Generative AI's Impact on 6G Networks: Optimizing Resource Use and Energy Efficiency
Researchers propose using Generative AI (GenAI) for demand-planning in 6G wireless networks to optimize resource utilization, enhance energy efficiency, and improve performance in scenarios such as cell-switching, user association, load balancing, and disaster response. Despite its transformative potential, challenges include hardware limitations, cybersecurity risks, information loss, and ethical concerns.
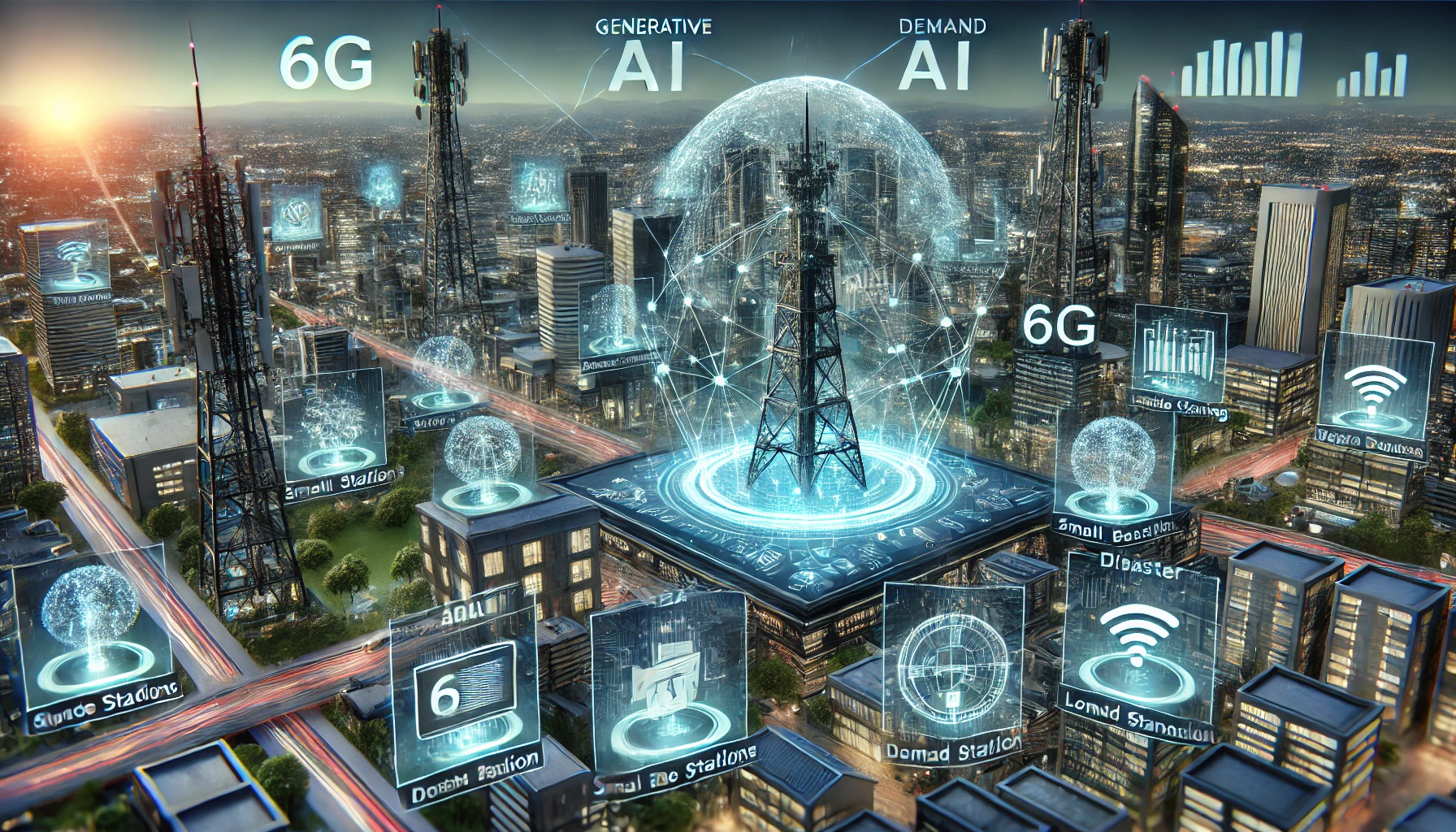
Researchers from Ankara Yıldırım Beyazıt University and Carleton University are exploring the use of Generative AI (GenAI) to revolutionize demand-planning in wireless communications, a development critical for the upcoming sixth-generation (6G) mobile networks. These networks promise hyper-reliable, low-latency communication, supporting applications like extended reality, holographic communication, and tactile internet applications that demand unprecedented performance levels. The study emphasizes the synergy between AI and wireless communications, with a focus on how GenAI can manage user demand more effectively than traditional AI, which primarily handles predictions, classifications, and optimization.
