From GPT-4 to 6G: How LLMs are Shaping the Future of Telecommunications
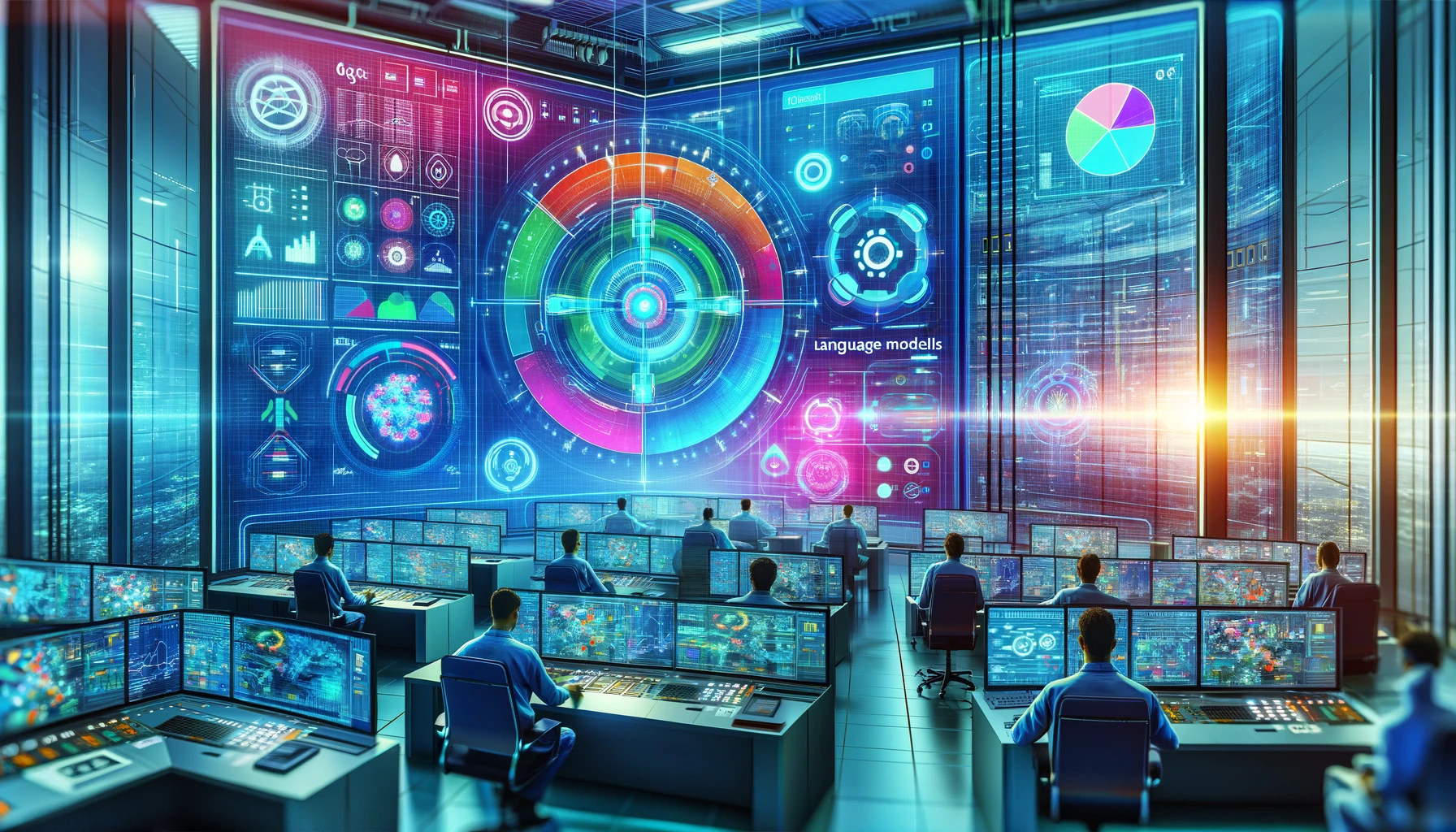
In the rapidly evolving world of telecommunications, large language models (LLMs) are emerging as transformative tools. These advanced models, known for their exceptional comprehension and reasoning capabilities, are set to revolutionize the telecom industry, paving the way for 6G networks and beyond. Researchers from McGill University, Samsung Research America, Western University, and Simon Fraser University are leading the charge in exploring the potential of these models in the telecom sector.
