Revolutionizing Gene Therapy: AI-Powered Protein Design Breakthrough
In a significant stride for gene therapy, scientists have utilized artificial intelligence (AI) to craft novel protein variants, aiming to outsmart the immune system's resistance and enhance treatment efficacy. Published in Nature Machine Intelligence, this pioneering research introduces ProteinVAE, an AI framework adept at generating complex proteins, promising a new era of efficient, AI-driven medical solutions with broad applications in disease treatment.
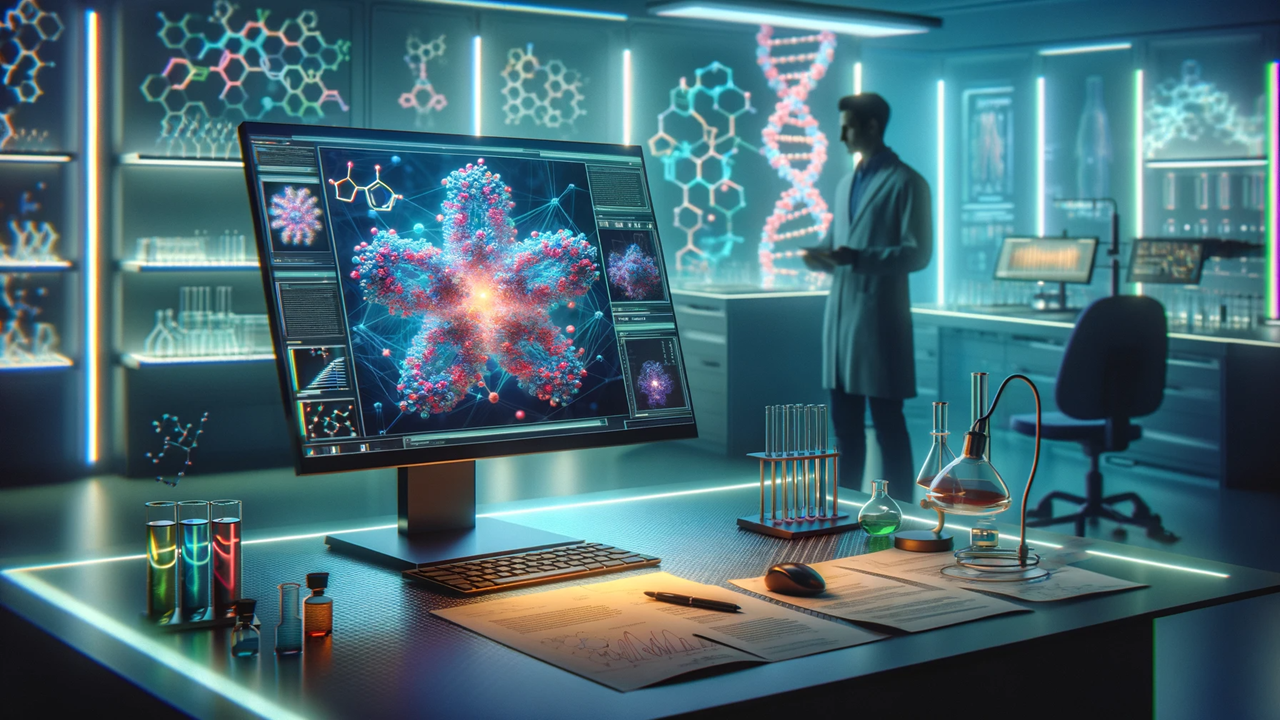
A collaborative team of scientists is leveraging artificial intelligence (AI) to transform the realm of gene therapy, a medical strategy that leverages genes to combat or prevent illnesses. Published in Nature Machine Intelligence, their pioneering research is centered on reengineering a pivotal protein to amplify the success of gene therapy while curtailing its adverse effects.
Michael Garton, a distinguished assistant professor at the University of Toronto, underscored the immense potential harbored by gene therapy. Yet, he also acknowledged a significant impediment: the body's immune system frequently mounts a defense against the viral vectors integral to gene therapy, thereby impeding its effectiveness. The team's investigative efforts are particularly directed at hexons, the core proteins in adenovirus vectors crucial for gene therapy, which are impeded by these immune defenses.
The immune system's response, especially due to specific antibodies, can thwart the therapy, culminating in suboptimal outcomes and pronounced side effects. To counter this, Garton's laboratory harnessed AI to engineer novel hexon protein variants that markedly differ from their natural counterparts, rendering them invisible to the immune system. Suyue Lyu, a Ph.D. candidate and the principal author of the study, is dedicated to crafting proteins so distinct that they elude immune detection.
Conventionally, the creation of new proteins is a tedious and expensive endeavor, fraught with extensive experimentation. However, the introduction of an AI-mediated methodology by the researchers heralds a more streamlined approach. It facilitates a wider spectrum of variations, slashes expenses, and accelerates the process by enabling swift simulations and pinpointing specific protein variants for empirical examination.
While a plethora of protein-designing frameworks is available, devising new variants, particularly for sizable proteins like hexons (averaging 983 amino acids), poses a formidable challenge. The team introduced an innovative AI framework, ProteinVAE, adept at deciphering the intricate structure of lengthy proteins with minimal data. ProteinVAE distinguishes itself through its generative prowess, rivaling that of bulkier models despite its more compact structure.
Lyu accentuated the model's proficiency, particularly its deployment of pre-trained protein language models for rapid assimilation of small data sets. The model is also intricately tailored for the generation of lengthy proteins. Contrasting with other more sizeable models that demand extensive computational resources, ProteinVAE delivers swift training and inference on conventional GPUs, enhancing its accessibility for a broader spectrum of research laboratories.
The AI model has demonstrated its potential in simulations, showcasing its capacity to modify a substantial portion of the protein's surface, potentially eluding immune detection. Garton is sanguine about the wider applications of this AI model, positing that it could be instrumental in protein design for various disease therapies. He envisages a future where generative AI can forge new biological entities with therapeutic significance for groundbreaking medical interventions.