Wildfire Management: Integrating Human-Autonomous Teaming for Enhanced Surveillance and Suppression
Stanford researchers have developed a hierarchical framework integrating human-autonomous teaming to optimize wildfire surveillance and suppression, significantly improving containment effectiveness. This innovative approach leverages drones for real-time data and manned aircraft for suppression, ensuring efficient and safe wildfire management.
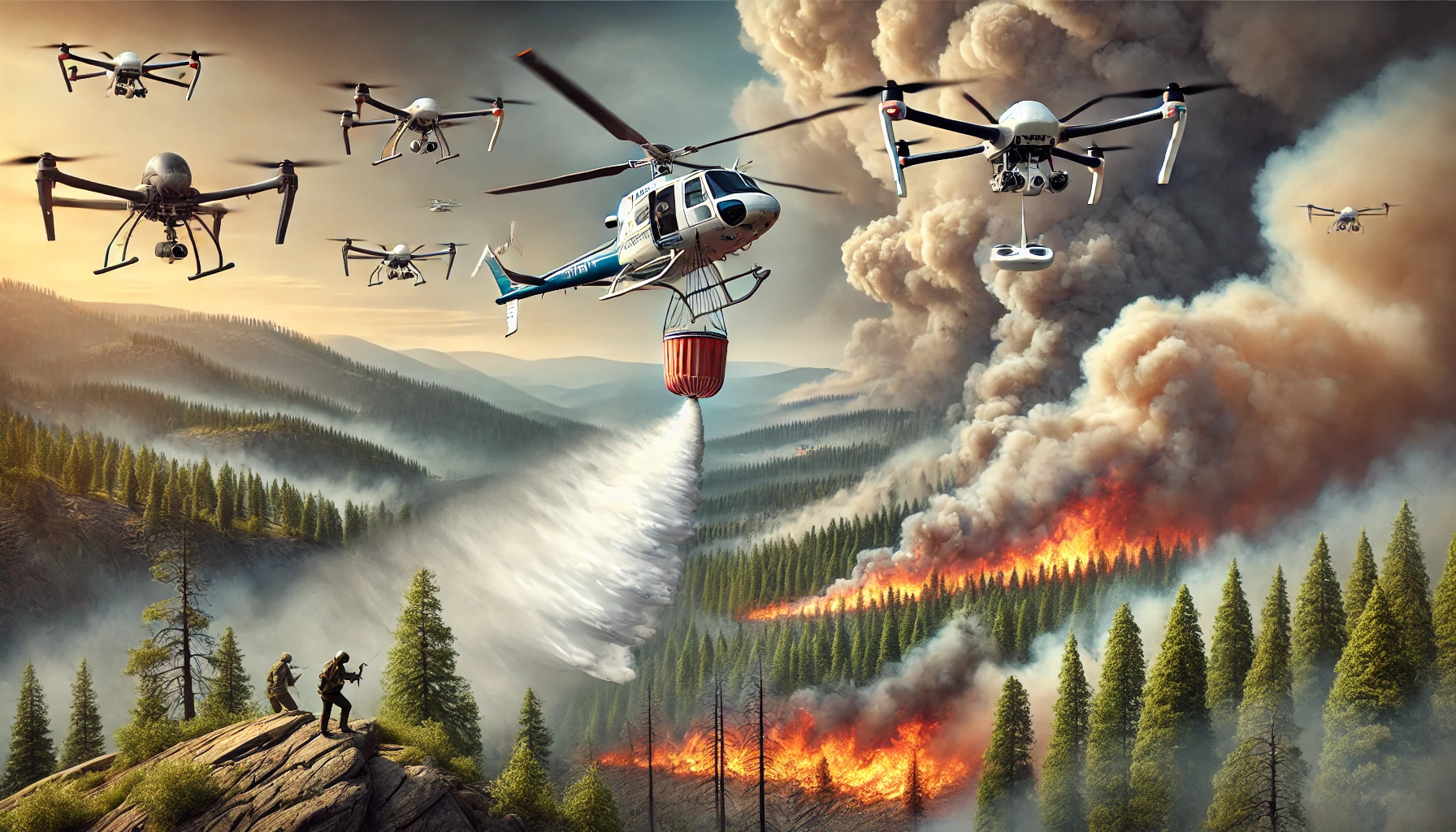
Researchers from the Stanford Intelligent Systems Laboratory, have developed an innovative hierarchical framework aimed at enhancing wildfire surveillance and suppression through the integration of human-autonomous teaming. This new approach seeks to optimize the initial attack phase of wildfire management, a crucial stage that significantly influences the outcome of containment efforts. The initial attack involves the deployment of the first set of resources to respond to a wildfire, and its success largely depends on the effectiveness and coordination of these resources. Traditional methods have faced significant challenges due to the complexity and vast action spaces required to optimize both surveillance and suppression simultaneously.
