Strengthening academic integrity by detecting and managing AI content in education
AI-generated content poses a unique challenge in academic environments, where originality and intellectual honesty are paramount. Educators are grappling with the increasing prevalence of students using AI tools to complete assignments, which undermines the development of essential writing and analytical skills.
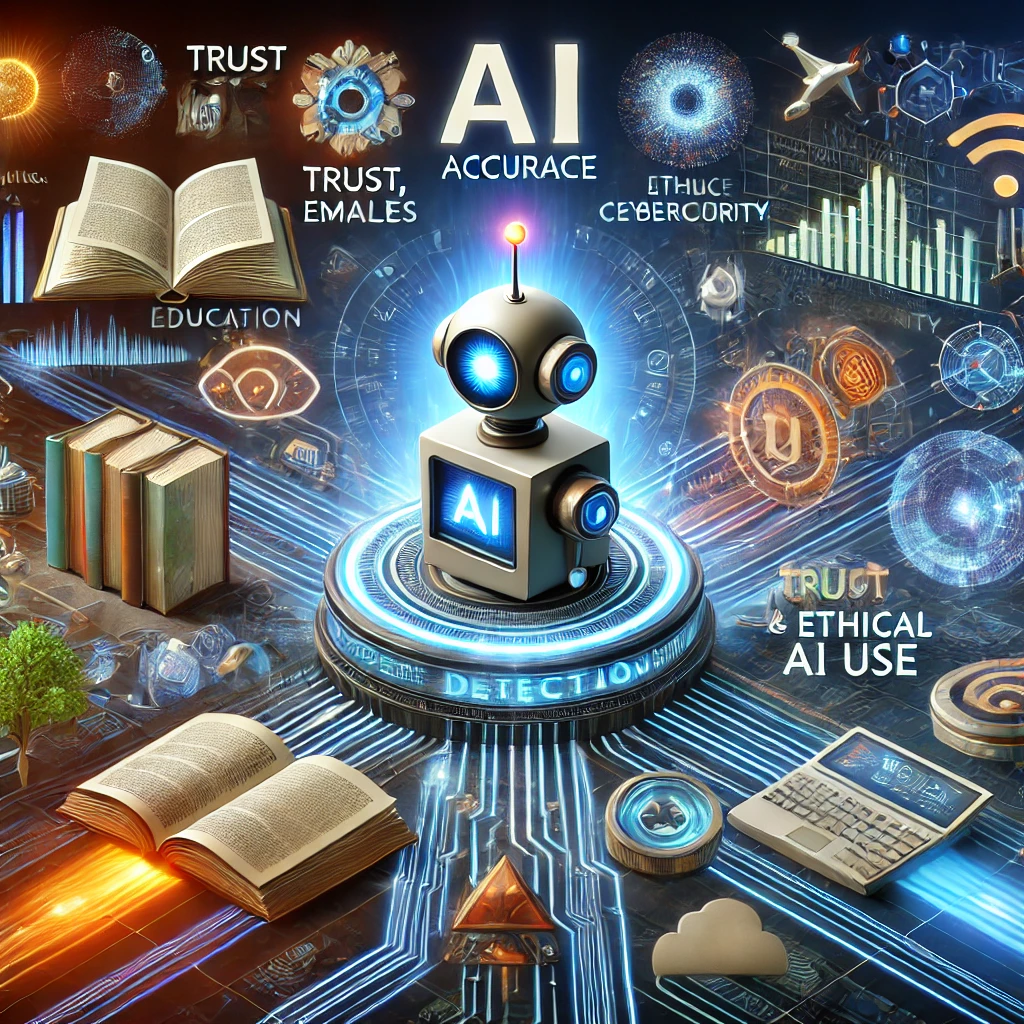
The rise of generative AI tools like ChatGPT has transformed how content is created, raising critical questions about authenticity, originality, and academic integrity. A recent paper titled "Detecting AI-Generated Text in Educational Content: Leveraging Machine Learning and Explainable AI for Academic Integrity," authored by Ayat A. Najjar, Huthaifa I. Ashqar, Omar A. Darwish, and Eman Hammad, explores innovative solutions to address these challenges. Submitted on arXiv, the paper provides valuable insights into the development of machine learning (ML) and deep learning (DL) models to differentiate between human-written and AI-generated text, specifically in cybersecurity education.
