process where bias can occur. From campaign selection and real-time bidding to ad selection, the dataset helps researchers understand and mitigate biases at each stage. It also introduces an unbiased utility metric to evaluate different bias mitigation techniques, ensuring that fairness improvements do not come at the cost of utility.
Unveiling Biases in Online Advertising
Experimental evaluations using the FairJob dataset show potential improvements in fairness through various bias mitigation techniques. These experiments underscore the trade-offs between fairness and utility, highlighting the need for context-dependent strategies. The dataset also emphasizes the importance of understanding and addressing selection bias and market bias, which can influence the fairness of AI systems. In the advertising ecosystem, ad-tech companies strive to deliver the most relevant advertisements to users by leveraging vast amounts of data on user behavior and preferences. However, if this data is biased, the algorithms can inadvertently perpetuate and amplify these biases. This issue is particularly acute in high-risk areas such as job advertising, where biased recommendations can exacerbate existing inequalities. For instance, if managerial positions are disproportionately shown to men over women, more men may apply, perpetuating historical biases and worsening gender disparities.
Challenges and Opportunities in Bias Mitigation
Bias can be introduced at several stages in the advertising process. When a user visits a webpage with an ad slot, ad-tech companies participate in a real-time bidding auction to select the most relevant ad. During this auction, companies consider various user and publisher attributes, including past interactions and context. Ensuring fairness in this auction process is crucial to prevent reinforcing existing inequalities. Once an ad-tech company wins the auction, it must decide which product to show, a decision that can also introduce bias. FairJob provides a comprehensive framework for understanding and mitigating these biases, ensuring that AI systems provide fair recommendations. The dataset includes pseudonymized user context and publisher features, collected during a five-month job targeting campaign. It contains over a million rows, each representing a product shown to a user. The dataset features a variety of user and product attributes, including a gender proxy derived from user interactions with gendered products. This proxy serves as a crucial element for fairness analysis, despite the inherent challenges of approximating sensitive attributes.
Towards Ethical AI Practices
FairJob is available under the CC-BY-NC-SA 4.0 license, allowing for sharing and adaptation while respecting attribution, non-commercial use, and share-alike conditions. It serves as a baseline for improving deep learning models on tabular data and exploring privacy-preserving techniques. The dataset's detailed description and feature statistics provide a comprehensive resource for researchers aiming to develop fair and robust AI models in the advertising domain. Addressing bias in AI systems requires proactive measures beyond legal compliance. The FairJob dataset represents a significant step towards this goal, enabling researchers to explore different AI and fairness algorithms. By providing a realistic and challenging benchmark, the dataset fosters inclusivity and ethical integrity in AI systems. It highlights the importance of understanding and addressing selection bias and market bias, which can influence the fairness of AI systems. Experimental evaluations using the FairJob dataset demonstrate the potential for improving fairness without sacrificing utility, underscoring the need for context-dependent strategies. Ultimately, FairJob offers a valuable resource for advancing algorithmic fairness in real-world scenarios, promoting ethical AI practices in job recommendation systems.
- FIRST PUBLISHED IN:
- Devdiscourse News Desk
ALSO READ
ID8NXT Presents a Nationwide Hybrid Hackathon in Collaboration with Public Sector Bank Bank of Baroda, Focused on Generative Artificial Intelligence
Tech Summit on Digital Marketing with AI in Punjab Reveals 82 Percent Spike in Artificial Intelligence Job Openings
AI Model Predicts Alzheimer's Onset with Speech Analysis
NVIDIA's Sewak Advocates for Culturally Diverse AI Models for India