Optimizing Soil Properties: Leveraging SMAP Satellite Data for Better Agricultural Outcomes
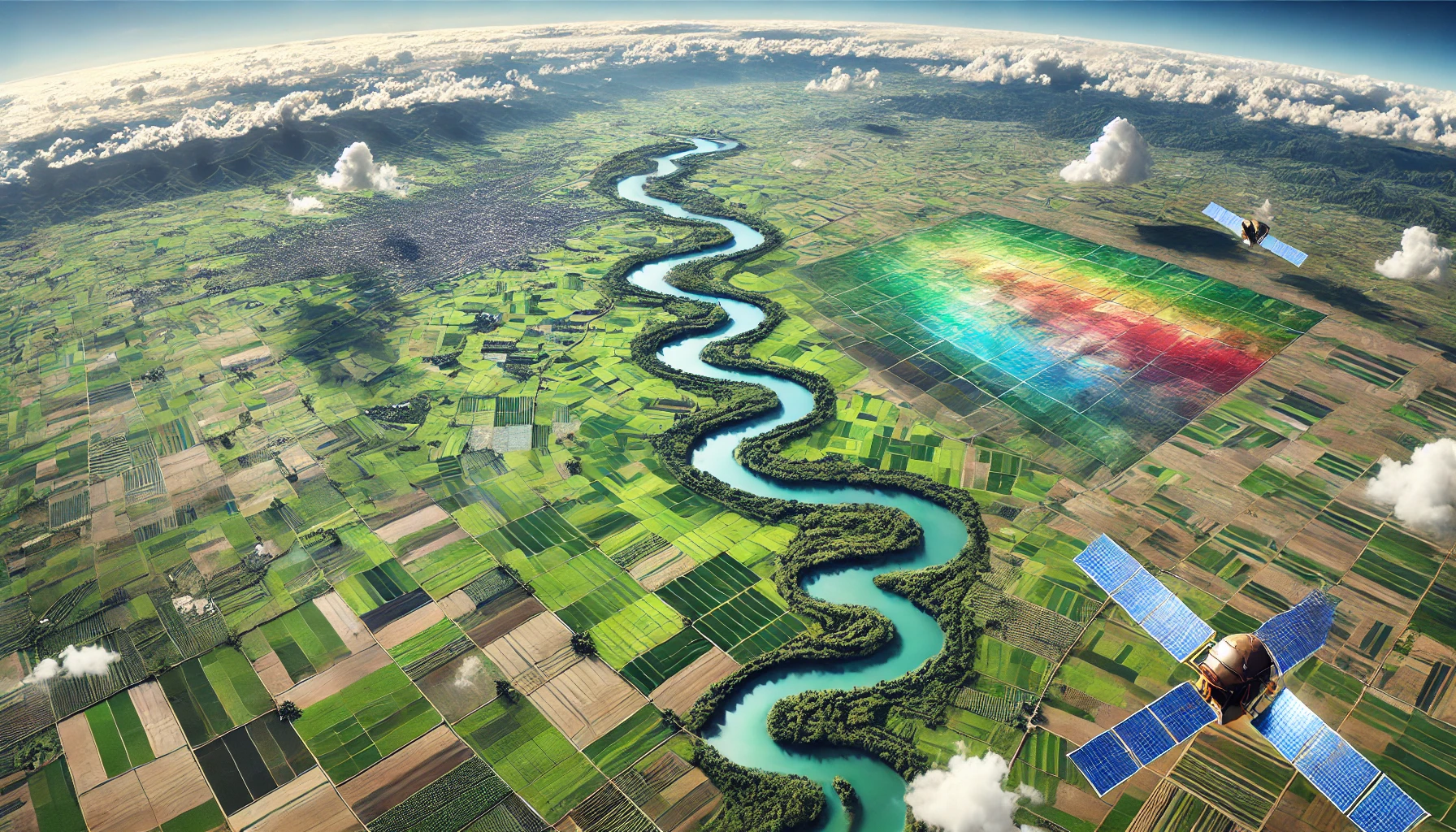
A new study introduces an innovative framework for optimizing soil properties to enhance water resource management in agriculture, using data from NASA's Soil Moisture Active Passive (SMAP) satellite. Accurate estimation of soil properties is critical for managing water resources, especially in agriculture-dominant regions like the Lower Mekong River (LMR) basin. The research from Michigan State University, NASA Goddard Space Flight Center, University of Massachusetts, and Asian Disaster Preparedness Center, Bangkok, focuses on optimizing three key soil properties: saturated hydraulic conductivity (Ksat), exponent parameter in Campbell’s equation for hydraulic conductivity (expt), and bulk density (bd). Using a modified Shuffled Complex Evolution (SCE-UA) algorithm with six years of SMAP data, the framework aims to improve the performance of the Variable Infiltration Capacity (VIC) hydrological model within the Regional Hydrological Extremes Assessment System (RHEAS).
