Advanced Water Table Predictions: A New Tool for Forest Hydrology and Wetland Management
Researchers developed a predictive model using BigVAR to estimate daily water table depth based on hydroclimatic variables, improving forest and wetland management. The model proved accurate and adaptable, even without certain data like streamflow, making it a valuable tool for sustainable resource management.
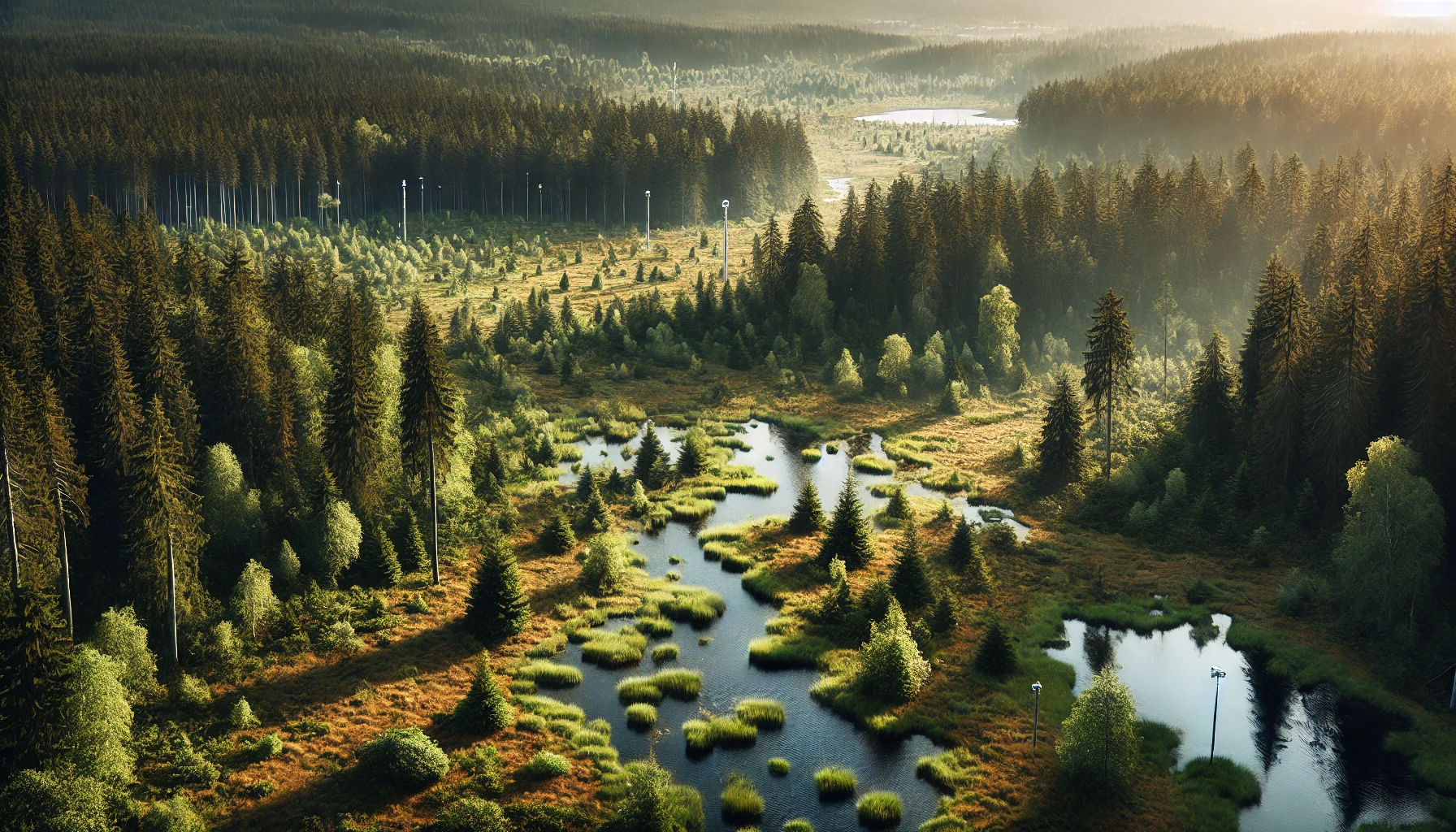
Researchers from the University of Connecticut, South Dakota State University, and the USDA Forest Service conducted a study focused on developing a predictive model for estimating daily water table depth in forested ecosystems, specifically wetland areas. They utilized BigVAR, a recently developed statistical model capable of handling high-dimensional multivariate time series data. The aim was to create a reliable and interpretable tool for predicting water table depth based on various hydroclimatic variables, such as soil temperature, precipitation, wind direction, and solar radiation, to improve decision-making in forest management and wetland hydrology. The model was developed using data from four experimental watersheds located in South Carolina (WS77, WS78, and WS80) and North Carolina (D1). These regions feature distinct hydrological characteristics, with the South Carolina sites situated on a low-gradient coastal plain and the North Carolina site on an artificially drained plantation. The data spanned from 2006 to 2019 in South Carolina and 1988 to 2008 in North Carolina.