The need for AI-Generated text detection
Generative AI models like ChatGPT have revolutionized industries, including education, by providing powerful tools for content creation. However, their growing adoption also raises concerns about plagiarism, loss of critical thinking, and the authenticity of student work. These challenges underscore the urgent need for tools that can accurately distinguish between human-authored and AI-generated text.
AI-generated content poses a unique challenge in academic environments, where originality and intellectual honesty are paramount. Educators are grappling with the increasing prevalence of students using AI tools to complete assignments, which undermines the development of essential writing and analytical skills. This study addresses these issues by proposing a robust framework to detect AI-generated content, helping maintain ethical standards in education while supporting the responsible integration of digital technologies
The study’s key contributions
The research focuses on developing and testing a machine-learning-based framework to identify AI-generated cybersecurity content. Key contributions include:
-
CyberHumanAI Dataset: The study introduced a novel dataset comprising 1,000 cybersecurity paragraphs—500 written by humans and 500 generated by ChatGPT. This dataset served as the foundation for training and evaluating the proposed models.
-
Performance of Machine Learning Models: Traditional ML algorithms such as Random Forest (RF) and XGBoost performed exceptionally well, achieving classification accuracies of 81% and 83%, respectively. These results highlight the strength of ML models in handling small, task-specific datasets, often outperforming deep learning models in these scenarios.
-
Challenges in Short-Content Detection: Detecting AI-generated content in short texts, such as paragraphs, presented a significant challenge due to limited context and overlapping linguistic patterns. This emphasizes the need for models optimized for shorter content analysis.
-
Explainable AI (XAI): By incorporating Local Interpretable Model-Agnostic Explanations (LIME), the study enhanced model transparency. The analysis revealed distinct linguistic differences, with human-written text favoring action-oriented words like "use" and "allow," while AI-generated text exhibited a more formal and generalized tone, using words such as "realm" and "employ."
-
Comparison with GPTZero: The study's tailored models outperformed GPTZero, a commercial AI detection tool, achieving a 77.5% accuracy rate compared to GPTZero's 48.5%. This underscores the importance of domain-specific models in improving detection precision.
Methodology and insights
The study employed a comprehensive workflow, including data preprocessing, feature selection using Term Frequency-Inverse Document Frequency (TF-IDF), and training on a combination of ML and DL models. The results showed that classical ML models, such as XGBoost and Random Forest, excel in distinguishing AI-generated content, especially when supported by explainable AI techniques.
The findings revealed fascinating insights into linguistic patterns. Human authors are more likely to use straightforward and action-oriented terms, while AI-generated content exhibits a structured and generalized tone. These differences not only assist in content classification but also provide a deeper understanding of how AI models like ChatGPT craft responses
Applications beyond education
The implications of this research extend far beyond academia, with significant potential to impact multiple domains. In the field of digital forensics, the ability to detect AI-generated content can bolster cybersecurity measures by identifying phishing emails and fake reports crafted using AI, thereby protecting individuals and organizations from malicious activities.
For content moderation, social media platforms and news outlets can employ these models to flag AI-generated misinformation, enhancing the reliability and credibility of online information in an era of growing digital manipulation.
In business operations, organizations leveraging AI for automated content creation can implement these tools to ensure the accuracy and accountability of AI-generated material, reducing risks associated with misleading or incorrect outputs. These applications demonstrate how the research findings can contribute to fostering trust, security, and accountability across various industries.
Challenges and Future Directions
The study highlights several challenges that must be addressed to improve detection systems. These include the difficulty of detecting AI-generated short texts, the need for scalable models capable of handling large datasets, and the necessity of developing robust solutions for paraphrased or hybrid content. Additionally, expanding datasets to include diverse domains, languages, and writing styles will be critical for enhancing model generalizability.
Ethical considerations also play a vital role. The researchers advocate for tools that not only detect AI-generated content but also educate users about responsible AI usage. By incorporating explainable AI, they aim to foster transparency and trust, ensuring these systems are both effective and accountable.
As AI continues to shape the way we communicate and learn, tools like those proposed in this research will play a crucial role in ensuring that these technologies are used responsibly and transparently.
- FIRST PUBLISHED IN:
- Devdiscourse