A New Era in Telecommunications
LLMs, like OpenAI's GPT-4, have already made significant strides in various fields such as healthcare, law, and finance. Now, their potential in telecommunications is being explored with promising results. These models, after undergoing pre-training and fine-tuning, can perform a diverse array of tasks based on human instructions, moving us closer to the dream of artificial general intelligence (AGI)-enabled 6G networks.
The principles of LLMs are rooted in their architecture and training processes. These models are built using transformers, a type of neural network architecture that enables them to understand and generate human-like text. The training involves exposing these models to vast amounts of data, allowing them to learn patterns and structures in language. Fine-tuning these pre-trained models on specific telecom data can further enhance their capabilities, making them adept at handling domain-specific tasks.
Key Applications in Telecom
The versatility of LLMs opens up numerous applications in the telecom sector. One of the primary areas is the generation of telecom domain knowledge. LLMs can create comprehensive summaries, overviews, and interpretations of telecom standards, technologies, and research findings. This capability democratizes access to advanced telecom knowledge, making it accessible to a broader audience, including researchers, practitioners, and even the general public.
For instance, LLMs can be used for question-answering tasks in the telecom domain. These models can answer complex queries about telecom technologies, standards, and best practices, providing accurate and detailed explanations. Studies have shown that fine-tuning LLMs on telecom-specific datasets significantly improves their performance in generating relevant and accurate responses.
Another exciting application is in troubleshooting telecom networks. Telecom networks are complex systems, and identifying and resolving faults can be time-consuming and require significant expertise. LLMs can automate this process by analyzing previous trouble reports and generating recommended solutions for new issues. This automation not only speeds up the troubleshooting process but also reduces the workload on human experts.
Enhancing Code and Network Configurations
Code generation is another area where LLMs are making a significant impact. Telecom networks rely heavily on efficient and reliable code for their operations. LLMs can generate code for various telecom applications, refactor existing code, and even validate the correctness of the code. This capability can drastically reduce the time and effort required for coding tasks in telecom, improving overall efficiency.
LLMs also excel in generating network configurations. Telecom networks require precise configurations to ensure optimal performance and security. LLMs can take natural language requirements and translate them into formal specifications and device configurations. This automation reduces the chances of human error and ensures that network configurations are consistently accurate and up-to-date.
Optimization and Prediction
Optimization is a critical aspect of telecom networks, and LLMs offer new opportunities in this area. These models can automate the design of reward functions for reinforcement learning, a type of machine learning used for optimizing network performance. By automating this process, LLMs can enhance the efficiency of reinforcement learning applications in telecom.
Prediction is another area where LLMs show great promise. Telecom networks generate vast amounts of data, and predicting future trends and behaviors is crucial for maintaining optimal performance. LLMs can be used for time-series prediction and multi-modality problems, enabling telecom operators to anticipate network demands and potential issues before they arise.
Challenges and Future Directions
Despite the immense potential of LLMs in telecommunications, there are several challenges that need to be addressed. One of the main challenges is training LLMs with telecom-specific data. This process requires extensive datasets and significant computational resources. Fine-tuning general-domain LLMs for specific telecom tasks is a more efficient approach but still requires careful dataset collection and preprocessing.
Practical deployment of LLMs in telecom networks also presents challenges. Telecom tasks often have stringent requirements for delay and reliability, and LLM models must be optimized for efficiency and rapid response times. Additionally, telecom devices typically have limited computational and storage resources, necessitating efficient model training, fine-tuning, and inference techniques.
Despite these challenges, the future of LLMs in telecommunications looks bright. These models have the potential to automate and optimize a wide range of tasks, from generating domain knowledge and troubleshooting solutions to optimizing network performance and predicting future trends. As research and development in this area continue, LLMs are poised to become indispensable tools in the telecom industry, driving the evolution of next-generation networks.
- FIRST PUBLISHED IN:
- Devdiscourse