The Power of Demand-Planning
The proposed demand-planning involves three main processes: demand-labeling, demand-shaping, and demand-rescheduling. Demand-labeling categorizes user data based on priority. Demand-shaping compresses or converts data formats to fit within available network resources, and demand-rescheduling shifts non-critical data transmissions to times of lower network usage. These methods aim to balance network load, reduce energy consumption, and enhance overall network efficiency.
Real-World Applications of GenAI
Several scenarios illustrate the application of GenAI in demand-planning. In cell-switching, idle or lightly loaded small base stations (SBS) offload their traffic to macro base stations (MBS) and enter sleep mode, saving energy. By compressing user data, GenAI can increase MBS capacity, allowing more SBSs to be switched off and reducing overall network energy consumption. This approach is especially beneficial in dense networks, including non-terrestrial networks (NTN) like High Altitude Platform Stations (HIBS), which can host offloaded users and further enhance energy savings.
User association and load balancing present another challenge. In vertical heterogeneous networks (VHetNets), where various network tiers collaborate, efficient user association schemes are crucial to balance the load and optimize performance. GenAI can compress user data to reduce demand on wireless backhaul links, preventing congestion and enabling more efficient resource allocation. It can also be implemented at user terminals to compress data, requiring less transmit power and addressing uplink communication challenges in scenarios with significant free-space path loss.
Interference management is another critical area. Ultra-dense VHetNets operating in a harmonized spectrum often face performance issues due to propagated interference. By shaping user data and managing traffic more effectively, GenAI can improve interference management and spectral efficiency. In a network with one HIBS and four MBSs serving 50 users, data compression through GenAI allows for more efficient resource allocation, reducing interference and increasing spectral efficiency.
Enhancing Disaster Response with AI
In disaster scenarios, reliable and fast communication channels are essential for search and rescue (SAR) operations. However, telecommunication infrastructures are often compromised, limiting reliable links. GenAI can enhance communication efficiency by categorizing user-generated content based on its importance to the SAR mission, shaping critical data to require fewer resources, and rescheduling non-critical data. This approach alleviates the load on communication networks and ensures that critical data is transmitted promptly, facilitating effective disaster response.
Despite its potential, implementing GenAI-based demand-planning poses several challenges. Hardware limitations at user terminals, cybersecurity risks, potential information loss, and ethical concerns are significant hurdles. User terminals may struggle with the high computation and processing demands of GenAI, leading to increased energy consumption and heat generation. Ensuring data and GenAI model security is crucial to prevent malicious interventions and protect user privacy. There is also a risk of losing contextual information during the demand-shaping process, which could result in incomplete information for the receiver. Ethical concerns include the consistency and reliability of GenAI outputs, data privacy, transparency, and accountability.
Overcoming Challenges for a Connected Future
Hardware limitations are particularly challenging, as user terminals may not have the processing power to handle GenAI’s demands without significant energy consumption and heat generation. This impacts both the current and long-term performance of devices. Cybersecurity is another critical area, as GenAI models require robust systems to store and process large datasets, and there is a risk of malicious interventions during the demand-shaping process. Information loss is a concern because oversimplification of data can result in the omission of important details and nuances, leading to incomplete information for the receiver. Ethical issues, such as the consistency and reliability of GenAI outputs, data privacy, transparency, and accountability, also need to be addressed to ensure the technology's viability.
Overall, the study highlights the transformative potential of GenAI in demand-planning for wireless networks. By optimizing resource utilization and energy consumption, GenAI can make future ultra-dense wireless networks more sustainable, dynamic, and resilient, paving the way for seamless integration of non-terrestrial and terrestrial networks in the 6G era. This innovative approach could revolutionize how we manage network traffic, making wireless communications more efficient and reliable in an increasingly connected world.
- FIRST PUBLISHED IN:
- Devdiscourse