Revolutionary Approach to Wildfire Management
The framework devised by the researchers divides the initial attack process into two interconnected components: surveillance and suppression. Each of these components operates on different time scales, allowing for more specialized and efficient planning and execution. Unmanned aircraft, or drones, are employed for surveillance, while manned aircraft, such as helicopters, perform suppression activities. This division not only enhances the operational efficiency but also allows the drones to provide real-time updates on wildfire progression, thereby informing and optimizing the suppression strategies of the manned aircraft.
Ensuring Safety and Efficiency with Advanced Technology
A key feature of this framework is its robust collision avoidance system, which ensures the safety and efficiency of operations by preventing potential collisions between manned and unmanned aircraft. By utilizing a multi-agent partially observable Markov decision process (MPOMDP), the researchers have managed to address the substantial computational challenges associated with optimizing such complex tasks. The hierarchical structure of the framework allows for asynchronous actions, meaning that surveillance drones can operate continuously, gathering and transmitting valuable data that informs the suppression efforts conducted by the manned aircraft.
Proven Effectiveness Through Simulation
The framework's effectiveness was demonstrated through simulations, where it significantly outperformed traditional firefighting techniques. For moderate wildfires, the new approach showed improvements of up to 242%, and for rapid wildfires, it achieved enhancements of 60%. These simulations included abstracted case studies as well as a real-world scenario based on the Makaha Valley fire in Hawaii, thereby underscoring the practical applicability and robustness of the framework. The use of Monte Carlo tree search (MCTS) and various domain-specific extensions has further augmented the decision-making capabilities of the system, ensuring that both surveillance and suppression actions are optimized in real-time.
Early Dispatch and Reward Models Enhance Response
In developing the suppression planner, the researchers explored different reward models, including localized and global resource destruction minimization. These models play a critical role in prioritizing suppression efforts based on the potential destruction of valuable resources, thereby making firefighting efforts more effective and efficient. The localized destruction minimization model focuses on the immediate area surrounding the suppression activity, allowing for a more precise and impactful response. Conversely, the global destruction minimization model considers the broader area, ensuring that suppression activities have a widespread beneficial impact.
Additionally, the framework incorporates early dispatching of additional suppression assets, a feature validated through regression models. These models predict the wildfire's progression and determine the optimal timing for deploying additional resources. This timely reinforcement significantly enhances containment efforts, preventing wildfires from escalating beyond control. The hierarchical framework's adaptability to various environmental conditions and its ability to integrate seamlessly with existing firefighting protocols make it a versatile and powerful tool in wildfire management.
Significant Advancement in Wildfire Response
The integration of human-autonomous teaming in wildfire management marks a significant advancement in the field, offering a scalable and adaptable solution to the escalating threat of wildfires. By leveraging the complementary strengths of manned and unmanned aircraft, this framework provides a robust and flexible approach to optimizing wildfire surveillance and suppression, with the potential to save lives and reduce economic losses. The hierarchical framework's ability to operate under different environmental conditions and its effectiveness in real-time decision-making underscore its potential as a critical tool in the future of wildfire management. The research highlights the importance of continued innovation and the adoption of advanced technologies in tackling the growing challenges posed by wildfires, ultimately aiming to enhance the resilience and effectiveness of wildfire response efforts.
- FIRST PUBLISHED IN:
- Devdiscourse
ALSO READ
Latvia to Send €112 Million Military Aid and Thousands of Drones to Ukraine
Wildfires Ravage Sao Paulo State: Two Dead, Cities on Alert
Tropical Storm Hone Threatens Hawaii with Floods, Strong Winds, and Wildfire Risks
Deadly Wildfires Ravage Southern Sao Paulo State in Brazil
Inferno in Sao Paulo: Wildfires Devastate Sugarcane Fields and Communities