Significant Gains in Simulation Accuracy
The researchers evaluated the impact of these optimized soil properties on streamflow simulation and agricultural drought severity. They tested four configurations: an open-loop setup, one optimized with SMAP soil moisture data (SMAP), another with actual streamflow data (Runoff), and a final one combining both datasets (SMAP_Runoff). The results were promising. Switching from the initial setup to the SMAP-optimized model increased the Nash-Sutcliffe Efficiency (NSE) by 56.4%, and upgrading from the streamflow-optimized to the combined data model raised the NSE by 21.9%. This demonstrated the significant benefits of optimizing soil properties for more accurate simulations.
Realistic Drought Representation
Moreover, the optimized model accurately represented the severity and extent of historical agricultural droughts, aligning closely with regional drought reports of the LMR basin. The study emphasized the crucial role of soil moisture variability, influenced by physical properties such as texture, structure, porosity, bulk density, surface and subsurface crusting, and organic matter content, in managing agricultural water. It also highlighted the need for accurate soil hydraulic properties, including hydraulic conductivity, water retention, soil water holding capacity, and infiltration rate, for effective water management and irrigation scheduling.
Critical Region for Rice Cultivation
The significance of the study area is underscored by the LMR basin's status as a major rice cultivation region in Southeast Asia, supporting over 300 million individuals. The research aims to optimize soil properties at a spatial resolution of 0.05 degrees to facilitate efficient agricultural water resource management. The study utilized data from various sources, including atmospheric forcing from the Climate Hazards Group InfraRed Precipitation with Station data (CHIRPS), soil and vegetation parameters from the Moderate Resolution Imaging Spectroradiometer (MODIS), and streamflow data from the Mekong River Commission (MRC) Data Portal to drive the VIC model within the RHEAS framework.
Enhanced Monitoring and Management
A sensitivity analysis identified three key soil parameters (expt, Ksat, bd) as highly sensitive for soil moisture simulations. The optimized soil parameters demonstrated significant spatial heterogeneity, reflecting the natural variability of soil properties. Streamflow simulations using these optimized parameters showed substantial improvements, especially in smaller, less regulated watersheds like Phienluang, compared to larger watersheds with extensive anthropogenic interventions like Lumphat.
Agricultural drought monitoring using the Soil Moisture Deficit Index (SMDI) indicated that the optimized model better captured the severity of drought conditions. The study demonstrated that integrating remote sensing data with hydrological models enhances the ability to monitor and manage agricultural drought, providing valuable insights for water resource management in agriculture-intensive and data-scarce regions.
The optimized soil properties significantly improved the spatial heterogeneity of soil parameters and demonstrated enhanced performance in simulating streamflow and monitoring agricultural drought in the LMR basin. This framework holds promise for applications in data-scarce, ungauged regions and agriculture-intensive watersheds, offering valuable insights for estimating irrigation water usage and managing agricultural reservoirs. Post-calibration, the model effectively depicted the actual extent and severity of drought events during reported drought years, aligning with MRC reports. The northeastern part of Thailand, substantial portions of Cambodia, and certain areas in Laos were identified as highly susceptible to agricultural drought. Croplands experienced the most significant benefits from model calibration, emphasizing the potential of this framework for crop water management.
The study highlights the effectiveness of integrating satellite-based soil moisture observations to optimize soil physical and hydraulic properties. This integration provides valuable tools for enhancing hydrological modeling and drought management in complex and variable environments. Ultimately, these improvements contribute to more effective agricultural water resource management and planning, underscoring the broader potential applications of this framework in similar regions to enhance food security and irrigation decision-making.
- READ MORE ON:
- SMAP Satellite Data
- Soil Moisture Active Passive
- NASA
- Asian Disaster Preparedness Center
- Shuffled Complex Evolution
- SMAP
- Shuffled Complex Evolution (SCE-UA)
- RHEAS
- Climate Hazards Group InfraRed Precipitation with Station
- CHIRPS
- Mekong River Commission
- RHEAS framework
- Agricultural drought
- Soil Moisture Deficit Index (SMDI)
- Soil Moisture Deficit Index
- LMR basin
- FIRST PUBLISHED IN:
- Devdiscourse