Autoregressive Modeling for Improved Accuracy
The study employed an autoregressive modeling approach, which takes into account lag structures in the variables to better capture the complex time-dependent relationships influencing water table depth. The model incorporated daily weather and hydrological data, such as rainfall, wind speed, relative humidity, and solar radiation, to predict water table depth. The inclusion of lagged variables allowed the model to account for the delayed effects of these environmental factors. For instance, the water table depth from the previous four days, as well as solar and net radiation from the prior two days, were found to be significant predictors. This approach helped improve the accuracy of the model, especially in fluctuating hydrological conditions. During the testing phase, the model’s performance was evaluated using root mean squared error (RMSE) and the coefficient of determination (R²), both of which indicated strong predictive capability. For instance, at the WS80 site in South Carolina, the model achieved an R² of 0.93 during a dry year (2019) and 0.96 during a wet year (2016), demonstrating its robustness across different environmental conditions.
Key Findings on Rainfall and Water Table Dynamics
One of the key findings of the study was the importance of rainfall and its lagged values in predicting water table depth, particularly during the growing and dormant seasons. While rainfall significantly influenced the water table depth, the inclusion of other variables like solar radiation and wind direction also contributed to the model’s predictive power. Interestingly, daily streamflow data, which is often considered a key predictor in hydrological models, was found to have a negligible impact on water table depth during the growing season. This result suggests that other factors, such as precipitation and the lagged effects of rainfall, play a more prominent role in controlling water table dynamics in these forested ecosystems.
Variable Selection and Its Impact on Model Performance
The study also explored the process of selecting the most relevant variables for the model, highlighting the varying degrees of influence that different environmental factors had across the seasons. For example, wind direction was more influential during certain seasons, while net radiation played a greater role in others. This variable selection process not only improved the model’s predictive performance but also enhanced its interpretability, allowing researchers and forest managers to better understand the drivers of water table fluctuations.
Robustness of the Model Without Streamflow Data
In addition to testing the model with all available data, the researchers explored its robustness by removing daily streamflow as a variable. The model’s performance remained relatively stable even without streamflow data, which is often difficult to obtain in certain regions. The mean squared error (MSE) increased only slightly in some cases, indicating that the model can still provide accurate predictions even when certain variables are unavailable. This flexibility makes the model particularly useful for forest managers and hydrologists working in regions where comprehensive data collection may be challenging.
Future Directions for Water Table Depth Prediction
The study underscores the importance of accurately predicting water table depth in forested ecosystems, particularly in wetland areas where water management is critical for maintaining ecological balance and supporting forest productivity. The BigVAR model offers a practical and interpretable solution for this challenge, with its ability to incorporate lagged hydroclimatic variables and perform variable selection, making it a valuable tool for silvicultural management. The researchers recommend further exploration of more complex spatiotemporal models that can account for broader regional variations, as well as the development of methods to address missing data in future studies. Overall, the model demonstrated strong potential for improving the understanding and management of water table dynamics, offering insights that could aid in the sustainable management of forest resources.
- READ MORE ON:
- BigVAR
- USDA
- water table depth
- hydroclimatic
- FIRST PUBLISHED IN:
- Devdiscourse